Tracking neurons’ long projections to unravel autism
Many brain imaging studies of autism focus on neurons’ connections or activity, but examining neuronal tracts across the brain is equally informative, say Julia Owen and Pratik Mukherjee.
One way to imagine the brain is as a computer with a large number of processing units, each specialized for a particular task. In this analogy, the brain’s gray matter — which consists of neurons’ cell bodies and their points of contact with other neurons — represents the processors. White matter, which is made up of neurons’ long projections, or axons, represents the wires that connect the network.
Many brain imaging techniques focus on features of gray matter. Magnetic resonance imaging (MRI) measures gray matter’s thickness and surface area, for example. And functional MRI (fMRI) looks at changes in blood oxygenation to regions of gray matter, which researchers interpret as brain activity.
However, it is equally important to assess the properties of white matter. These studies can tell researchers how strong the connections are between brain regions and whether the neuronal tracts that support these connections show signs of injury or abnormal development.
When making conclusions about white matter, researchers should remember that most measures of white matter structure tend to be indirect. The most common method to assess the structural properties of white matter is diffusion-weighted MRI (dwMRI). This method looks at the direction of water diffusion in the brain to understand the orientation and structure of white matter tracts.
Each measured pixel of a dwMRI image contains on the order of 50,000 axons. The ability to noninvasively scan individual axons — which are about 0.001 millimeters in diameter — is not yet possible. Butwe can extrapolate features of white matter structure from dwMRI measurements. Typically, we do this by looking at fractional anisotropy (FA), a measure of the directionality of water diffusion.
Water ways:
Water tends to flow more easily along tracts of white matter than if it goes perpendicular to the axons. This is because in the latter scenario, it may bang up against other structural elements such as the axon membranes and layers of insulation, called myelin. Along the direction of the axons, water faces no such barriers.
For example, highly parallel axons have high FA values, whereas less parallel axons have low FA (see inset). However, it’s not yet possible to distinguish the different scenarios that could give rise to altered FA measurements, including the presence of crossing axons, rather than parallel or dense ones.
Although we cannot directly relate FA to distinct features of white matter, FA measures are nevertheless a useful indicator of white matter’s structure. For instance, FA increases up to age 30 and then starts decreasing after 401. Studies have shown that people with low FA measurements struggle with cognitive tasks such as navigating a maze2 and have low intelligence quotients (IQ)3.
FA can be a sensitive biomarker for conditions ranging from traumatic brain injury to autism4, 5. However, studies have not found any consistent abnormalities in FA in people with autism6. This may be because of the heterogeneity of the disorder: Autism results from many different originating causes and individuals with the disorder can have deficits in many different aspects, including social, emotional, language, sensory and motor skills.
To add to the complexity, FA has been found to be higher or lower in people with autism than in controls, depending on the study.
For example, many studies show low FA levels in people with autism, but someteams have reported elevated FA in children with autism younger than 4 years of age7, 8, 9 and in adolescents with the disorder10. Researchers have described this same disparity in results from fMRI studies of functional connectivity in people with autism.
Cataloging the features of individuals who have the same genetic lesion may lessen some of the heterogeneity. For example, FA is thought to be elevated in people with a deletion of the autism-linked 16p11.2 chromosomal region12 and low in those with a duplication of the region11, 12.
In either case, the affected regions of white matter are widespread across the brain and do not localize to areas with specialized functions. It is fascinating, and perhaps a bit perplexing, that variation in the same chromosomal region can lead to either an increase or a decrease in FA. This suggests that significant deviations from normal can lead to neurological impairments, no matter the direction: The stronger the deviation, the worse the impairment.
For example, in a study published in April, we found an association between higher axial diffusivity (a metric that correlates with highFA) and low IQ in people with the 16p11.2 deletion12.
As we mentioned above, FA is only an indirect measure of white matter and does not directly predict changes in neurons. To address this shortcoming, researchers are developing biophysical models of white matter that require advanced MRI hardware and techniques. For example, a technique called neurite orientation dispersion and density imaging quantifies the density of the axons and how parallel the white matter is13.
Using this method, we have found that people with a 16p11.2 deletion have lower measures of axon density than controls do, whereas those with a duplication show the opposite trend.
This result suggests a biophysical explanation for the opposing changes in FA seen in people with deletion or duplication of 16p11.2. Researchers can adapt clinical MRIs to include advanced measures of white matter. This would allow them to better relate changes in white matter to properties of the underlying neurons.
In the grand scheme of things, most of what we know about the brain comes from studying individual neurons or up to hundreds of them. Connecting brain imaging measurements to this wealth of cellular neuroscience knowledge will enable better understanding of disorders such as autism.
Pratik Mukherjee is professor of radiology and bioengineering at the University of California, San Francisco. Julia Owen is a postdoctoral scholar in his laboratory.
References:
1:Kochunov P. et al. Neuroimage 58, 41-49 (2011) PubMed
2: Grieve S.M. et al. AJNR Am. J. Neuroradiol. 28, 226-235 (2007) PubMed
3: Schmithorst V.J. et al. Hum. Brain Mapp. 26, 139-147 (2005) PubMed
4: Niogi S.N. and P. Mukherjee J. Head Trauma Rehabil. 25, 241-255 (2010) PubMed
5: Nucifora P.G. et al. Radiology 245, 367-384 (2007) PubMed
6: Travers B.G. et al. Autism Res. 5, 289-313 (2012) PubMed
7: Ben Bashat D. et al. Neuroimage 37, 40-47 (2007) PubMed
8: Weinstein M. et al. Hum. Brain Mapp. 32, 534-543 (2011) PubMed
9: Wolff J.J. et al. Am. J. Psychiatry 169, 589-600 (2012) PubMed
10: Cheng Y. et al. Neuroimage 50, 873-882 (2010) PubMed
11: Stefansson H. et al. Nature 505, 361-366 (2014) PubMed
12: Owen J.P. et al. J. Neurosci. 34, 6214–6223 (2014) PubMed
13: Zhang H. et al. Neuroimage 61, 1000-1016 (2012) PubMed
Recommended reading
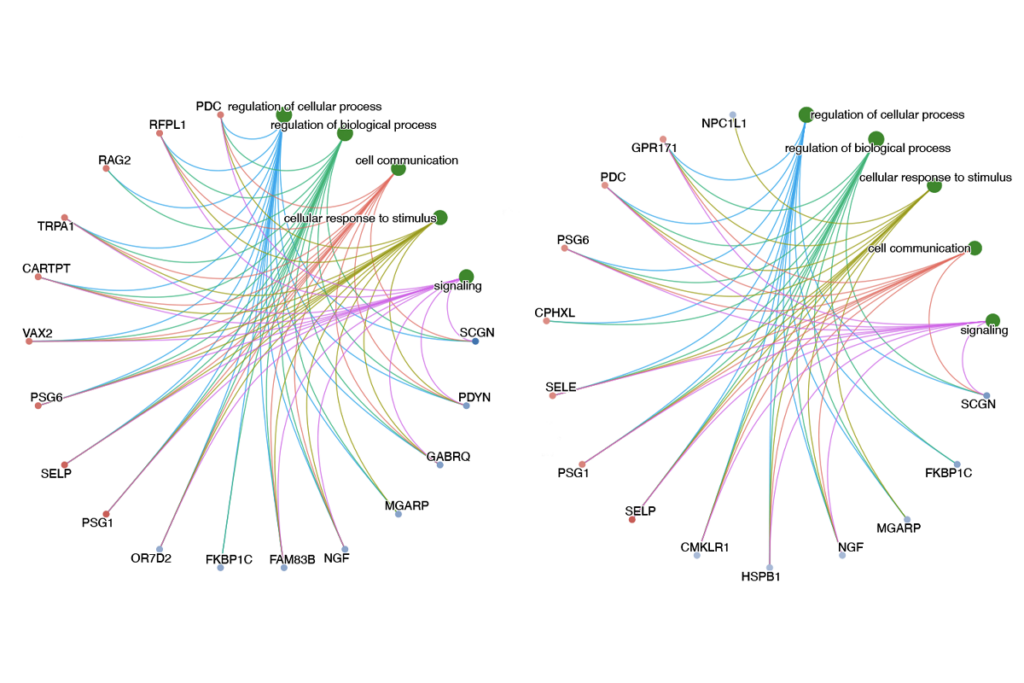
New tool may help untangle downstream effects of autism-linked genes
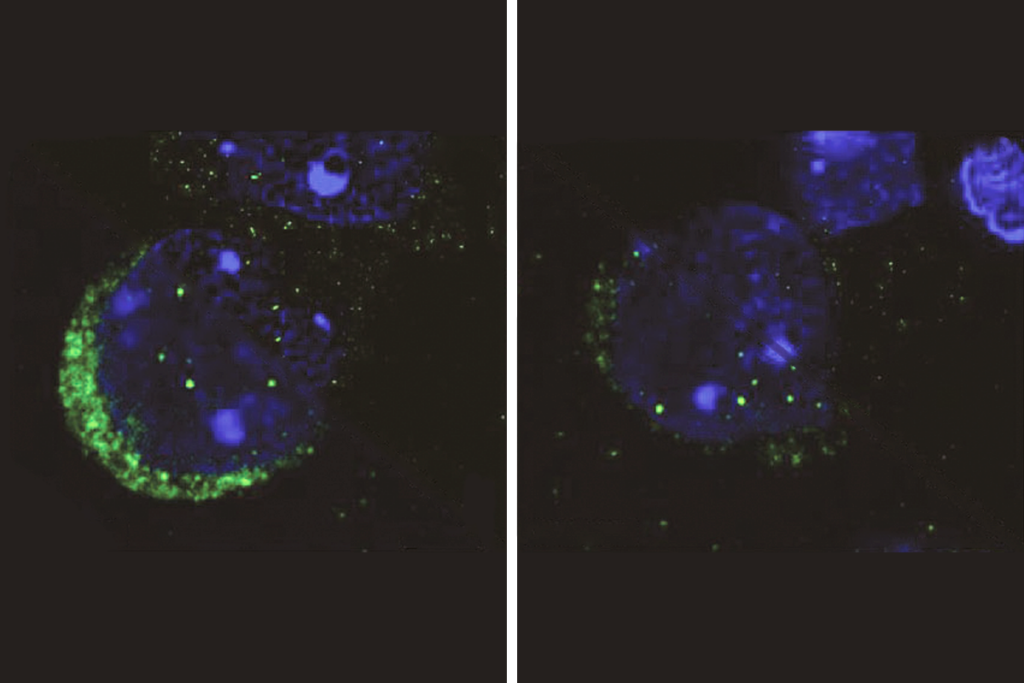
NIH neurodevelopmental assessment system now available as iPad app
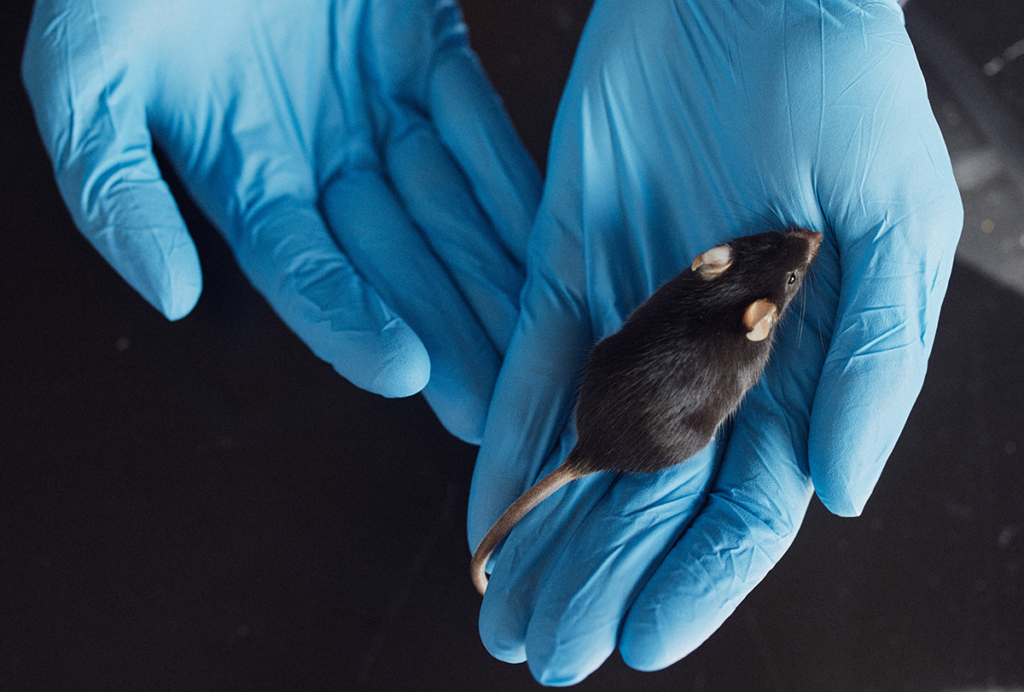
Molecular changes after MECP2 loss may drive Rett syndrome traits
Explore more from The Transmitter
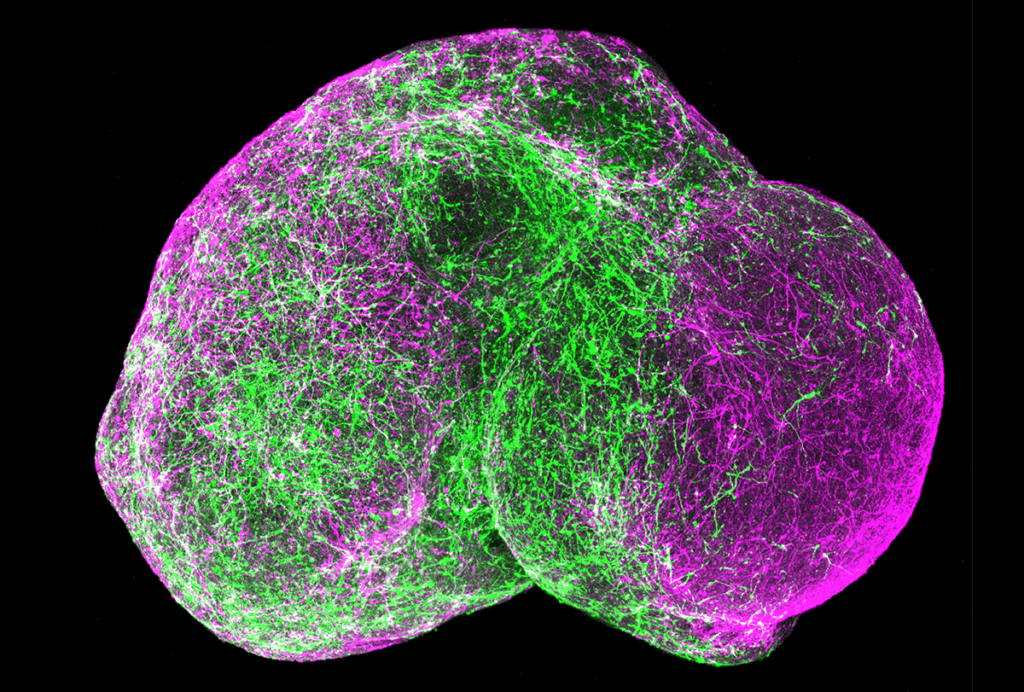
Organoids and assembloids offer a new window into human brain
Who funds your basic neuroscience research? Help The Transmitter compile a list of funding sources
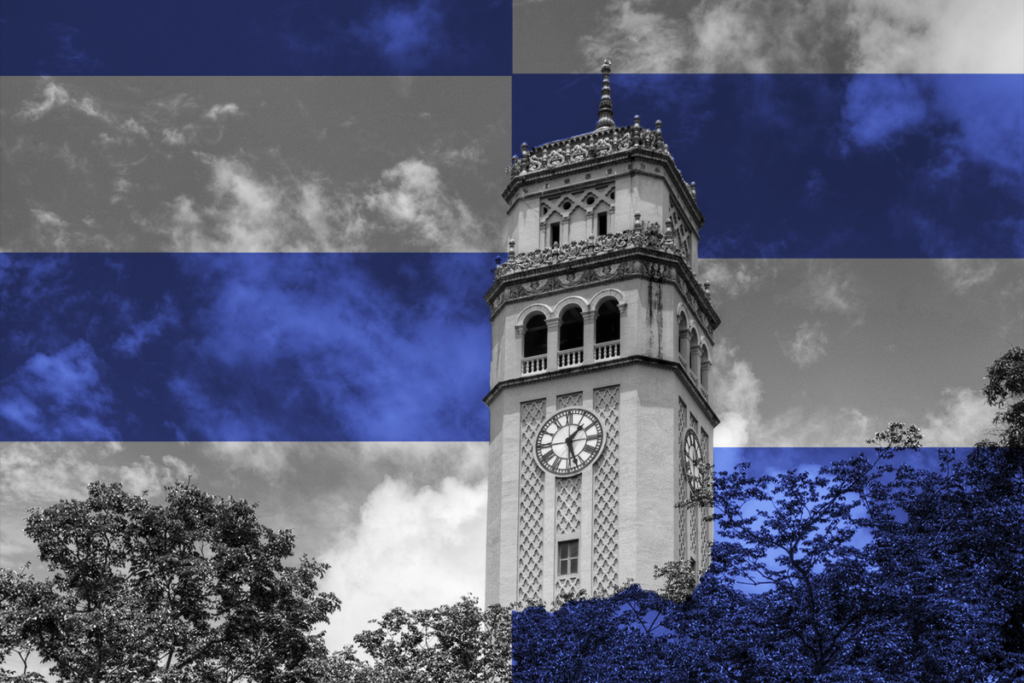