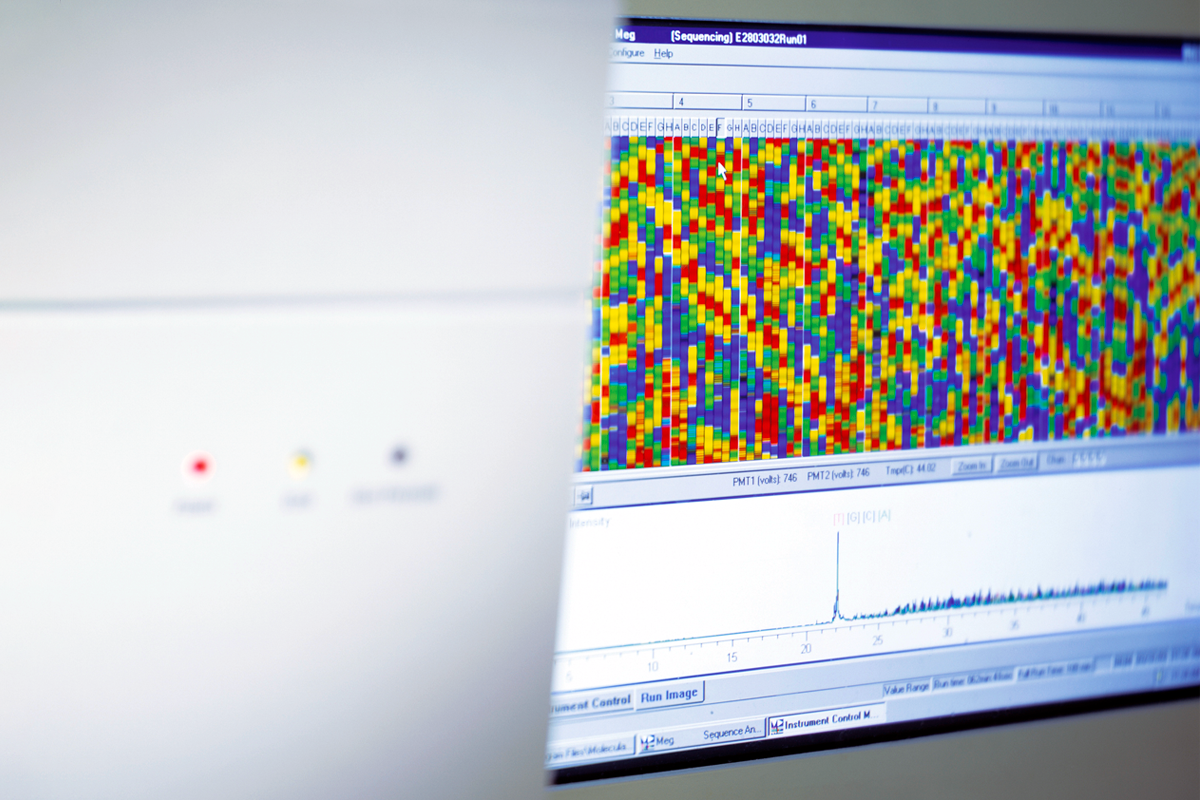
Method predicts impact of DNA variants on gene expression
A new computational approach predicts how sequence variations in both the coding and noncoding regions of a gene affect the gene’s expression. The method, described today in Science, may help researchers understand how specific variants contribute to disorders such as autism.
A new computational approach predicts how sequence variations in both the coding and noncoding regions of a gene affect the gene’s expression. The method, described today in Science, may help researchers understand how specific variants contribute to disorders such as autism1.
The researchers also used the method to analyze genetic variants in people with autism. They identified 171 genes predicted to have some role in the disorder, though more work is needed to firmly establish them as autism candidates.
Autism is highly heritable, with studies attributing anywhere from about 50 to 90 percent of risk to genetics. But the genetic causes of autism can be pinned down in only about 20 percent of autism cases.
Most studies looking for risk factors have focused on the exome — the small fraction of the genome that encodes proteins. Exome sequencing studies have uncovered 50 genes that are strongly linked to autism. But the remaining 97 percent (or more) of the genome remains relatively unexplored.
“The leaders in the autism community say that they have to go after noncoding mutations,” says lead investigator Brendan Frey, professor of electrical and computer engineering at the University of Toronto in Canada. “We have a tool that allows you to do that.”
Few studies have sequenced the entire genomes of people with autism. A 2013 study sequenced the whole genomes of 32 children with autism and each child’s parents, linking thousands of previously unidentified mutations to autism. But it limited its analysis to mutations in coding regions, the effects of which are easiest to interpret.
“When they do get the whole genome sequence, the problem is that nobody knows what to do with noncoding variants,” says Lilia Iakoucheva, assistant professor of psychiatry at the University of California, San Diego, who was not involved in the study. The new technique is an important step in interpreting noncoding variants, she says.
Variable genes:
The first step in translating a gene into a protein involves the creation of an RNA-based copy of a gene. This messenger RNA then undergoes a process called splicing, in which the noncoding regions, called introns, are cut out, leaving behind only the coding segments, or exons, to join together.
Most genes undergo alternative splicing, in which the exons of a gene can be pieced together in various combinations. This results in multiple versions of messenger RNA being produced from a single gene, and gives rise to proteins with divergent properties in different tissues.
This process goes awry in a range of disorders, including autism. A 2011 study found altered splicing patterns in the postmortem brains of people with autism2.
The new tool predicts how variations in the sequence of a gene influence how it is spliced.
“Understanding splicing diversity and the impact of genetic variation in terms of splicing could help with our understanding of the genetic basis of autism,” says Benjamin Neale, assistant professor of analytic and translational genetics at Massachusetts General Hospital, who was not involved in the research.
To develop the tool, Frey and his team fed DNA sequences of 10,689 gene fragments, each of which is known to undergo alternative splicing, into a computer. Each fragment comprises three exons interrupted by two introns; the center exon is sometimes left out of the messenger RNA. The computer scanned these fragments for 1,393 patterns known to influence RNA splicing.
The researchers then entered the sequences of messenger RNAs found in 16 human tissues, including the brain. The computer analyzed the RNAs for the presence of the center exon, and looked for sequence patterns that predict its presence or absence.
“It tries to figure out what we call a splicing code,” Frey says. “It’s a little bit like teaching the system how to read.”
Once the computer has learned to read, it can analyze any sequence. When presented with new DNA sequences, it accurately predicts which exons would be included in the corresponding RNA from each tissue.
Splice machine:
The researchers then used the tool to explore the effects of various genetic variants on RNA splicing. They analyzed more than 650,000 variants: 540,000 common variants known as single nucleotide polymorphisms and 110,000 rare, disease-associated mutations.
The analysis predicted roughly 20,000 variants that may disrupt splicing, including 465 located within introns and 579 so-called synonymous mutations that alter a DNA sequence but not the amino acids it encodes. Traditionally, researchers have had a difficult time interpreting the effects of these two classes of variants.
“As far as I know, this is by far the most advanced effort to try to have a predictive model of whether a variant affects splicing or not,” says Tuuli Lappalainen, a junior investigator at the New York Genome Center who was not involved in the work. “If it works as well as they say, it has substantial value for people doing genetic analysis and trying to predict the functional effects of genetic variants.”
The researchers also used the tool to analyze whole genomes in postmortem brain tissue from 5 people with autism and 12 controls. They identified 171 genes predicted to have abnormal splicing in people with autism. Of these, 39 fall into categories of similar function, such as the transmission of signals between neurons and neuronal growth. Two of the genes — CTNND2 and PTEN — have been implicated in autism.
However, some experts caution that it is too soon to consider these 39 genes strong autism candidates.
“The physiological impact of the splicing changes needs to be validated, and also shown to exist in these brain tissues from autism patients,” says Gene Yeo, associate professor of cellular and molecular medicine at the University of California, San Diego, who was not involved in the study.
Iakoucheva calls the approach a hypothesis-generating tool. “You have to follow up with experiments to see whether a specific gene definitely has disrupted splicing due to a mutation,” she says.
She also notes that the RNAs used to develop the tool were derived from a 77-year-old woman, whereas the postmortem brains used in the autism analysis range in age from 8 to 39. Unpublished data from her lab suggests that splicing patterns vary with age and by brain region.
“Autism is a neurodevelopmental disorder, so it mainly affects the fetal brain,” she says. “If we do care about assigning functional impact of mutations in [autism], maybe this model can be tuned more finely.”
References:
1. Hui Y. et al. Science Epub ahead of print (2014) Abstract
2. Voineagu I. et al. Nature 474, 380-384 (2011) PubMed
Recommended reading
Assembloids illuminate circuit-level changes linked to autism, neurodevelopment
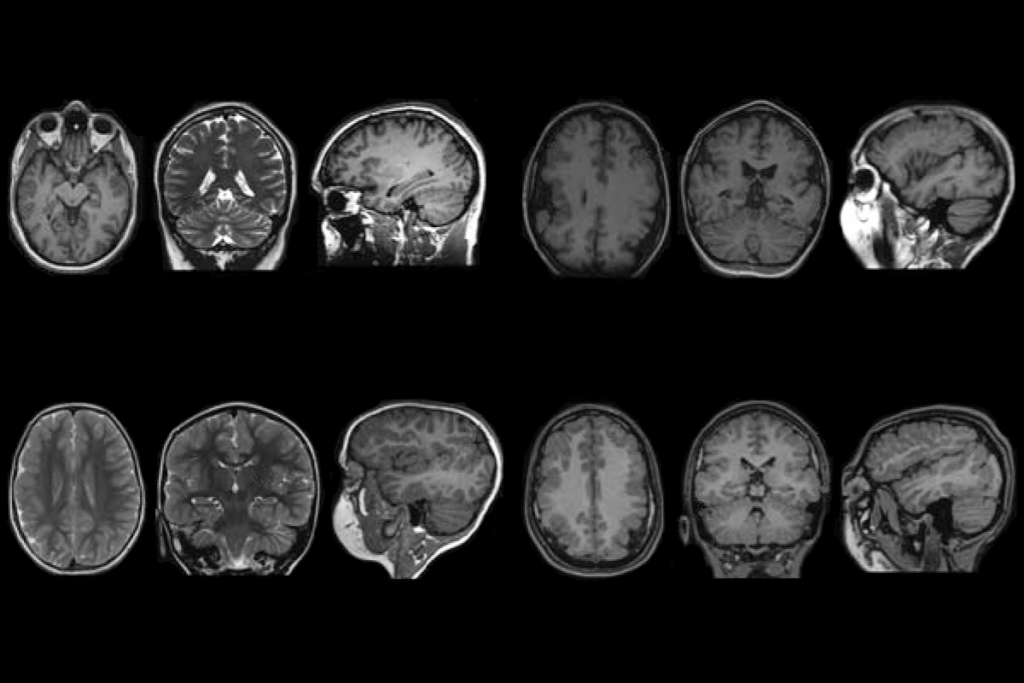
Impaired molecular ‘chaperone’ accompanies multiple brain changes, conditions
Explore more from The Transmitter
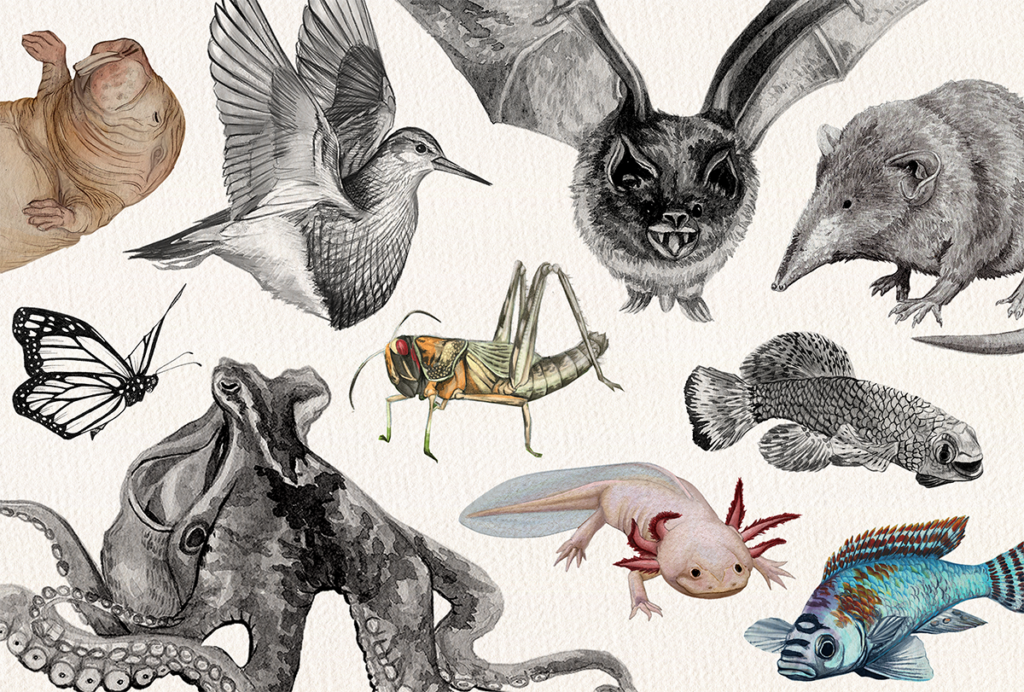
The non-model organism “renaissance” has arrived
Rajesh Rao reflects on predictive brains, neural interfaces and the future of human intelligence
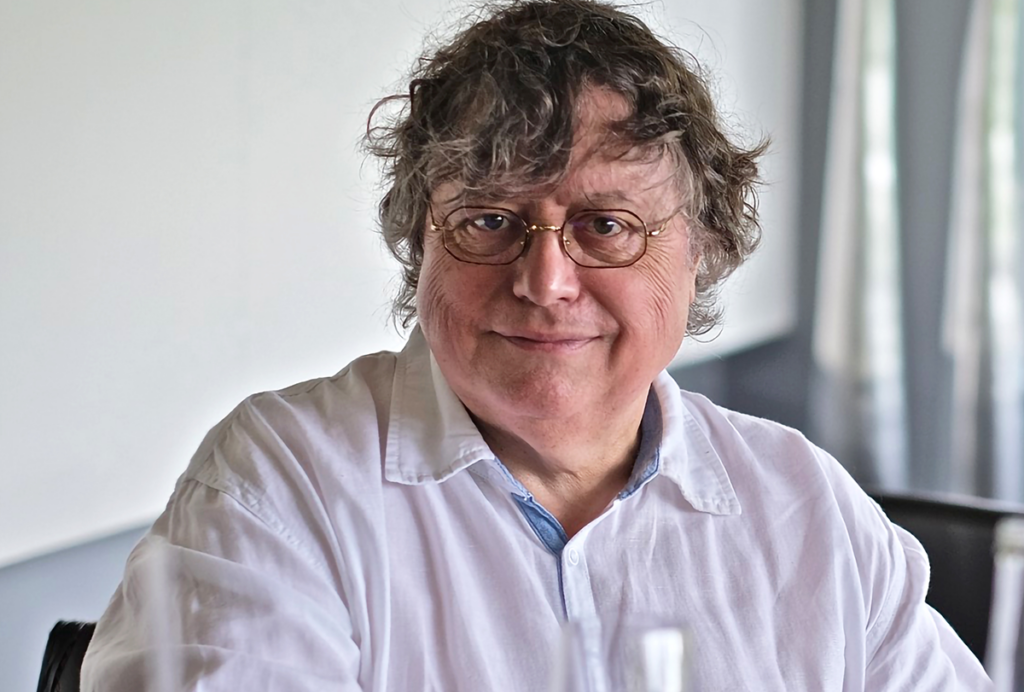