New model merges data streams to boost gene discovery
A new statistical model pulls together information about inherited and spontaneous mutations in a single analysis to enhance the search for autism candidate genes. The method, called transmission and de novo association, or TADA, was described 15 August in PLoS Genetics.
A new statistical model pulls together information about inherited and spontaneous mutations in a single analysis to enhance the search for autism candidate genes.
The method, called transmission and de novo association, or TADA, was described 15 August in PLoS Genetics1.
Unlike traditional genetic analyses, which rely on algorithms that search for mutations in only one type of genetic data at a time, TADA can layer two or more sets of genetic data together, such as inherited and de novo mutations. De novo mutations are rare genetic errors that appear spontaneously in a child.
This method increases the chances of turning up multiple hits on the same new gene, implicating that gene in autism.
“This stage of gene discovery is probably one of the most exciting times in autism research,” says Joseph Buxbaum, professor of psychiatry at the Mount Sinai School of Medicine in New York and a member of the research team.
Our understanding of autism genetics has surged forward in the past decade, thanks to several studies revealing new genetic suspects for autism2. Just last year, three sequencing studies published in the same issue of Nature fingered six genes with de novo mutations3, 4, 5. Other studies have focused on rare inherited mutations6, 7.
Several large-scale sequencing projects, planned or underway, may turn the stream of new genetic data into a torrent, but finding autism candidate genes within it is the real challenge.
“Generally speaking, this is a more specific problem for diseases like autism because there are so many genes involved and no one really knows what their function is,” says Stefan Mundlos, professor of human genetics at the Max Planck Institute for Molecular Genetics and Charité-Universitätsmedizin Berlin, who was not involved in the study.
Some teams have estimated the number of autism candidate genes at about 300, but in the new study, the researchers peg that number at around 1,000.
“We’re never going to find the full 1,000, but if we find a pretty big number of them, then we’ll start to understand the networks that work together and possibly get closer to having some sort of therapy or understanding of the development of autism,” says lead investigator Kathryn Roeder, professor of statistics at Carnegie Mellon University in Pittsburgh.
Stronger signals:
Traditional methods analyze inherited and de novo variants using separate models predicting their likelihood of being involved in autism risk. These methods are not powerful enough to find many candidate genes from looking at either inherited or de novo mutations by themselves, however. They are also expensive and, even with large sample sizes, have sometimes yielded nothing.
Roeder says she first hit upon the idea for TADA while working on de novo studies and feeling frustration at the small return on her effort. For example, after combing through the exomes — the protein-coding portions of the genome — of the members of ten families, she and her colleagues might find a single damaging de novo mutation.
“It seemed kind of amazing to have the full sequence on 30 individuals and only have one piece of information,” she recalls.
In contrast, analyzing inherited and de novo mutations together might highlight genes with errors in both datasets. This is akin to layering two transparent photos of the same patch of starry sky on top of each other: The stars that appear in both photos seem to shine brighter.
Roeder’s team built statistical models to handle more than one dataset at once. She and her colleagues used existing exome sequence data from four studies of 932 families and inherited mutations from about two-thirds of these families. They combined this with data from studies that compared the genes of 935 people with autism and 870 controls from the Autism Sequencing Consortium.
The analysis pulled out de novo mutations that turned up in two or more individuals. This analysis gave them a shortlist of candidate genes in which to look for inherited errors.
They then combined this smaller list with datasets containing rare inherited mutations, zeroing in on the part of the data that gave the strongest signal. The process dramatically boosts the power to identify risk genes.
By taking advantage of data on inherited mutations, the new analysis allows researchers to account for them as important contributors in autism, says Stephen Scherer, director of The Centre for Applied Genomics at The Hospital for Sick Children in Toronto, who was not involved in the study. “This new paper represents a very positive step in this direction.”
As powerful as the new method is, it can’t be applied in the clinic right away. Instead, says Roeder, the method will guide experiments and data collection in the next year or two. “This can be used for in-depth sequencing at much larger sample sizes, and then there will be payback.”
References:
- He X. et al. PLoS Genet. 9, e1003671 (2013) PubMed
- Buxbaum J. et al. Neuron 76, 1052-1056, (2013) PubMed
- Sanders S.J. et al. Nature 485, 237-241 (2012) PubMed
- O’Roak B.J. et al. Nature 485, 246-250 (2012) PubMed
- Neale B.M. et al. Nature 485, 242-245 (2012) PubMed
- Lim E.T. et al. Neuron 77, 235-242 (2013) PubMed
- Yu T.W. et al. Neuron 77, 259-273 (2013) PubMed
Recommended reading
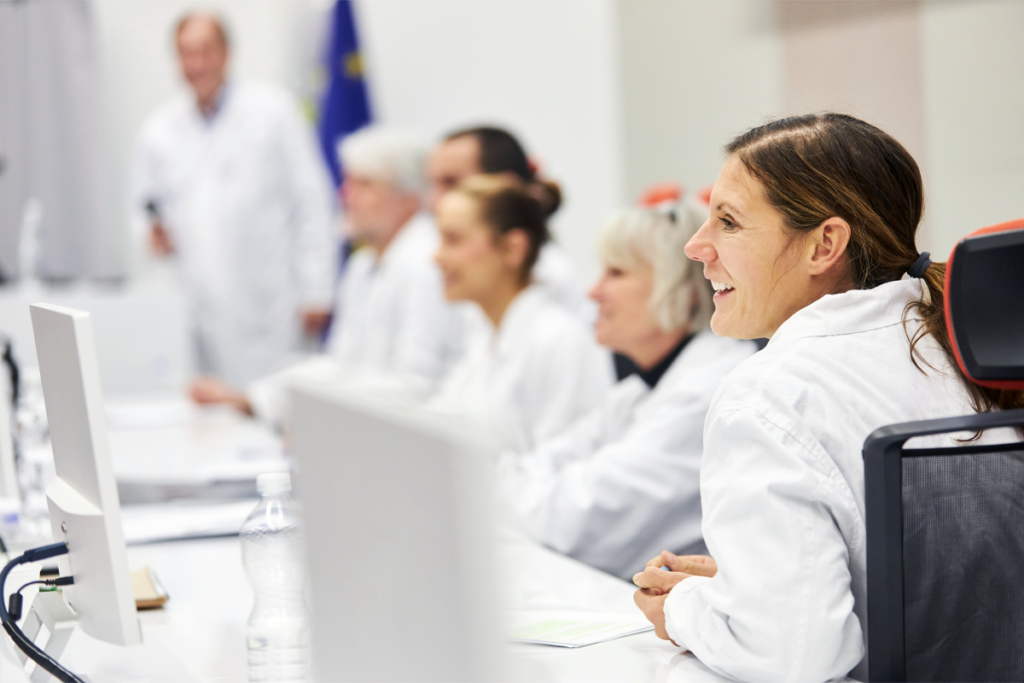
INSAR takes ‘intentional break’ from annual summer webinar series
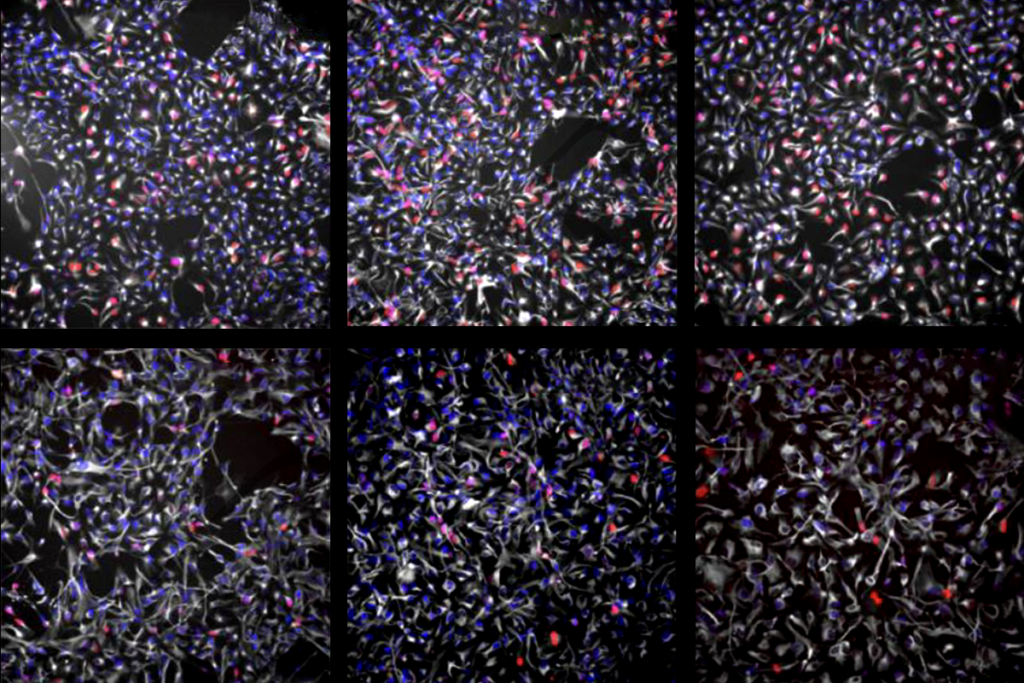
Dosage of X or Y chromosome relates to distinct outcomes; and more
Explore more from The Transmitter
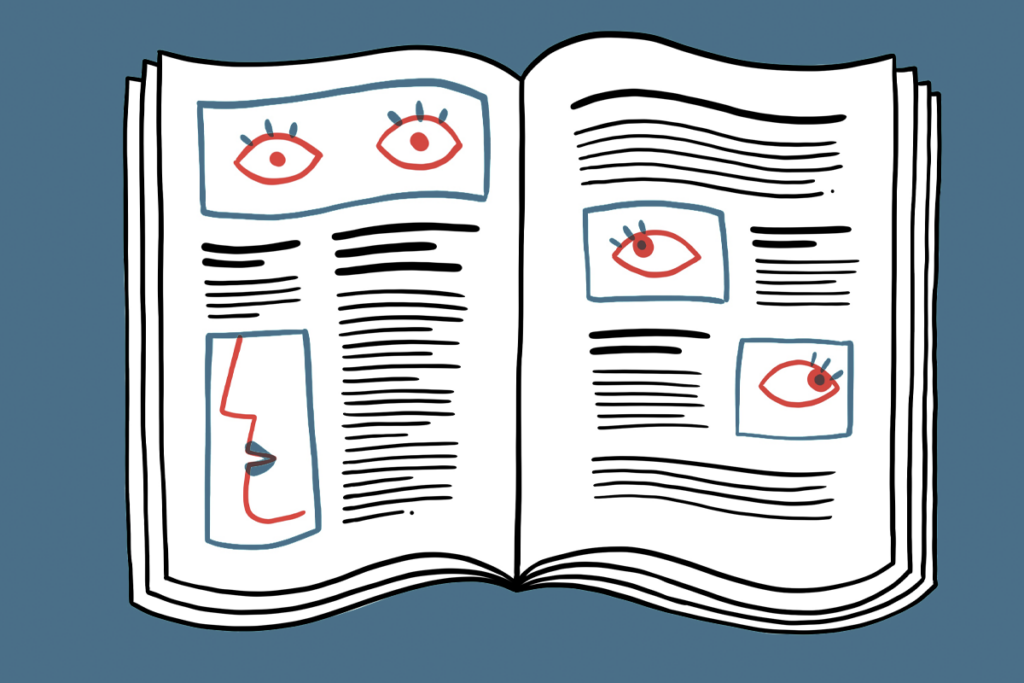
Anti-seizure medications in pregnancy; TBR1 gene; microglia
Grace Hwang and Joe Monaco discuss the future of NeuroAI
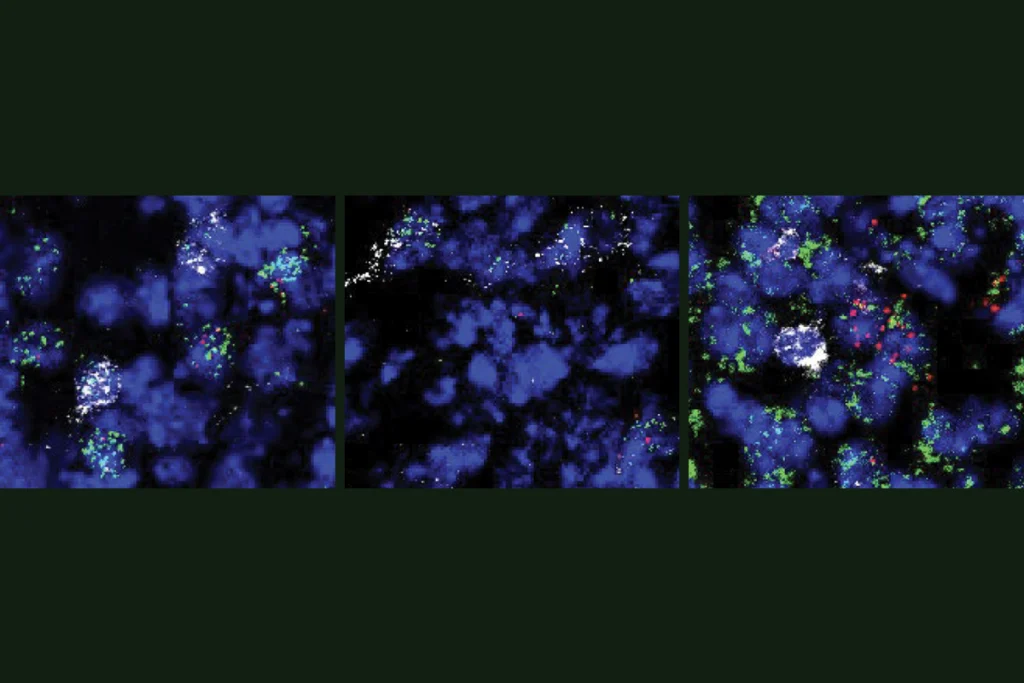