For studies, size matters: Let us count the ways
In autism research, as in other fields, small sample sizes can lead to false findings. The size of the sample needed for statistical significance depends on the type of study.
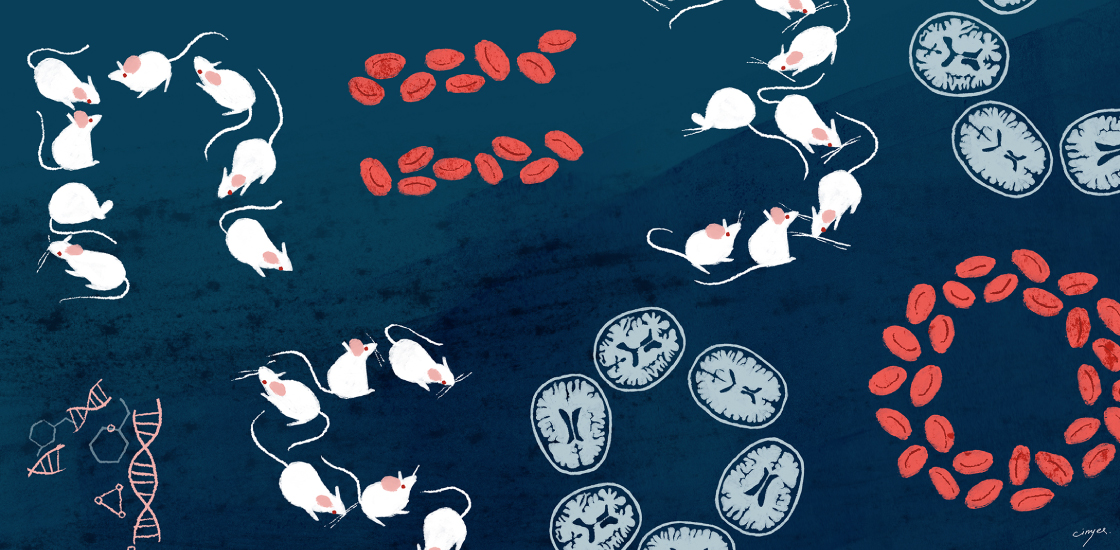
In autism research, as in other fields, a small sample size can lead to false findings.
Insufficient sample size can fail to distinguish signal from noise, confounding attempts to determine which patterns are real and which are merely an artifact.
Most studies of treatments for people with autism include fewer than 100 participants, and some dip well below 60. Although the ideal number varies with the question under study, these numbers are too small to generalize to larger populations of autistic individuals in most cases, experts say. And the ramifications of trusting underpowered studies can be serious.
“People have assumed there are treatment effects for interventions on the basis of trials that are too small and badly designed,” says Jonathan Green, professor of child and adolescent psychiatry at the University of Manchester in the United Kingdom. “The good news is we’ve wised up to it.”
But how big is big enough? That depends on what researchers are studying, and why.
Here are the ideal sample sizes for different types of studies:
Clinical research:
Behavioral or drug treatments should include enough participants to represent all of the autism subtypes for which that treatment might be used.
But some fundamental problems in the field complicate this effort. Clinicians still lack reliable methods for diagnosing autism, in part due to the diverse ways it manifests. That difficulty leads to little agreement on how to identify subtypes.
As a rough rule of thumb, researchers say that studies of treatments should include at least 100 people. The actual number needed to find a valid effect depends on a range of factors, including the magnitude and frequency of the effect in the general population.
Brain imaging studies:
Many imaging studies historically included 20 or fewer participants. In the past 10 years, however, researchers have been aiming for studies with closer to 100 participants. Studies that aim to trace developmental trajectories should also track the same few individuals over time, scanning their brains at regular intervals, rather than examining a cross-section of people of different ages.
Some studies are including scans from hundreds of participants. For example, an open-access repository of brain images called the Autism Brain Imaging Data Exchange (ABIDE) includes anonymized scans from more than 1,000 people with autism and more than 1,000 controls. However, these scans come from different sites, and the differences in methods used at those sites can affect the results.
Genetic studies:
A large number of genes influence autism, each making a small contribution. So scientists who are looking for rare autism-related mutations by sequencing protein-coding genes must rely on large numbers of sequences to identify statistically significant effects — typically on the order of thousands of people for the rarest variants.
The studies must be even larger when analyzing whole genomes, which include sequences that don’t code for proteins, to find risk variants. That’s because scientists run millions of statistical tests to measure the likelihood that each variant from each person in the study is associated with the trait of interest. The use of large numbers of statistical tests increases the chance of false-positive results, so the studies need tens of thousands of individuals to counterbalance the influence of random fluctuations.
Even larger sample sizes — hundreds of thousands of individuals — are necessary for genome-wide association studies, in which researchers compare the DNA of people with and without a condition using thousands of markers scattered throughout their genomes. Using this design, scientists can identify common gene variants that contribute to the risk of a condition.
Preclinical research:
Biomedical researchers have performed underpowered animal studies for decades, in part due to the cost and ethical issues of working with large numbers of animals. In some cases, researchers try to make up for their low numbers by analyzing a large number of cells or other samples from each animal, which sometimes results in an error called ‘pseudoreplication.’
Researchers can control lab animals’ diets, ages and housing conditions, and scale doses or treatments by weight. Many researchers therefore considered sample sizes on the order of 10 animals to be acceptable. However, in autism research, a good rule of thumb is that studies should include a minimum of 15 animals per group to identify important biological effects.
In the past few years, statisticians and some funders have pushed for larger numbers in animal studies. Some autism researchers now voluntarily comply with guidelines for animal research set by a government agency in the United Kingdom in consultation with scientists; hundreds of journals and funders have endorsed the guidelines. One item asks researchers to show how they calculated the number of animals they needed to include in their study to ensure statistical rigor.
Biomarker studies:
Scientists continue to seek physiological characteristics, such as patterns of eye movements, brain waves or activity, or blood chemistry, that could help clinicians and researchers identify children with autism. These biomarkers may also distinguish subtypes of autism.
The identification of biomarkers would speed up trials, cut costs and enable earlier delivery of treatment. But candidate biomarkers have often failed in subsequent studies.
Researchers agree that biomarker studies in autism usually include too few individuals to yield meaningful findings. To show clinical promise, these studies must draw samples from at least 100 individuals. And clinical trials of biomarkers designed to flag people with autism, or a particular form of autism, should ideally include at least 1,000 participants. Researchers should also replicate the efficacy of a biomarker in an independent sample.
Some scientists are designing biomarker studies of thousands of participants that combine data from behavioral, imaging and genetic studies.
Field trials:
Researchers are increasingly designing trials of autism and autism treatments in real-world settings. Such studies involve variables that are hard to control, and so must include hundreds of individuals to yield meaningful results.
Educational psychologist Connie Kasari at the University of California, Los Angeles, for instance, is testing combinations of behavioral interventions tailored to meet individual children’s needs. In her ‘adaptive trial,’ which includes 192 children with autism, all children received the same initial intervention and were grouped into different arms with various follow-up treatments based on how they responded to the initial treatment.
Strong study designs require more than an appropriate number of participants. Researchers should also include a representative mix of sexes and ages, because the condition presents in different ways in girls and boys, and features change as children develop.
Studies of treatments should also adhere to data-reporting standards, such as the CONSORT guidelines. These stipulate, for instance, that in randomized controlled clinical trials, researchers give detailed explanations of their methods — for instance, how participants were randomized to different trial arms. And results are more meaningful if researchers assess the efficacy of a treatment both immediately afterward and also months or years later.
Recommended reading
Assembloids illuminate circuit-level changes linked to autism, neurodevelopment
Explore more from The Transmitter
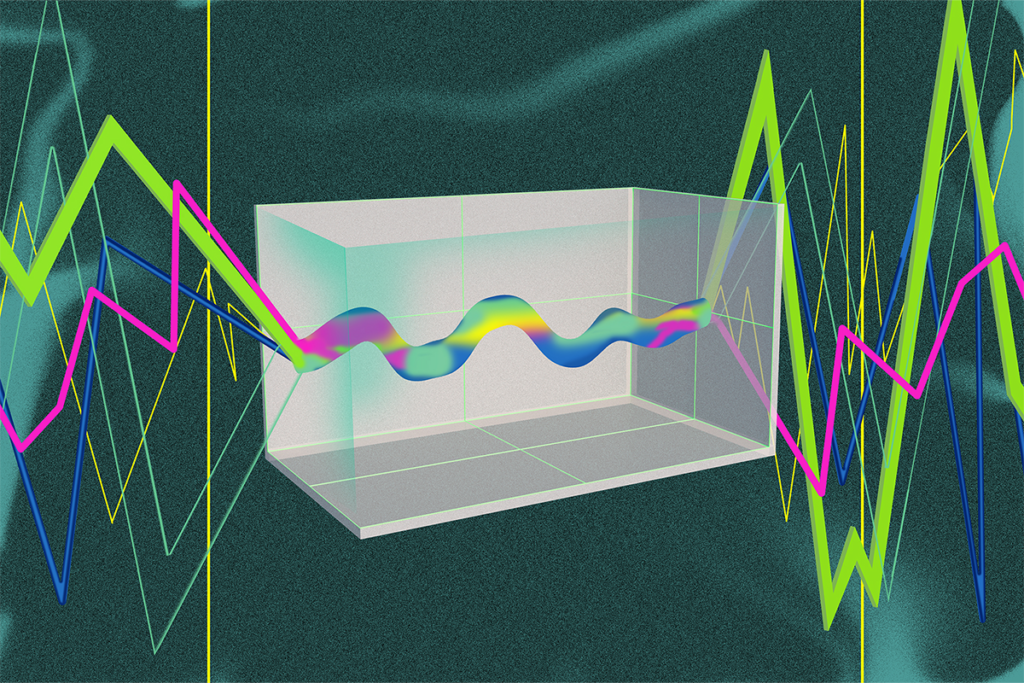
The Transmitter’s favorite essays and columns of 2024
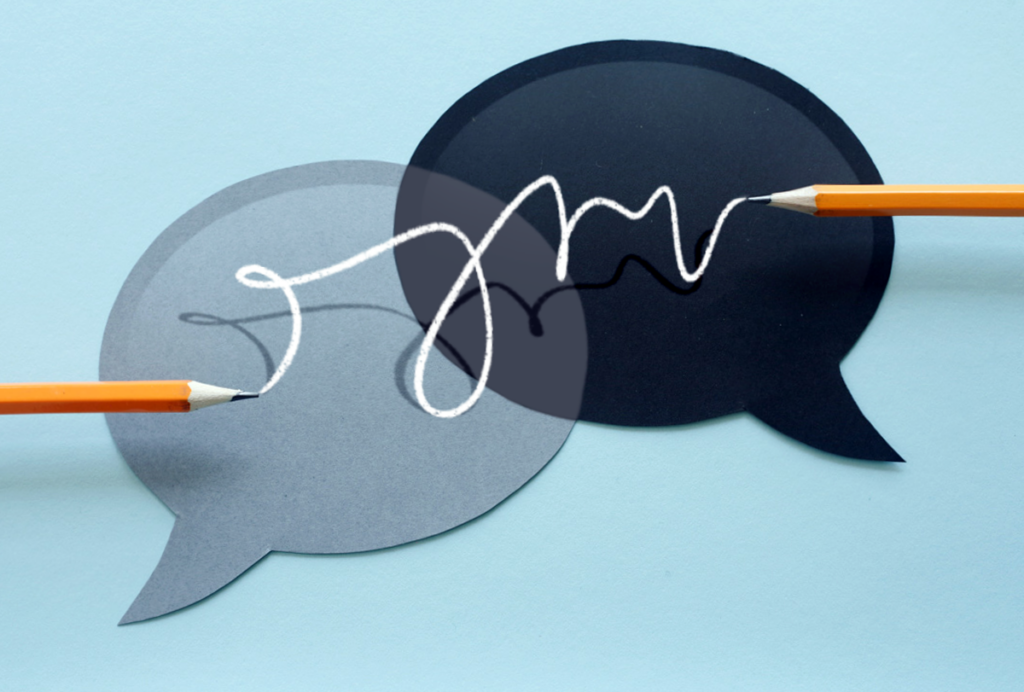
Say what? The Transmitter’s top quotes of 2024
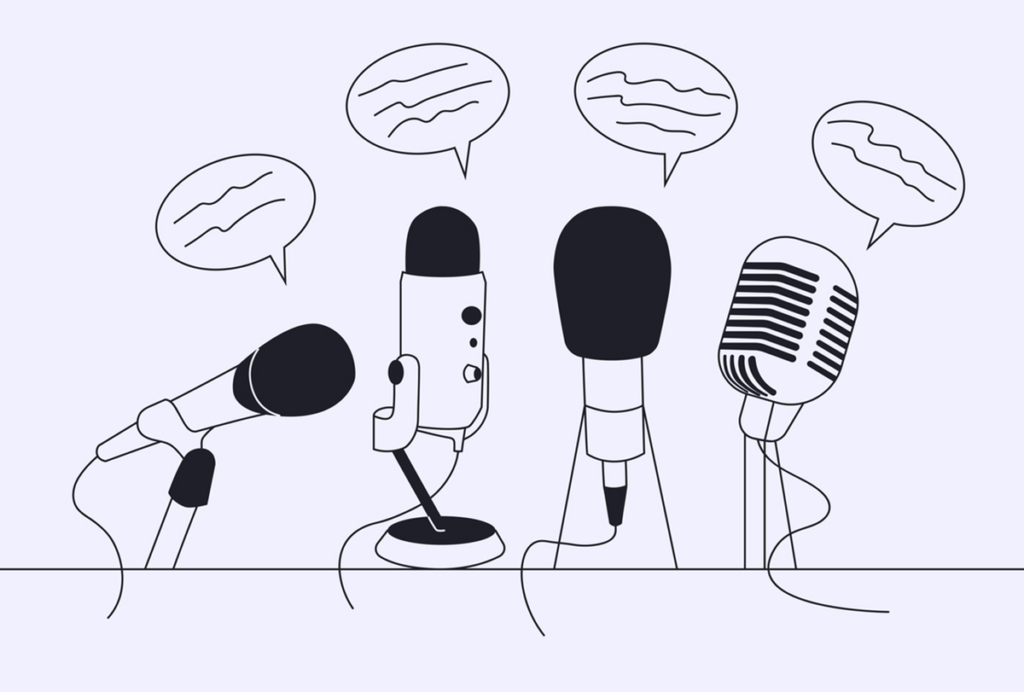