In quest for autism biomarkers, this technique has magnetic appeal
To find biological markers of autism, scientists would be wise to measure the brain’s electrical activity along with the resulting magnetic fields.
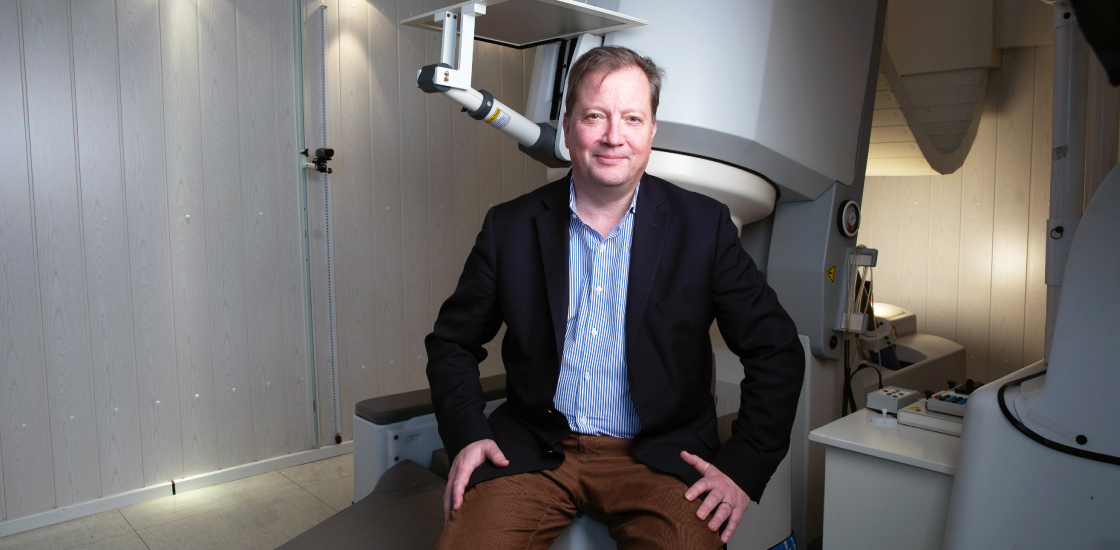
There are no good biological markers for autism, and that dearth has broad consequences.
We usually think of biomarkers as aiding in diagnosis, the way a blood test can point to an infection. But a biomarker — broadly defined, an objectively measured index of a condition — can also aid in prognosis, track progress, stratify people into subgroups, monitor treatment or evaluate whether a therapy is effective.
Noninvasive brain imaging such as magnetic resonance imaging (MRI) and other assays of brain activity have gained attention as ways to identify biomarkers for autism. These methods can reveal details of brain structure and hint at regional brain chemistry. But they cannot capture the real-time fluctuations associated with seeing, hearing and thinking.
By comparison, electrophysiological techniques such as electroencephalography (EEG) and magnetoencephalography (MEG) are well suited to this purpose. EEG tracks the brain’s electrical activity via electrodes affixed to the scalp; MEG detects the magnetic fields that electrical activity produces. Both are sensitive to the nuances of neural activity that characterize the brain at work.
Both methods can track neuronal activity at submillisecond speeds. MEG can characterize oscillatory brain rhythms, large-scale electrical patterns arising from activity in countless local circuits. These oscillations appear to be disrupted in autism. By tracking oscillations, MEG provides a window into the activity of these circuits, and possible biological targets for interventions.
EEG is accessible, readily available and relatively inexpensive. By contrast, MEG machines are expensive to purchase and operate, and their results require specialist interpretation. But MEG has technical advantages that could help researchers evaluate candidate biomarkers faster and more accurately.
In developing biomarkers for autism, scientists would be wise to combine the two technologies1.
High resolution:
As electrical signals travel from their origins to the surface of the head, they wend their way through gray matter (cell bodies), white matter (nerve fibers), skull and scalp. Those tissues’ electrical conductivity is not well known, and their thicknesses vary from one person to the next. Both factors shape how and where electrical potentials propagate.
So by the time electrical signals reach the scalp, it is difficult to determine their origin. With EEG, it is also difficult to separate the neuronal activity of interest from electrical artifacts created by scalp muscle activity, for example.
Brain tissues, skull and scalp do not distort magnetic fields to the same extent. So MEG more faithfully reflects the anatomical origin of neural signals. This increased spatial fidelity makes it possible to identify and discard artifacts such as signal from scalp muscle.
MEG arrays have good coverage and high resolution because they commonly have several hundred sensors — 275, in one common setup; most EEG arrays use fewer electrodes, typically 64 or 128 for EEG instruments used in research.
Finally, MEG may be more practical in some situations, as some children with autism may be unable to tolerate electrodes on the scalp.
MEG does have drawbacks, however. Its systems require extreme cooling, typically through bathing in liquid helium. Because of the extreme sensitivity of the detectors, they generally must be housed in rooms shielded from external electromagnetic fields.
Different drummer:
Electrophysiological studies have already identified potential autism biomarkers. More than a decade ago, my lab’s MEG studies of autistic children revealed a characteristic delay during the first stage of auditory processing, as signals travel from ear to primary auditory cortex2. This tiny shift in what is known as the auditory evoked M100 response occurs around 100 milliseconds after the sound hits the ear. It may be related to the problems in auditory processing, speech and language that are so prevalent in autism.
The length of the delay is variable: in some children, the M100 is actually an M110; in others closer to an M111. The longer the delay, the more severe the autism — suggesting that the length of the delay might serve as a biomarker3.
Another potential biomarker is the quality of neural oscillatory activity in the gamma band (30-80 Hz). Brain-wide oscillatory patterns indirectly reflect the balance of the neurotransmitters gamma-aminobutyric acid and glutamate in the underlying circuitry — a balance thought to be disrupted in autism. Researchers have associated differences in these oscillatory rhythms with autism.
If an unusual auditory delay or oscillatory rhythm could be identified early on — for instance, in infants at high risk of autism — it might assist in diagnosis and prognosis. The pattern of delays or differences also could help in treatment selection. For example, M100 delays accompanied by gamma-band deficits may implicate dysfunction in the synapse, and suggest the use of treatments that target this mechanism.
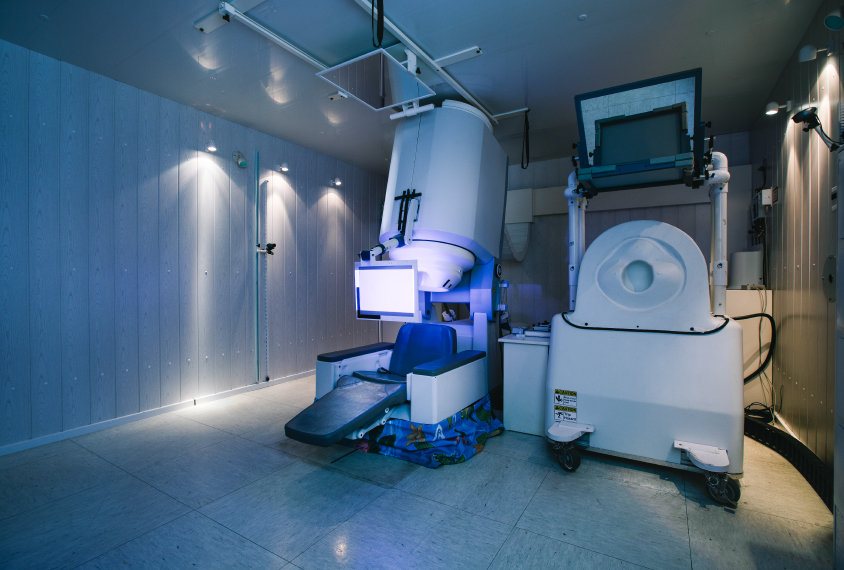
All together now:
MEG might be used to vet potential biomarkers and prioritize them for larger clinical trials. It could identify those measures that are robust enough for widespread use with EEG, which is more practical for use on a large scale.
For some candidate biomarkers, such as some latency measures, EEG can approximate the superior discriminatory power of MEG. To establish whether EEG is good enough to detect a particular biomarker, researchers could use both EEG and MEG in a small subset of the trial population to reveal their relative power. If EEG is nearly equivalent to MEG, then EEG can confidently be adopted in the trial as a whole. But if not, MEG should probably be used in the study, even though few clinical sites have MEG machines, or the measure should be dropped. The researchers would then have to evaluate the practicality of the biomarker.
Technological advances in the works could make MEG more widespread. The capital costs of MEG remain high. But there are recycling systems that reduce the need to replace liquid helium for cooling and can be used as retrofits to legacy systems. These can lower operational costs to levels comparable to those for EEG.
Although only about 200 MEG systems exist worldwide, they are available in most major metropolitan areas in North America through teaching hospitals. Researchers have developed MEG helmets small enough for infants as young as 1 or 2 months of age. Also in the works are wearable MEG devices that could record a child in motion.
In the long run, the promise of combining EEG and MEG is the potential for more accurate and specific diagnosis and prognosis. It may also enable researchers to match the treatment to the person at the appropriate time, realizing the goal of precision medicine.
Timothy Roberts is Oberkircher Family Chair in Pediatric Radiology at the Children’s Hospital of Philadelphia, and professor of radiology at the University of Pennsylvania.
References:
Recommended reading
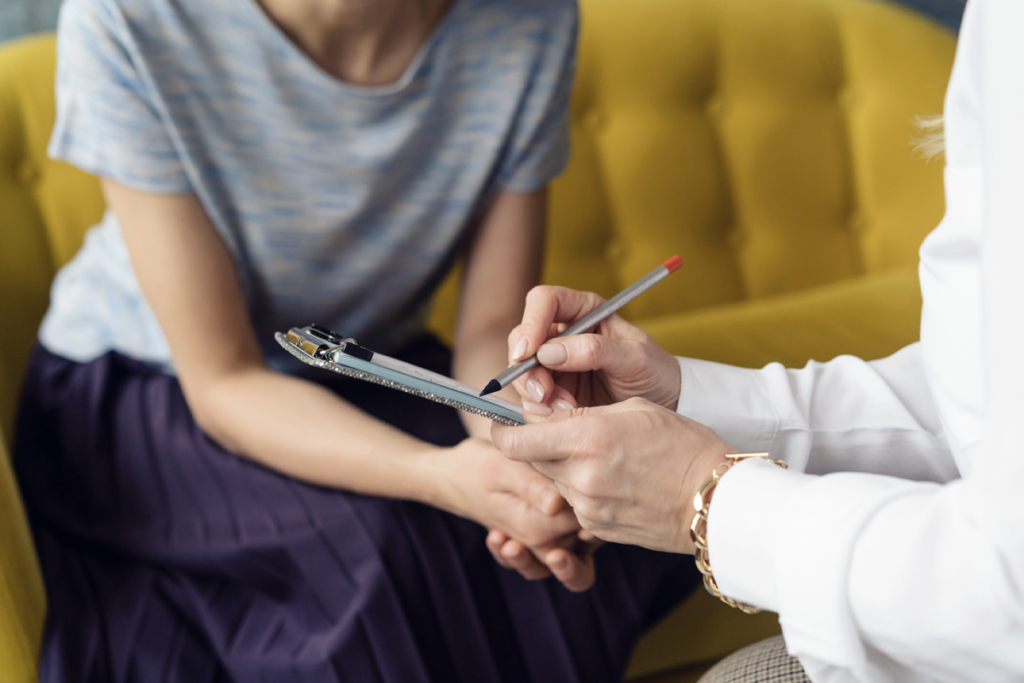
Expediting clinical trials for profound autism: Q&A with Matthew State
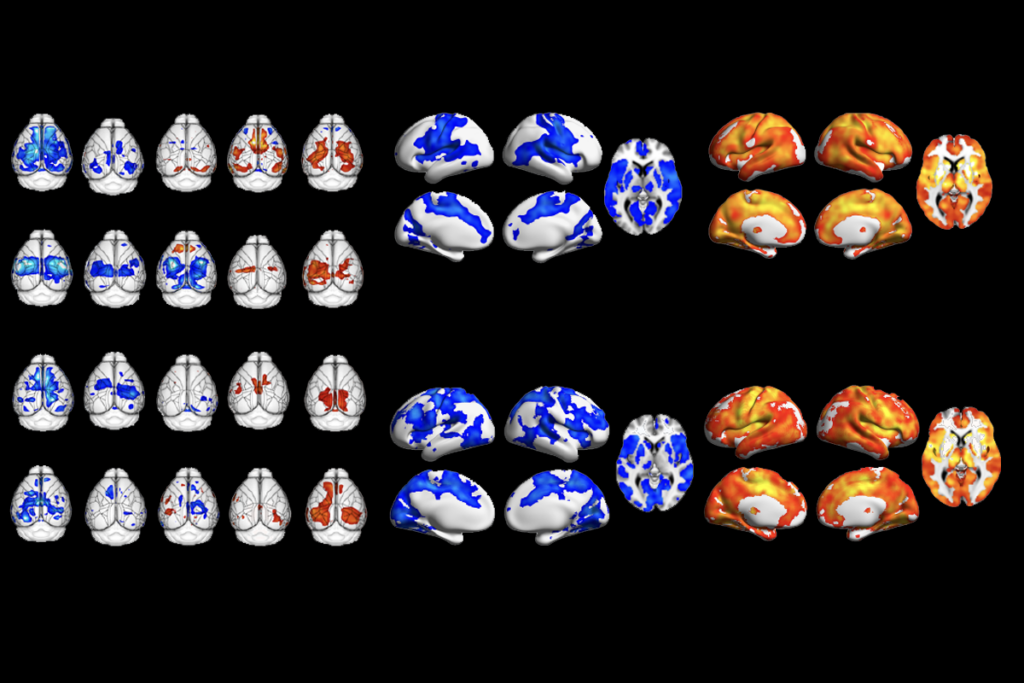
Too much or too little brain synchrony may underlie autism subtypes
Explore more from The Transmitter
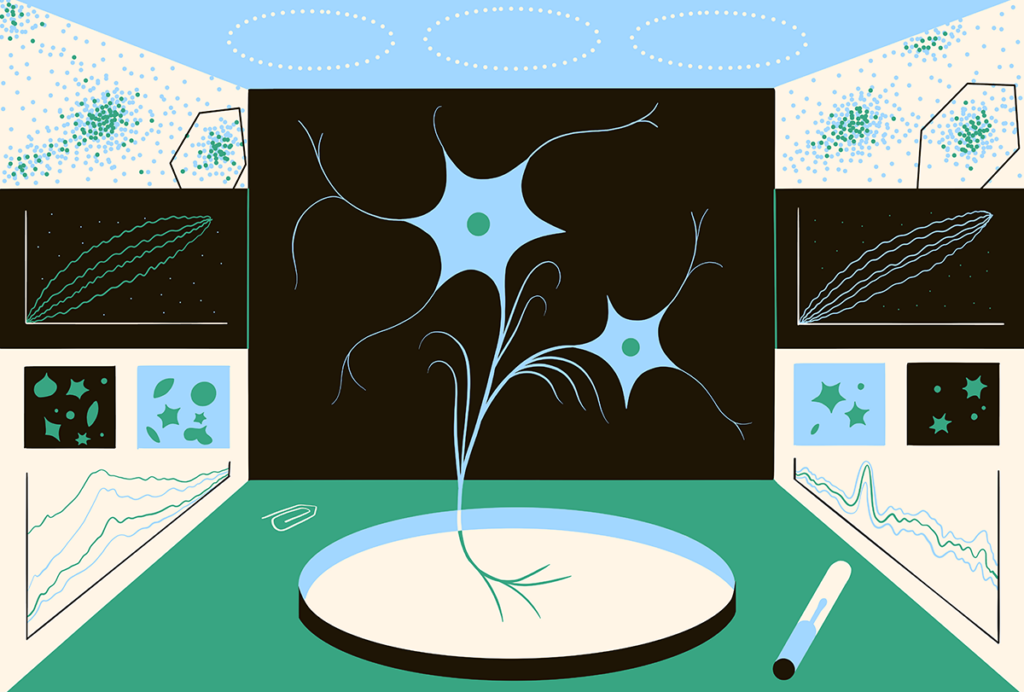
This paper changed my life: Shane Liddelow on two papers that upended astrocyte research
Dean Buonomano explores the concept of time in neuroscience and physics
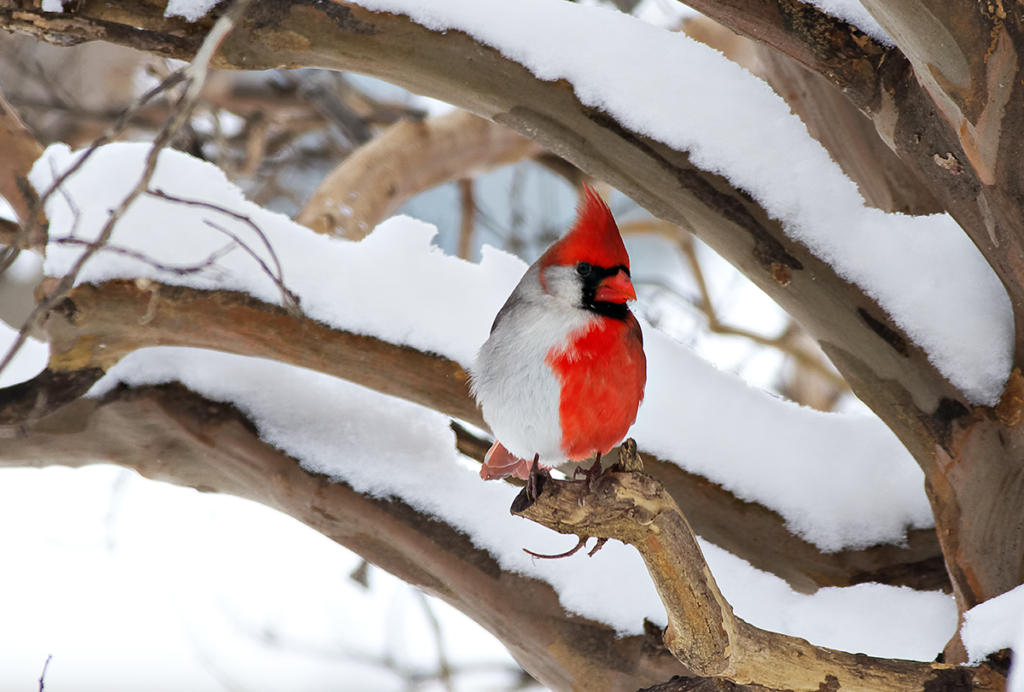