Save your money
A genetic test purported to detect children with autism may instead be highlighting ancestry differences, says T. Grant Belgard.
Despite presenting early signs, most children with autism don’t get diagnosed until after age 4. This is unfortunate, as intensive early behavioral interventions change the prognosis for many.
A biomarker capable of robustly identifying toddlers or infants at high risk of developing autism would revolutionize clinical practice by enabling physicians to detect the disorder much earlier1.
Last year, researchers from the University of Melbourne in Australia reported an algorithm that purports to accurately predict one’s risk of developing autism using just the common variation in one’s genome2 (i.e., the kind of genetic information assessed by companies such as 23andMe for as little as $99).
While this was exciting, many researchers were skeptical. In a new analysis published 2 April in Molecular Psychiatry, my colleagues and I show that the results are likely to be an experimental artifact3.
For years, many tried without success to develop such an algorithm. If this new algorithm were real, it would mean that a lot of people missed something big.
In addition, a clinically useful genetic test like this one, trained using merely hundreds of individuals, is inconsistent with what we know about autism genetics.
Cumulatively, common variation does indeed confer a considerable proportion of autism risk4. However, we don’t know the identity of the vast majority of common variants, and that will be extremely difficult to resolve even with sample sizes much larger than what we currently have. In other words, it’s like looking for a needle in a haystack.
Pathway analysis:
In their study, the researchers attempted to get around this problem by using ‘pathway analysis’ — considering groups of functionally related genes together — but we are unconvinced by its implementation. One particular cause for concern with this analysis is the fact that the researchers heavily weighted some common variants, even though we know that any single common variant contributes very little to autism risk.
Finally, the methods used to design the test are problematic. The approach used for the genetic analysis is one most geneticists don’t use because it is susceptible to bias. The sample sizes are unusually small, and no statistical method can compensate for an inadequate dataset. Critically, the people with and presumably without autism in the study were recruited in different ways and for different reasons.
When we examined the strongest signals in the data, we noted some trends that confirmed our initial suspicions3. (The full algorithm — or classifier — they used to analyze the genetic variants is proprietary and was not made available to us, so we were limited to the strongest signals in the data.)
The frequencies of these genetic variants do not differ between people with and without autism in a new dataset, but they markedly differ between Eastern and Western Europeans. Likewise, when we reconstructed the algorithm using these strongest signals and applied it to a new group of people with autism and a group without the disorder, it classified about 30 percent of both the control group and the autism group as having autism.
When we analyzed a group of Finnish people from a study entirely unrelated to autism, the algorithm likewise predicted that 30 percent have autism. In contrast, it predicted far fewer Western Europeans to have the disorder.
Clearly the classifier did not work as advertised. Why not?
In the original study, the participants with autism were a diverse group of European Americans in the Autism Genetic Resource Exchange, and the controls were descendants of Northern and Western Europeans, specifically recruited for a study of genetic differences among populations.
Likewise, the independent validation of the classifier used another diverse group of European Americans as cases (this time from the Simons Simplex Collection, funded by SFARI.org’s parent organization) and a British birth cohort as controls. These East-West genetic differences confounded the algorithm not only in its construction, but also in its validation.
Of course, this is one of several genetic tests being developed or offered for autism. Until the tests are made fully available to the research community for close scrutiny and independent replication, my advice is to save your money.
T. Grant Belgard is a postdoctoral researcher in Daniel Geschwind’s lab at the University of California, Los Angeles.
References:
1. Walsh P. et al. Nat. Rev. Neurosci. 12, 603-612 (2011) PubMed
2. Skafidas E. et al. Mol. Psychiatry Epub ahead of print (2012) PubMed
3. Belgard T.G. et al. Mol. Psychiatry Epub ahead of print (2013) PubMed
4. Stein J.L. et al. Neuron 77, 209-211 (2013) PubMed
Recommended reading
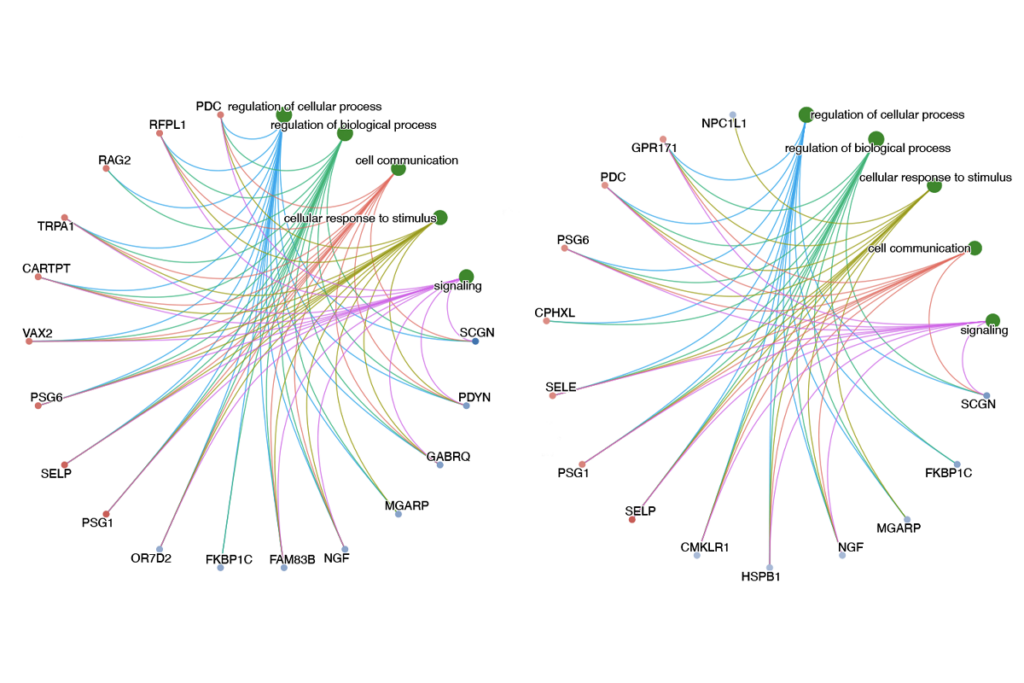
New tool may help untangle downstream effects of autism-linked genes
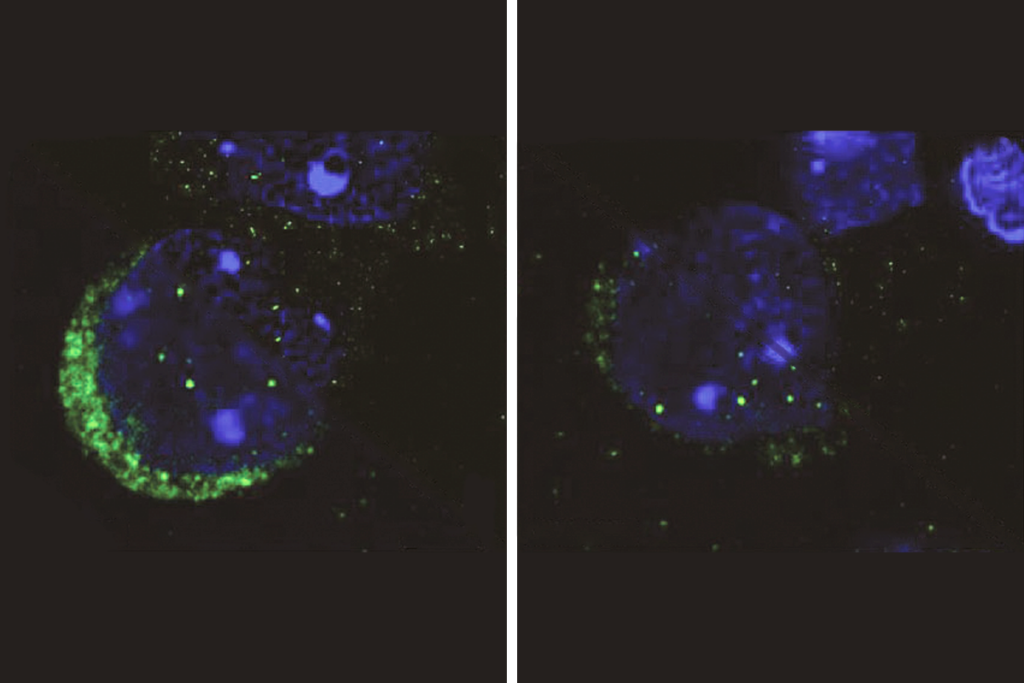
NIH neurodevelopmental assessment system now available as iPad app
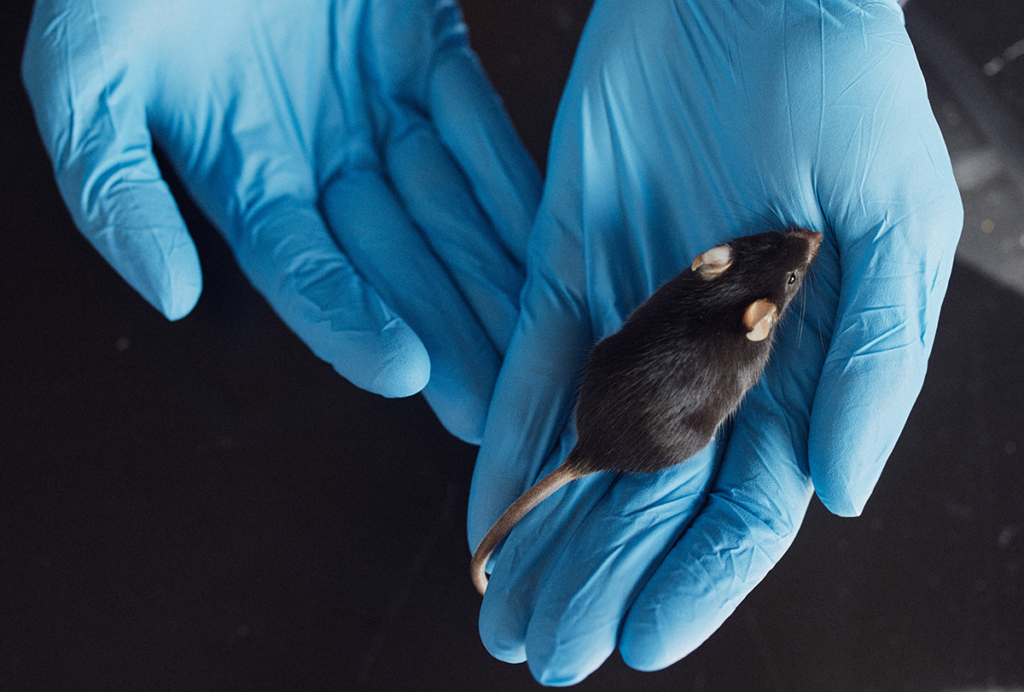
Molecular changes after MECP2 loss may drive Rett syndrome traits
Explore more from The Transmitter
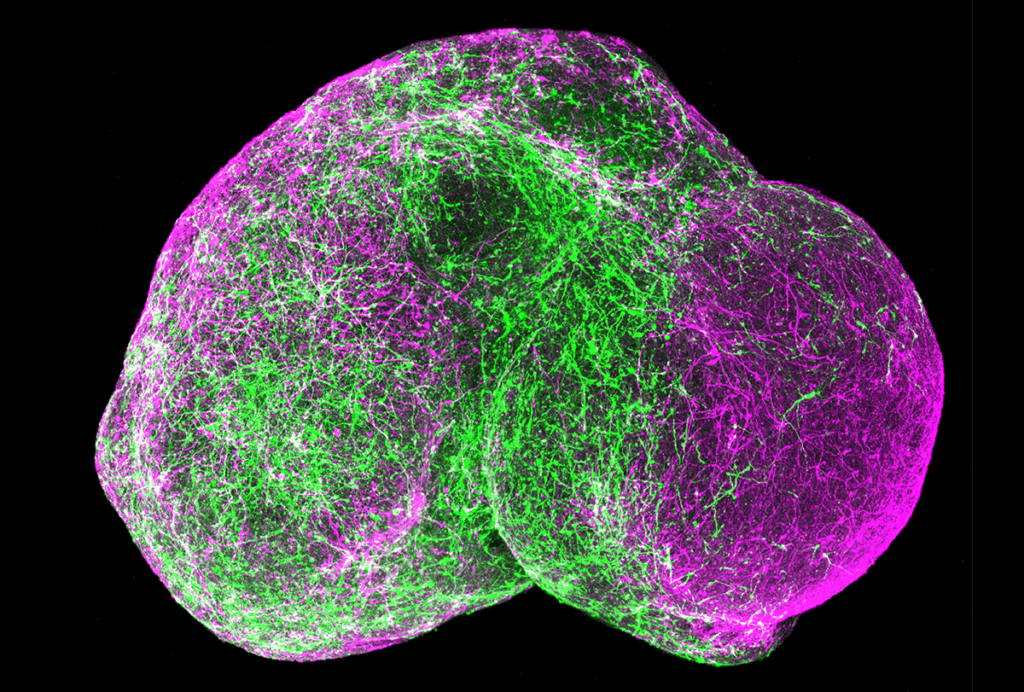
Organoids and assembloids offer a new window into human brain
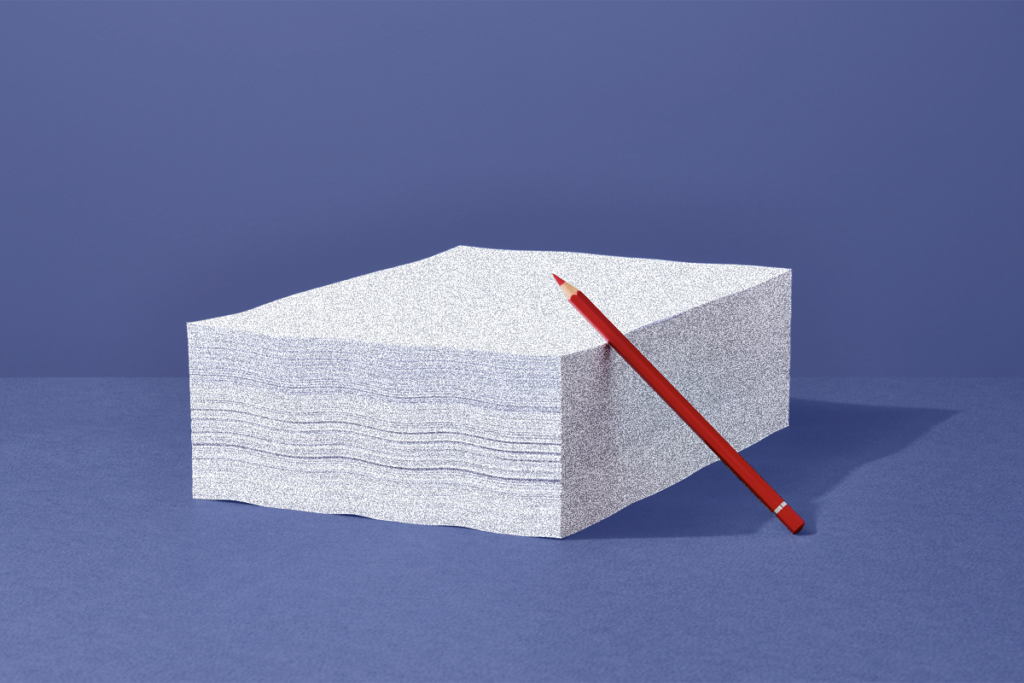
Who funds your basic neuroscience research? Help The Transmitter compile a list of funding sources
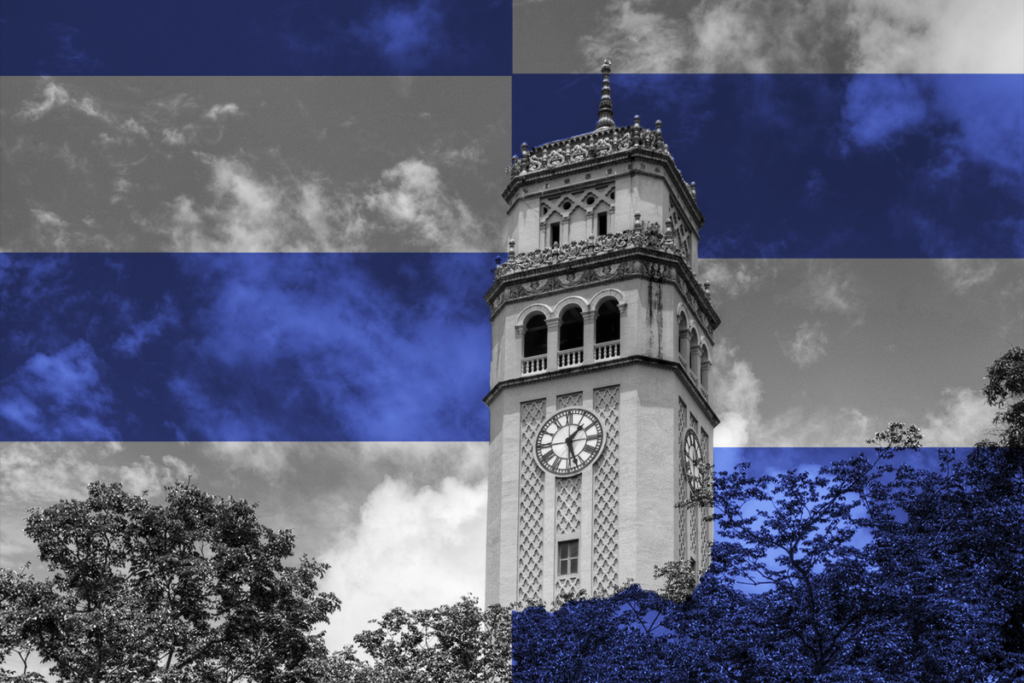