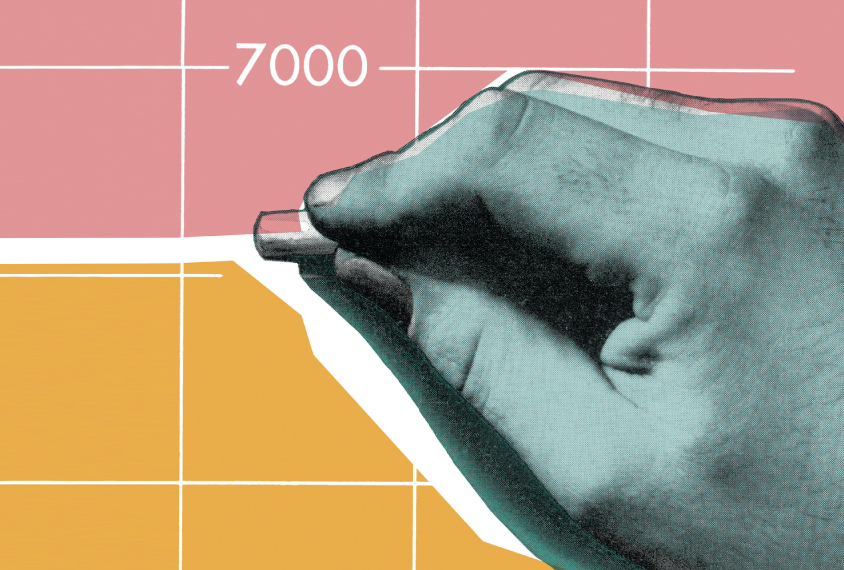
Statistics experts urge scientists to rethink the p-value
The American Statistical Association is asking researchers to revamp how they use common statistical methods.
In 2015, science journalist John Bohannon fooled countless people into believing chocolate helps with weight loss. But as he later revealed, Bohannon and his collaborators had deliberately set up the study to yield spurious correlations, which they marketed to reporters seeking splashy headlines.
Although the hoax was controversial, as it included real volunteers and spread disinformation to prove its point, it revealed several lessons on shoddy research practices. In particular, Bohannon’s team showed how easy it is to draw big claims from weak evidence. To do this, they tried to measure whether several factors — including weight, cholesterol, sleep quality, blood protein levels and more — change as a result of eating a chocolate bar every day. They studied only 15 people. But as Bohannon noted, one of science’s dirty secrets is that measuring many variables in a small number of participants makes it easier to find correlations that exist purely by chance.
Although Bohannon’s study was designed to deliberately surface findings that don’t exist, some scientists have been exploiting this loophole more subtly to pump out flashy findings. Now, the American Statistical Association (ASA) is looking to tackle the problem head-on, asking researchers to revamp how they use common statistical methods.
For decades, researchers have used a statistical measure called the p-value — a widely debated statistic that even scientists find difficult to define — that is often a requirement for publication in academic journals. In many fields, experimental results that yield a p-value of less than 0.05 (p<0.05) are typically labelled as ‘statistically significant.’ Lower p-values imply that a result is more likely to be real, instead of a statistical fluke.
Playing with data to meet the significance thresholds required for publication — known as p-hacking — is an actual thing in academia. In fact, for decades, it’s been mainstream practice, partly due to researchers’ lack of understanding of common statistical methods.
But in recent years, many academics have gone through a methodological awakening, taking a second look at their own work, in part due to heightened concern and attention over p-hacking. Perhaps the most high-profile recent case of mining and massaging of data was that of food scientist Brian Wansink, who eventually resigned from Cornell University after being found to have committed scientific misconduct.
Yet, Wansink’s main misdeed of torturing data until achieving statistically significant results has been common scholarly practice for years. “I think Wansink’s methods are emblematic of the way statistics is misused in practice,” Susan Wei, a biostatistician now at the University of Melbourne in Australia who sifted through years of Wansink’s emails, previously told Undark. “I lay the blame for that partially at the feet of the statistical community.”
In response to concerns, the ASA has released advice on how researchers should — and should not — use p-values, devoting an entire issue of its quarterly publication, The American Statistician, to the topic.
In 2016, the ASA, waking up to the scale of p-hacking that plagues scholarly research, took an unprecedented step: For the first time in its history, the society issued explicit guidelines on how to avoid misapplying p-values. Poor practice, the organization said, was casting doubt on the field of statistics more generally.
Since its release, the 2016 statement has been cited nearly 1,700 times and attracted almost 300,000 downloads. Still, the ASA knew there was more work to be done, as their 2016 recommendations told researchers only what they shouldn’t do but didn’t offer advice on what they should do. “We knew that was a shortcoming in the p-value statement,” says ASA executive director Ronald Wasserstein.
Moving beyond thresholds:
In 2017, the ASA organized a symposium on statistical methods, which led to inviting experts to submit papers to a special issue of The American Statistician. This was published on 21 March and consists of 43 papers and an editorial, all aimed at explaining to non-statisticians how to use p-values responsibly.
Specifically, the ASA is calling for researchers to stop using the term ‘statistical significance’ altogether, noting it was never meant to indicate importance. Instead, Wasserstein says, the term was popularized by British statistician Ronald Fisher in the 1920s to hint that something may warrant a further look. “What statistical significance was supposed to mean,” he says, is equivalent “to what a right swipe on Tinder is supposed to mean.”
The ASA isn’t the first to voice concerns over how p-values are used in practice. In 2015, one scholarly journal — Basic and Applied Social Psychology — went as far as banning p-values entirely. The reason is simple, says the journal’s executive editor, David Trafimow, a social psychologist at New Mexico State University: “I have never read a psychology paper where I felt p-values improved the quality of the article; but I have read many psychology papers where I felt p-values decreased the quality of the article.”
Even though the shortcomings of p-values have been known for decades, the last couple of years have seen heated debates about significance thresholds. In 2017, a group of 72 prominent researchers urged researchers to abandon p<0.05 as the gold standard and instead start using p<0.005 (some fields, such as particle physics and genomics, already require much lower p-values to support new findings). Doing so, they argued, would dramatically lower the number of effects reported to exist when they actually don’t — or false positives — in scholarly literature.
Later in 2017, a different batch of 88 academics hit back against the idea of lowering p-value thresholds, suggesting instead that researchers should be allowed to set their own thresholds as long as they justify them.
The ASA is suggesting a different approach. The organization wants to move academic research beyond significance thresholds, so that studies aren’t selectively published because of their statistical outcomes. According to the ASA, p-values shouldn’t be used in isolation to determine whether a result is real. “Setting loose the bonds of statistical significance lets science be science and lets statistics be statistics,” Wasserstein says.
Although the ASA thinks moving beyond thresholds will cause upheaval at first, it will be beneficial in the long term. “Accepting uncertainty … will prompt us to seek better measures, more sensitive designs, and larger samples,” Wasserstein and his colleagues write in the new editorial.
Researchers should report findings regardless of their outcomes, rather than cherry-picking results and publishing only positive findings, the ASA suggests. Wasserstein notes that scientists should also publish details about the methods they plan to use before conducting studies. This would solve the problem of ‘hypothesizing after the results are known,’ or HARKing, where researchers actively hunt for trends in already collected data, which seemed to be the case in the Wansink saga. (Psychology is already going through a reformation, in which preregistration — where research design, hypotheses and analysis plans are published beforehand — is catching on.)
John Ioannidis, who studies scientific robustness at Stanford University in California, says the ASA’s move is a step in the right direction and may result in more reproducible literature. But it won’t fix all of academia’s problems, he adds: “There are still major issues around transparency, sharing, optimal design methods, publication practices, and incentives and rewards in science.”
Outdated tools:
Not everyone is convinced the ASA’s recommendations will have the desired effect.
“Statisticians have been calling for better statistical practices and education for many decades, and these calls have not resulted in substantial change,” Trafimow says. “I see no reason to believe that the special issue or editorial would have an effect now where similar calls in the past have failed.” Trafimow does, however, acknowledge that some areas of research are changing, and says perhaps the special issue can help accelerate that change.
Others question the ASA’s approach. “I don’t think statisticians should be telling researchers what they should do,” says Daniël Lakens, an experimental psychologist at Eindhoven University of Technology in the Netherlands. Instead, he adds, they should be helping researchers ask what they really want to know and give more tailored field-specific practical advice. For this reason, Lakens doubts whether the new special issue will improve current practice.
One reason why academics may be forced into cutting corners is the constant pressure to publish papers. “I think the problem is that the research community themselves don’t have a strong incentive for raising the bar for significance because it makes it harder from them to publish,” says Valen Johnson, a statistician at Texas A&M University in College Station, who is a proponent of the p<0.005 threshold. But in the long run, Johnson says, raising standards should result in more discoveries and better science, as researchers would have more confidence in previous work and spend less time replicating studies.
Unlike the ASA in its editorial, Johnson believes that researchers, especially non-statisticians, would benefit from thresholds to indicate significance. Lakens, who advocates for researchers to choose thresholds as long as they justify them, agrees, noting that bright-line rules may be necessary in some fields.
But allowing cutoffs, even in select cases, may mean that researchers’ biases encourage p-hacking — even if unconsciously, notes Regina Nuzzo, a statistician at Gallaudet University in Washington, D.C., and associate editor of the ASA’s special issue.
For Nuzzo, a substantial change will require educating researchers during college years and developing software that helps with statistical tests. “If scientists aren’t using the same lab equipment as they did a century ago,” she says, “why are we using the same statistical tools from a hundred years ago?”
It’s also important to leave behind the idea of a binary world of success and failure, Nuzzo says. “We are battling human nature that wants to dichotomize things. In our society, we’re now coming to realize that things aren’t as black and white as we previously thought.”
Disclosure: The author of this story previously worked in a freelance capacity, and on unrelated topics, with science journalist John Bohannon, whose work is referenced in the opening paragraphs.
This article was originally published on Undark. It has been slightly modified to reflect Spectrum’s style.
Recommended reading
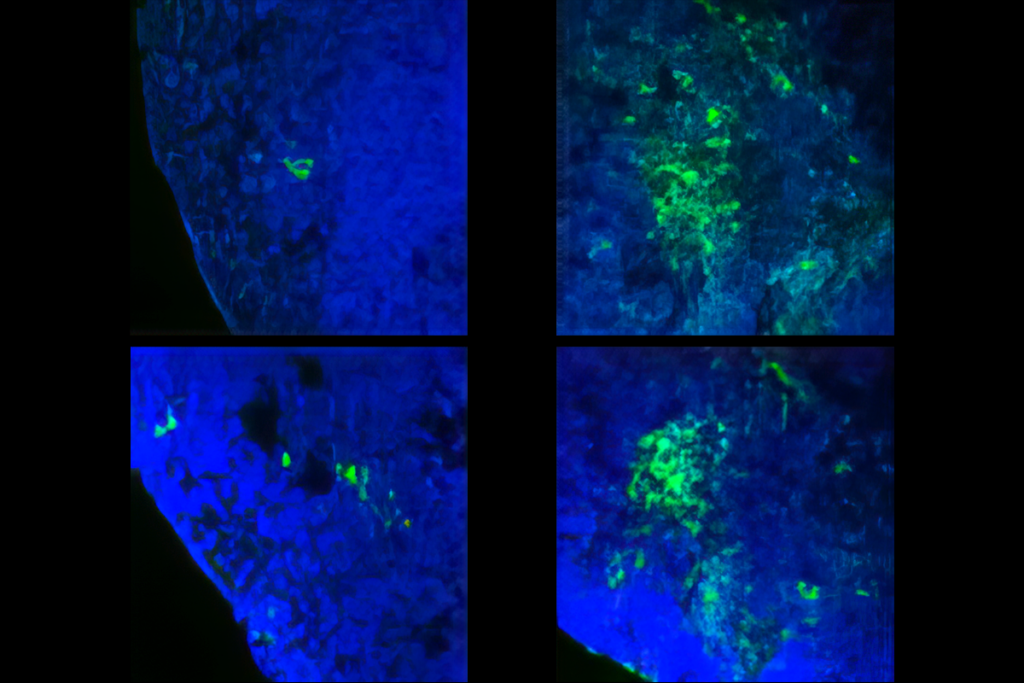
Personalized medicine; astroglia organoids; fast track for fragile X drug
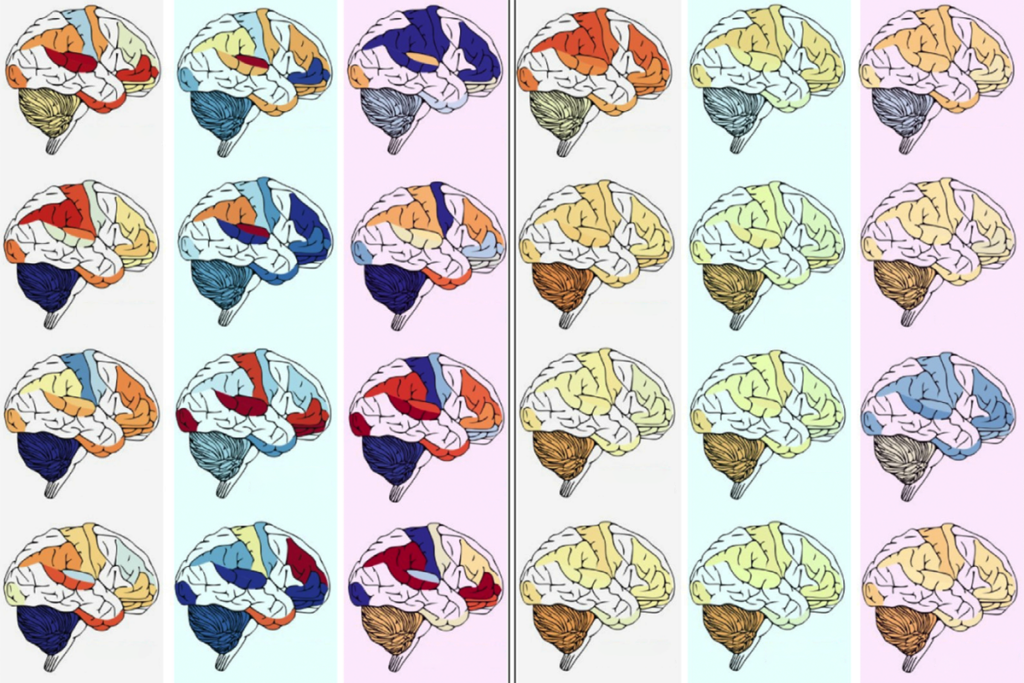
X marks the spot in search for autism variants
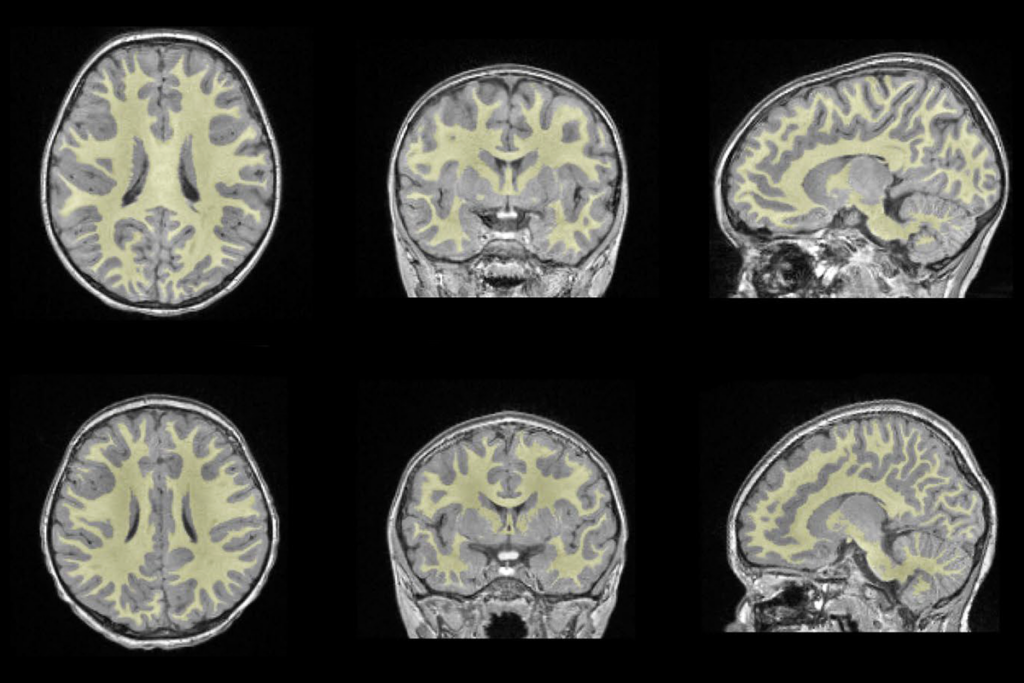
White-matter changes; lipids and neuronal migration; dementia
Explore more from The Transmitter
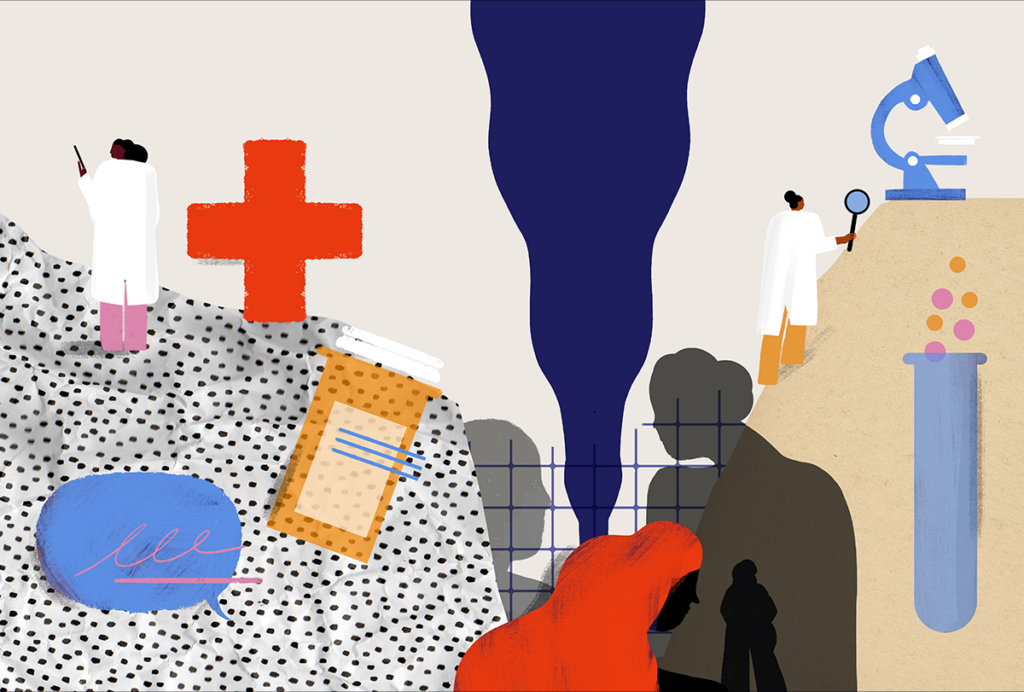
Neuroscientists need to do better at explaining basic mental health research
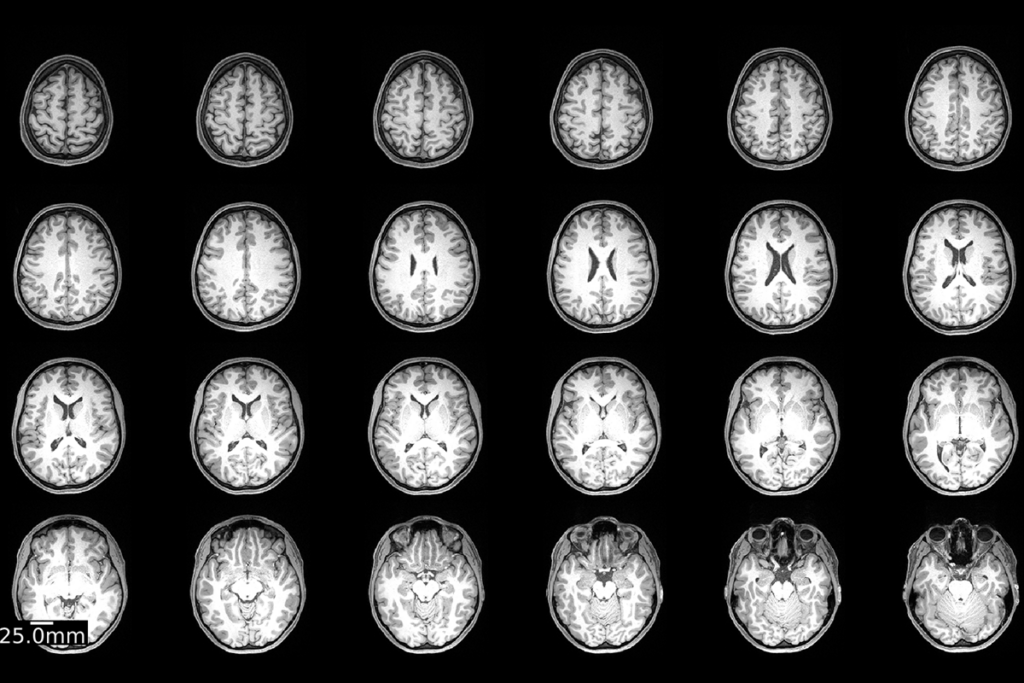
Dose, scan, repeat: Tracking the neurological effects of oral contraceptives
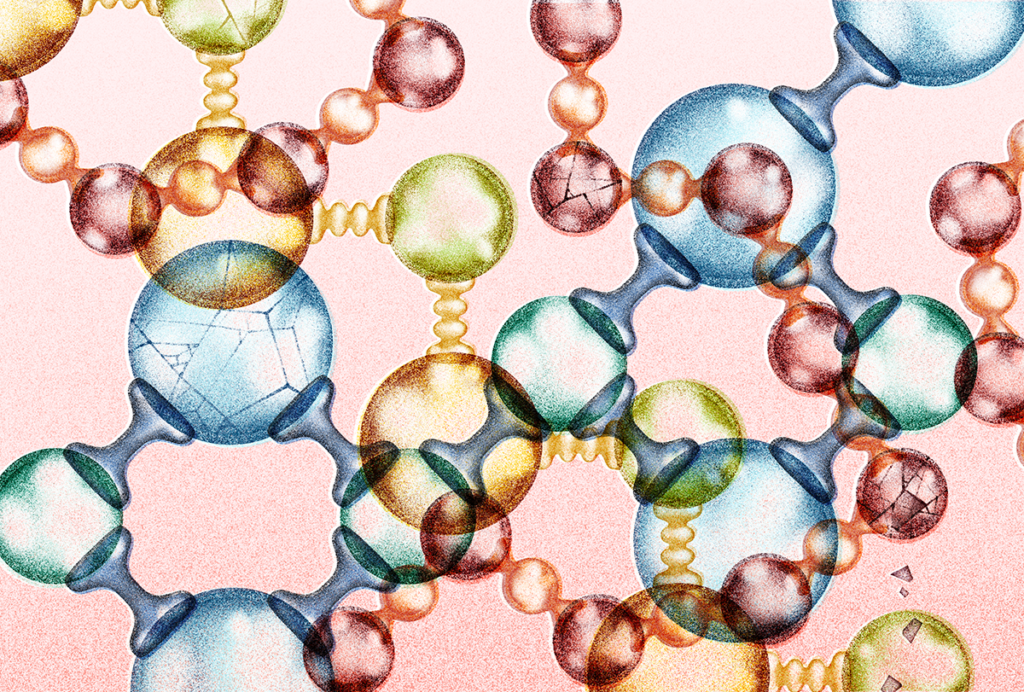