Health records reveal autism’s full picture
Electronic health records may help researchers assemble information about autism, such as its full range of symptoms, from thousands of individuals, says Isaac Kohane.
The structure of the many perturbations that underlie the constellation of symptoms that we call autism is steadily coming into focus1. But this is less as if autism were a tissue preparation viewed under a microscope and more as if it were an iceberg emerging from the Arctic mist.
Autism is much more heterogeneous than we originally thought, with variants of more than 1,000 genes and an ever-increasing list of environmental risk factors implicated in its etiology. But we also now understand that this previously-thought-to-be rare disorder has a vast number of non-neurological symptoms. Until recently, these symptoms were largely unseen and unappreciated — except, that is, by affected individuals and their families.
In this context, automation of the clinical process, most notably electronic health records, can serve the essential function of developing a more complete and representative picture of autism.
Researchers can use electronic health records to identify people who have clinical symptoms of interest. They can then use the samples left over from other clinical diagnostics to characterize the genetics of these individuals2.
The development of algorithms called‘natural language processing techniques’ enables researchers to search these records for more than simple codified data such as laboratory results or medications. Instead, researchers can search for the words that clinicians use to communicate with one another about their patients, obtaining a far more accurate assessment than the codified data alone3. For example, a pediatrician might have noted repetitive behaviors and speech delay in a child months to years before making the diagnosis of autism.
This has been done successfully for several neuropsychiatric disorders, including major depressive disorder4, 5 and bipolar disorder6. Several groups are working on similar approaches for autism.
Easy recruitment:
The merit of this approach is in obtaining large study groups at about one tenth of the cost and one percent of the time that it takes withtraditional methods2. This allows for a genetic study of hundreds of thousands of individuals with the budget used for less than a thousand people.
However, there is a different application of electronic health records that may be even more important in our understanding of autism overall. That is the use of electronic health records to understand the full set of symptomsand clinical trajectories that people with autism traverse — not just their neurobehavioral profile but also their multiple non-neuropsychiatric symptoms.
For example, last year, we conducted one of the largest-ever studies of autism comorbidities using electronic medical records of more than 14,000 individuals with autism over a 15-year period7. This study confirmed well-known findings: for example, a prevalence of about 20 percent of seizures in people with the disorder.
But the study also found that 12 percent of people with autismhave a variety of bowel disorders, and an increased rate of autoimmune disorders, such as inflammatory bowel disease and type-1 diabetes. We also confirmed the well-known increased prevalence of autism in those with fragile X syndrome and tuberous sclerosis complex, and the much less-documented, but also surprisingly high, autism prevalence of five percent in the muscular dystrophies.
These electronic-health-record-driven studies have also shown that such comorbidities are not spread evenly across the population, but cluster within specific subtypesof autism.
Overall, children with autism who have seizures have trajectories or clusters of symptoms and other clinical findingsthat differ from those of children with autism who have anxiety disorders and psychiatric comorbidities, such as schizophrenia or major depression. There are also other subgroups, characterized by frequentear infections and hearing loss, or inflammatory bowel disease, that have their own distinct developmental trajectories.
The existence of these distinct subgroups suggests an underlying biological architecture that may represent distinct causative or compensatory mechanisms.
We have developed software called i2b2 thatallows for the extraction and analysis of electronic health record data. The viral spread of such tools has enabled the remarkably low cost and speed of studies8. These tools allow each healthcare institution to mine the information generated during the course of clinical care for population studies, clinical trial recruitment and improvements in the quality of care.
More than 100 large academic health centers across the world, including more than 80 within the U.S., have adopted i2b2. This allows researchers to search across multiple i2b2 sites to determine the reproducibility of many of their findings across large numbers of individuals with autism. The infrastructure provides appropriate governance, oversight and privacy protection measures 9, 10.
For example, the software requires that each user is a legitimate researcher who is already approved by an institutional review board for the investigation in question. All queries are also fully audited and most require additional oversight for sharing information such as an individual’s name or address.
With increasing awareness of the feasibility of electronic-health-record-driven studies, we will be able to tease apart the massive substructures of autism with unparalleled resolution and efficiency. We can anticipate an era when every autism clinical care visit also contributes to furthering our understanding of the disorder.
Isaac S. Kohane is professor of pediatrics and health sciences technology at Harvard Medical School and Boston Children’s Hospital.
References:
1. Loscalzo J. et al. Mol. Syst. Biol. 3, 124 (2007) PubMed
2. Murphy S. et al. Genome Res. 19, 1675-1681 (2009) PubMed
3. Kohane I.S. Nat. Rev. Genet. 12, 417-428 (2011) PubMed
4. Perlis R.H. et al. Psychol. Med. 42, 41-50 (2012) PubMed
5. Gallagher P.J. et al. Am. J. Psychiatry 169, 1065-1072 (2012) PubMed
6. Pato M.T. et al. Am. J. Med. Genet. B Neuropsychiatr. Genet. 162, 306-312 (2013) PubMed
7. Kohane I.S. et al. PLoS One 7, e33224 (2012) PubMed
8. Kohane I.S. et al. J. Am. Med. Inform. Assoc. 19, 181-185 (2012) PubMed
9. Weber G.M. et al. J. Am. Med. Inform. Assoc. 16, 624-630 (2009) PubMed
10. McMurry A.J. et al. PLoS One 8, e55811 (2013) PubMed
Recommended reading
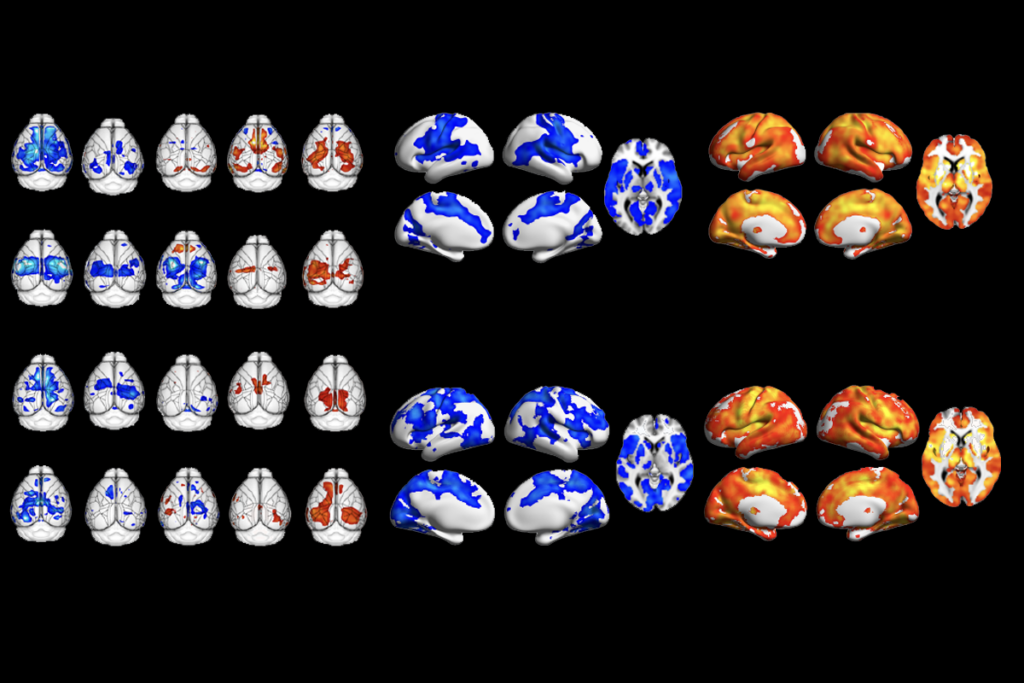
Too much or too little brain synchrony may underlie autism subtypes
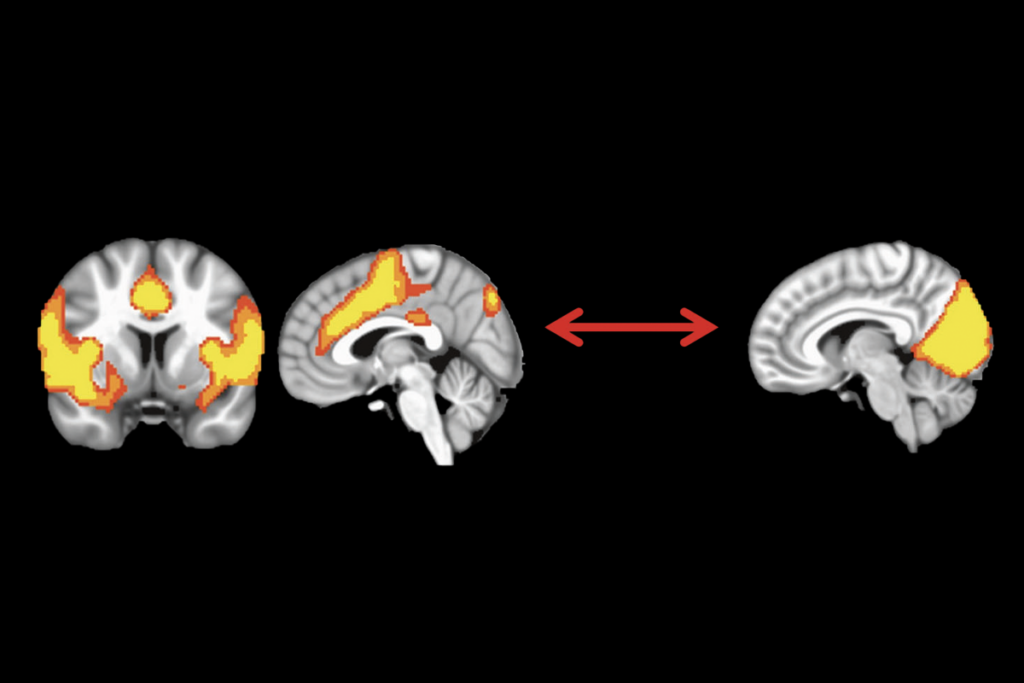
Developmental delay patterns differ with diagnosis; and more
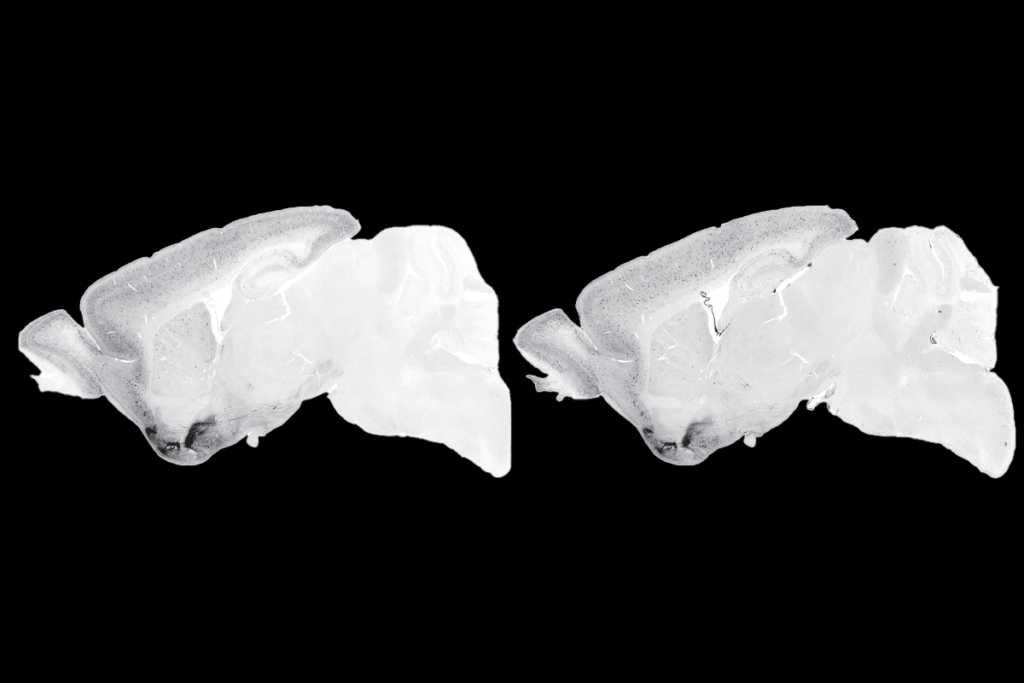
Split gene therapy delivers promise in mice modeling Dravet syndrome
Explore more from The Transmitter
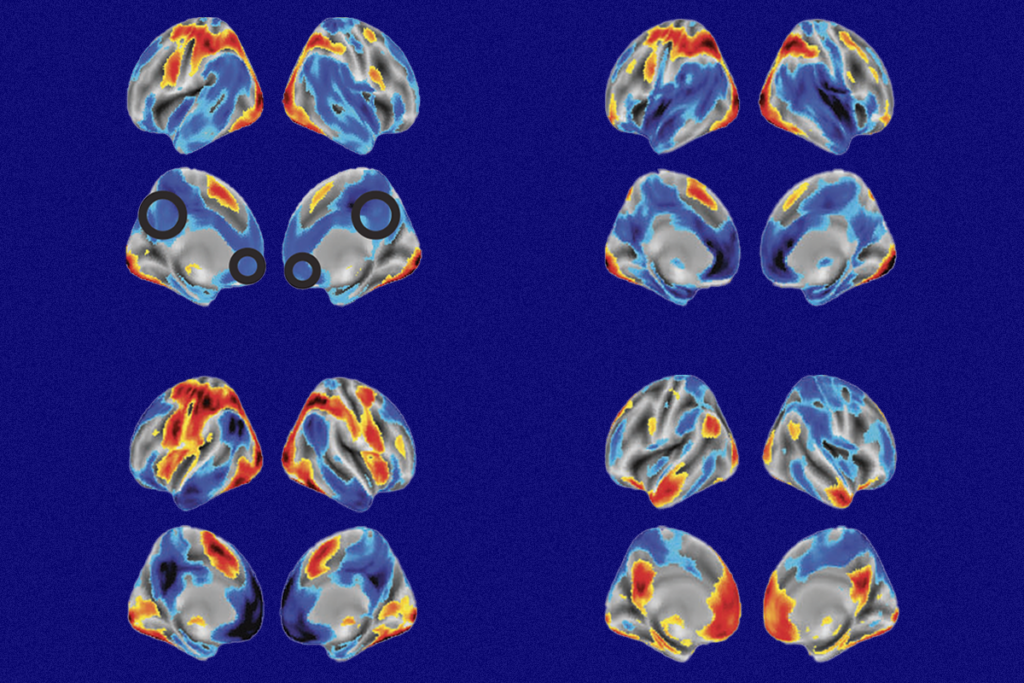
During decision-making, brain shows multiple distinct subtypes of activity
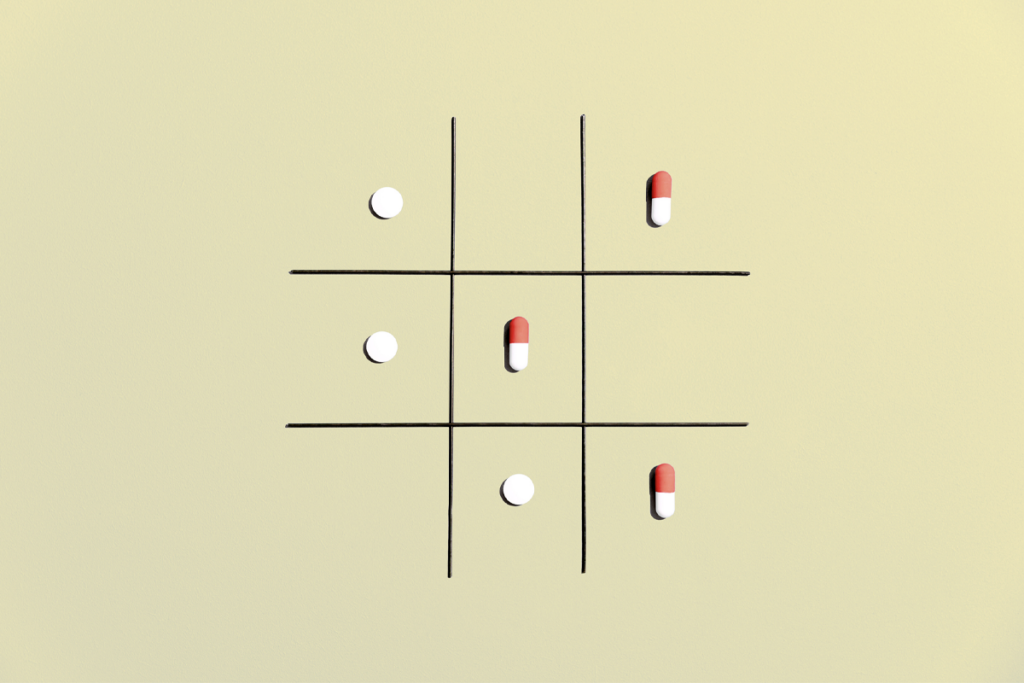
Basic pain research ‘is not working’: Q&A with Steven Prescott and Stéphanie Ratté
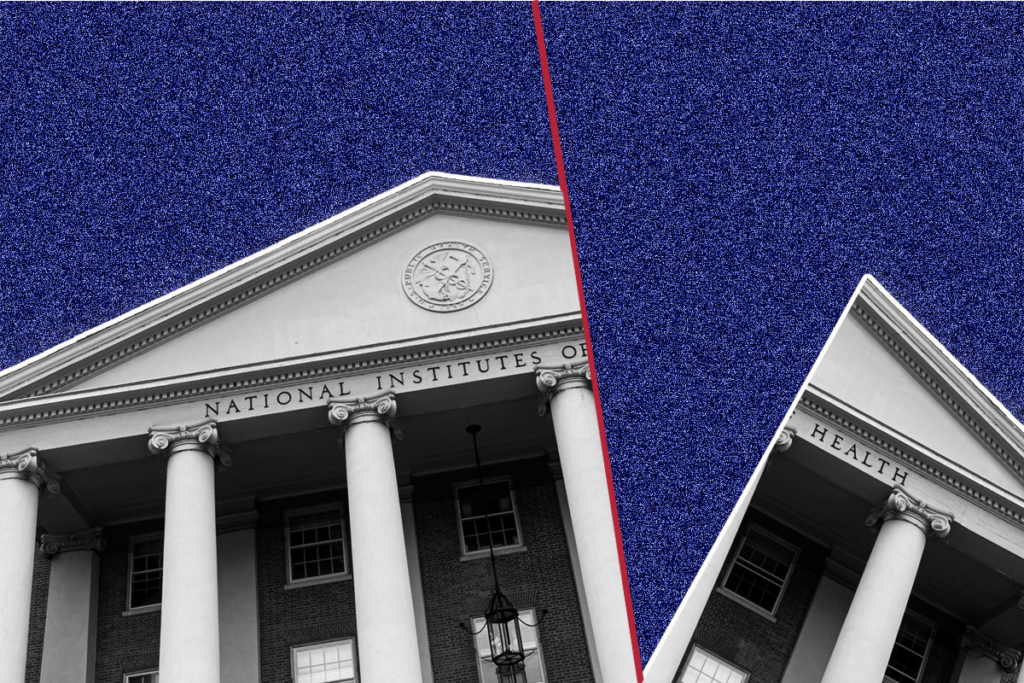