Heeding head motion’s effects
Even small differences in head motion between groups can substantially increase group differences in brain imaging scans. This underscores the importance of accounting for head motion in any study in which one population is likely to move more than another, says Kami Koldewyn.
Almost any task, from planning the simplest motor movement to solving the most complex math equation, involves coordination across the brain. Thus, connectivity between regions of the brain is considered critical to many cognitive and social skills.
Connectivity has become particularly interesting in autism research because changes in the brains of those with autism spectrum disorder could change how the brain integrates information across distant areas. Changes in information integration could in turn help explain the cognitive profile of those with the disorder.
One way to investigate connectivity is to look at how brain regions are connected structurally, using diffusion-weighted magnetic resonance imaging (DW-MRI). DW-MRI uses information about the speed and direction of water diffusion in the brain to infer information about the orientation and structure of the white-matter tracts that connect brain regions to each other.
In a study published 21 November in NeuroImage, my colleagues and I explored the effects of differences in head motion between children with autism and typically developing controls on DW-MRI scans. We found that even small increases in the difference in head motion between groups substantially increases group differences in the scans.
Diffusion tensor imaging findings in people with autism or other clinical disorders are particularly vulnerable to the effects of artifacts for two reasons. First, group differences are typically very small. Second, head motion during DW-MRI scanning leads to a decrease in image intensity, changing the very phenomenon that produces the DW-MRI contrast.
Scarily, this means that any difference between groups in how much they move inside the scanner could lead directly to between-group differences in DW-MRI measures.
Movers, shakers:
Interestingly, the between-group DW-MRI differences hold true not only when comparing autism and typical groups but also when both groups include only typically developing children. Indeed, we found that head-motion differences between groups also induce differences in DW-MRI measures even when the children in the two groups are the very same children scanned twice.
Our results underscore the importance of accounting for head motion in any study in which one population is likely to move more than another — not only in imaging studies investigating autism, but also those looking at other clinical populations, or at change across development.
There are several implications for autism research. First, we must measure and pay attention to motion in DW-MRI scans, especially when making group comparisons. Currently, researchers often do not quantify or report head motion measures in these studies. This has to change if we are to have any hope of finding the ground truth about structural brain differences in autism.
Second, our results demonstrate that currently available analytical tools cannot fully mitigate the effects of head motion on DW-MRI data. To deal with head-motion differences between groups, more action is needed.
Happily, we found that introducing a composite head-motion measure into our statistical model as a nuisance regressor — that is, a known source of ‘noise’ variability in data — in the group analysis can greatly reduce spurious group differences in DW-MRI measures.
Doing this cannot, however, eliminate false positives completely. If possible, matching groups on head motion after eliminating truly bad data is an even better solution.
Third, our results highlight the importance of looking at the relationship between motion measures and other variables that are of interest before drawing conclusions. In our study, head motion measures correlate with both age and symptom severity in the autism group. Such confounds could spuriously drive (or at least influence) associations between DW-MRI measures and demographic, clinical or behavioral measures.
The above suggestions can go a long way toward eliminating false positives — in this case, spurious group differences. But it is important to recognize that if overall data quality is too low, we cannot eliminate false negatives, meaning that we may fail to find a difference that actually exists between groups. In the end, there is nothing that can be done to save truly bad data.
Even when groups are matched on head motion, noisy data can obscure true differences between groups. Even worse, those individuals with autism who cannot tolerate being scanned cannot contribute to our imaging picture of the disorder, potentially biasing our findings.
Thus, our results also demonstrate the need to continue to develop new methods of DWI-MRI acquisition to create scans that measure and compensate for motion as data is acquired and that gather data more quickly and quietly. It is critical to make such scans widely available to researchers, and make it common practice to use them in clinical and developmental studies.
Most importantly, our results emphasize how crucial it will be for us, as a community, to acknowledge and discuss data quality issues in autism research. Issues of motion artifacts and data quality are relevant not only to DWI-MRI, but also to many other imaging techniques. The more such data quality issues are discussed and debated, the more solutions will be developed, and the closer we can get to finding the answers we all seek.
Kami Koldewyn is a psychology researcher and founder of the Developmental Social Vision Laboratory at Bangor University in the U.K.
Recommended reading
Explore more from The Transmitter
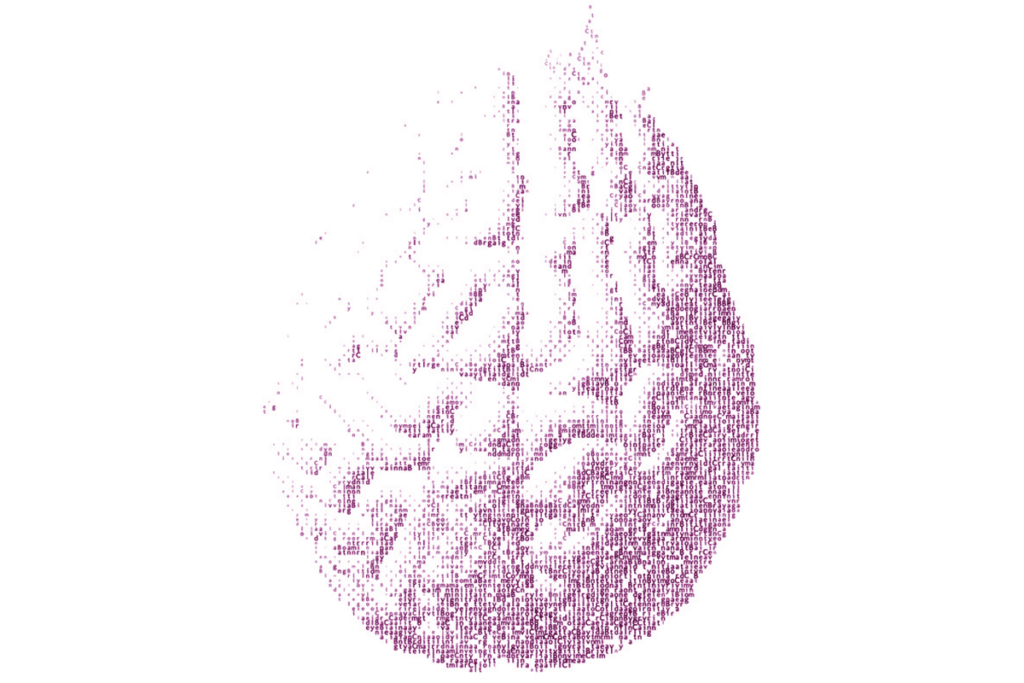
ABCD Study omits gender-identity data from latest release
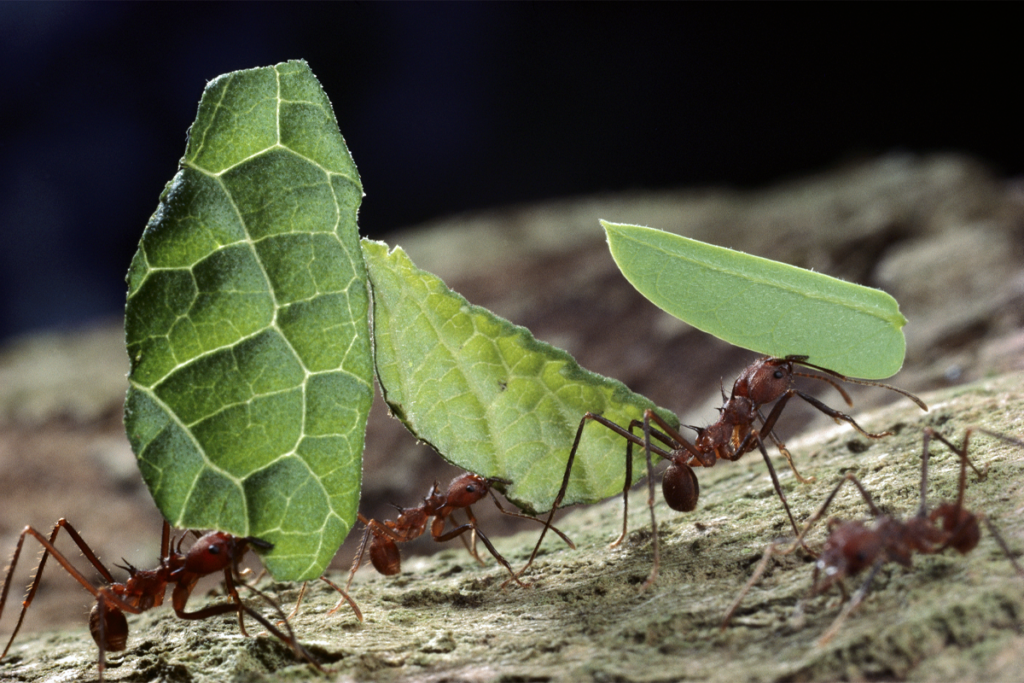
Neuropeptides reprogram social roles in leafcutter ants
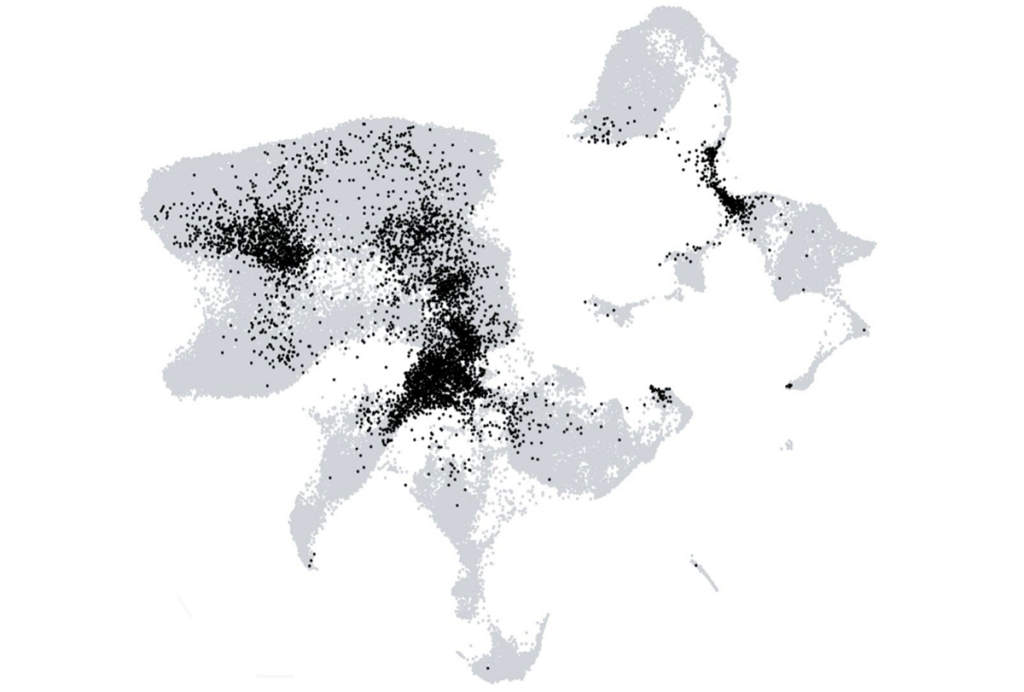