Questions for Pua, Seal: What’s wrong with brain imaging work?
Despite the completion of hundreds of imaging studies in people with autism, researchers have yet to find features that distinguish people with the condition.
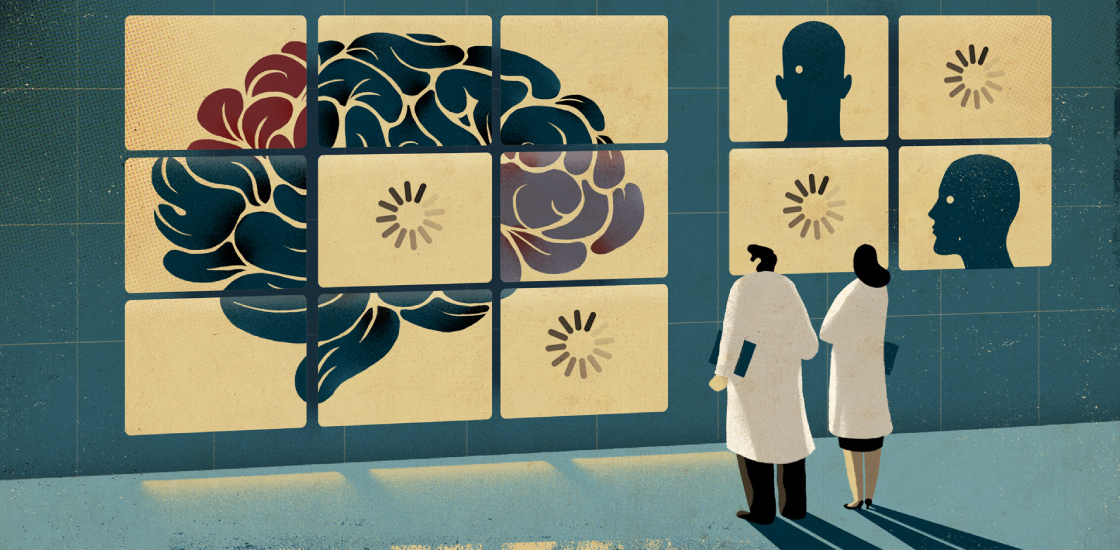
Autism is a condition of the brain, so it seems reasonable to think people with autism should have brain features that distinguish them from neurotypical individuals. Despite the completion of hundreds of studies, however, no one has found structural brain features that mark people with autism reliably and accurately.
A team of researchers at Murdoch Childrens Research Institute in Melbourne, Australia, set out to better understand why this is so. They compared the findings from 12 meta-analyses, each of which looked at multiple studies, to see where various analyses agree or diverge.
They found that the inconsistencies boil down to variations in the methods different teams use, the ages and other characteristics of the study participants, and shifting diagnostic criteria. The analysis is set to be published in February in Research in Autism Spectrum Disorders1.
We asked Marc Seal and Emmanuel Pua, who led this detective work, how researchers can minimize the noise in brain imaging data.
Spectrum: What did you find in your analysis?
Emmanuel Pua: Our goal was to better characterize the problem of inconsistent brain imaging findings in autism research. We focused on the volume of gray matter, which is made up of the cell bodies of neurons. We chose it because it’s a single measurement and is reliably quantitative for this kind of analysis.
There are numerous reports of gray-matter abnormalities in autism, but we found they are inconsistent in terms of both the brain regions in which they appear and the direction of those differences. Some meta-analyses found increases in gray-matter volume in certain regions, whereas others found a reduction in volume in the same regions.
It’s quite interesting that the results are so different between meta-analyses. It’s even more interesting when you consider that some meta-analyses that arrive at different conclusions include some of the same studies.
S: What might explain these inconsistencies?
EP: All of the meta-analyses we looked at had different criteria for the studies they included. And all of the studies had different diagnostic criteria for the people they included. This variability could be masking important differences in brain structure between people with autism and controls. Autism is heterogeneous and its subgroups may have different brain features.
Another inconsistency across the meta-analyses is the age of the study participants. This is important because there is increasing evidence that there are age-dependent differences in brain structure and function. How the brain changes with age in a child with autism differs from the developmental trajectory seen in typical individuals.
Studies also differ in methodology. Different labs have different scanners and protocols for acquiring and analyzing magnetic resonance imaging (MRI) data. This makes it really hard to compare data from different sites.
Another complication is head motion in the scanner, which can skew results. People with autism could have systematic ways of moving in the scanner that could explain differences between groups. So any differences between people with autism and controls could just be due to head motion. That is something that needs to be controlled well when looking at MRI data.
Marc Seal: We now know that to accurately track neurodevelopment, we need large datasets that include three or more scans per individual. To maximize the number of participants, we need to collect data from multiple sites. But this comes with a cost: Different sites have different scanners and protocols, and these differences can add noise to the data.
S: Did you find any consistent brain differences?
EP: It’s difficult to find a brain area that is consistently different in people with autism compared with controls across all studies. Some brain areas, such as the temporal lobe, may appear more relevant than others. But it’s also possible that we will not find anything consistent for such a heterogeneous condition.
Over the past decade or so, there has been a shift from looking for structural brain changes in autism to exploring changes in how brain regions are functionally connected. We may find more revealing patterns among autism brains in this functional measure than we do using conventional brain structure methods.
S: How can researchers minimize variability across studies?
EP: I think our results highlight the importance of following the same individuals over time. A longitudinal approach allows us to capture variations between individuals as well as within the same individual across the lifespan.
We should also continue using large databases such as the Autism Brain Imaging Data Exchange (ABIDE), which contains brain scans and clinical data from around the world. This allows us to see if findings from one site match those from other sites. If findings can be replicated across different sites, that would increase people’s confidence in them.
MS: Our work with the ABIDE I dataset has revealed that the largest contributing factor to differences in brain structure is not whether a person has autism, but at which site he or she was scanned. This accounts for about 20 percent of the variance. There are a range of statistical techniques that can address this issue, but it’s always best to minimize the potential for noise before collecting the data.
The Baby Connectome Project and the Human Connectome Lifespan project have set the standard for multisite studies. In these projects, the lead investigators have worked hard to minimize the sources of noise by using the same types of scanners and software at every site, and regularly checking this equipment for differences cropping up between sites.
References:
- Pua E.P.K. et al. Res. Autism Spectr. Disord. 34, 28-33 (2017) Abstract
Recommended reading
Assembloids illuminate circuit-level changes linked to autism, neurodevelopment
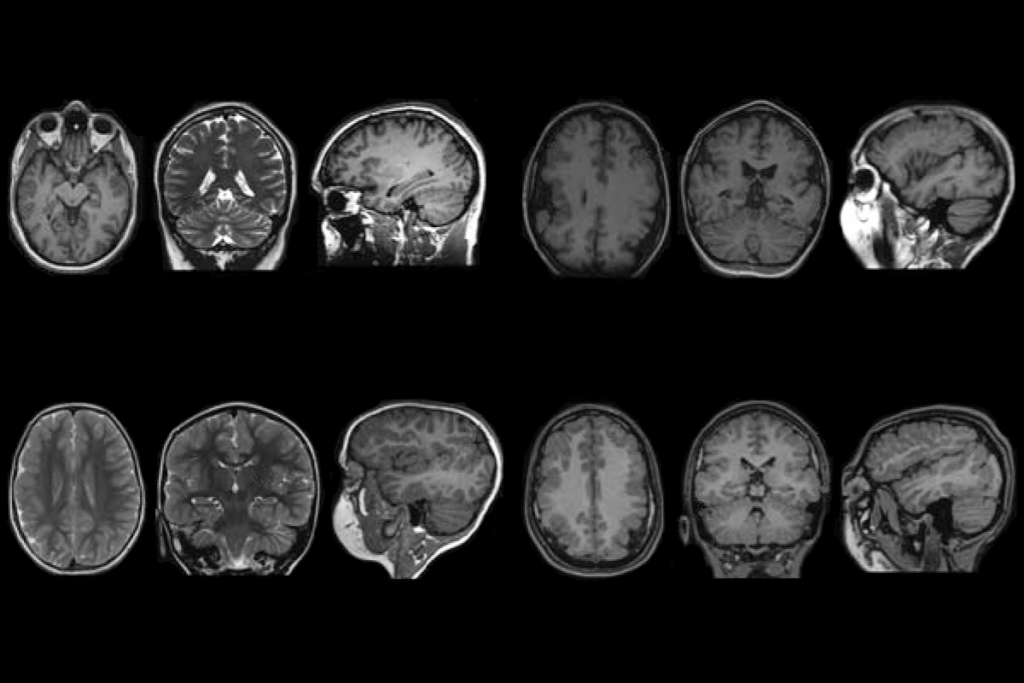
Impaired molecular ‘chaperone’ accompanies multiple brain changes, conditions
Explore more from The Transmitter
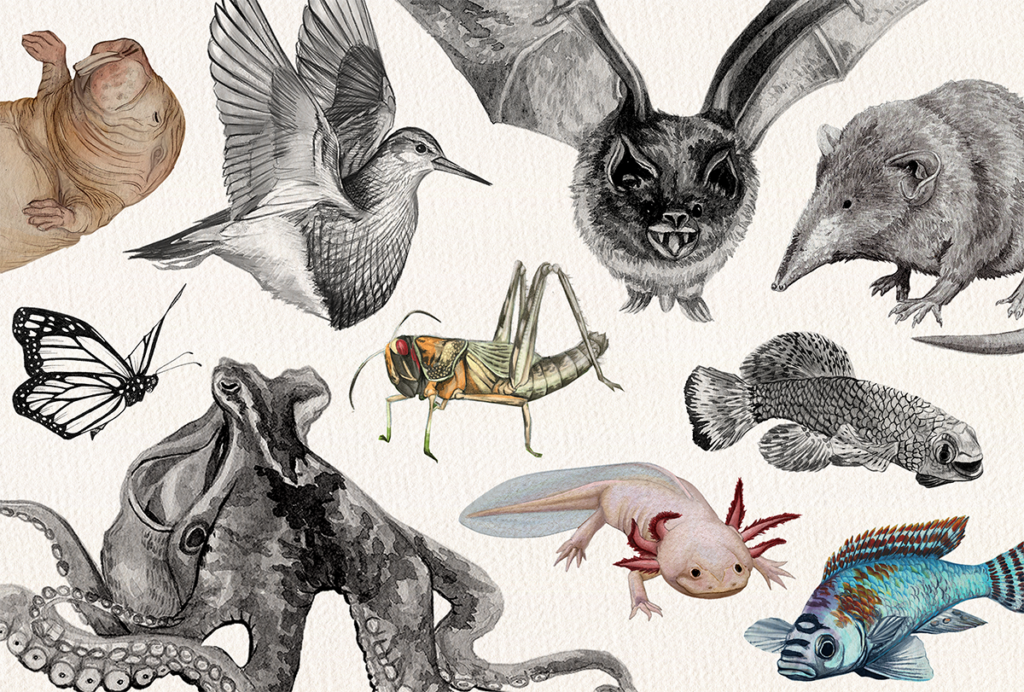
The non-model organism “renaissance” has arrived
Rajesh Rao reflects on predictive brains, neural interfaces and the future of human intelligence
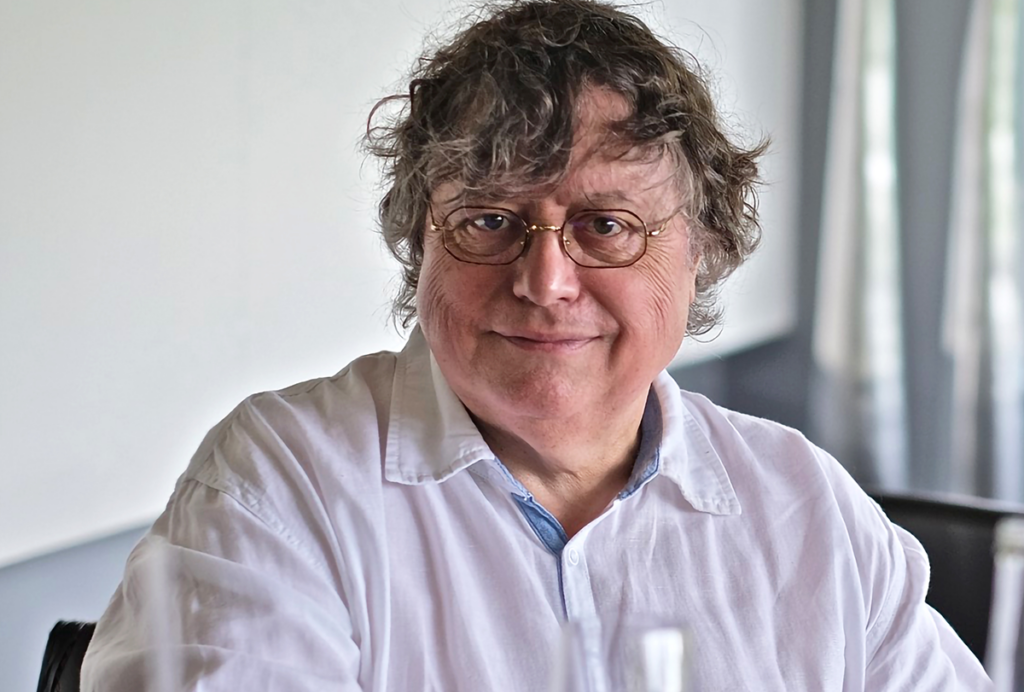