Burning debate: What’s the best way to nab real autism genes?
How to best use a large volume of data to discover new genetic risk factors for autism is a matter of intense debate, particularly in light of historical challenges.
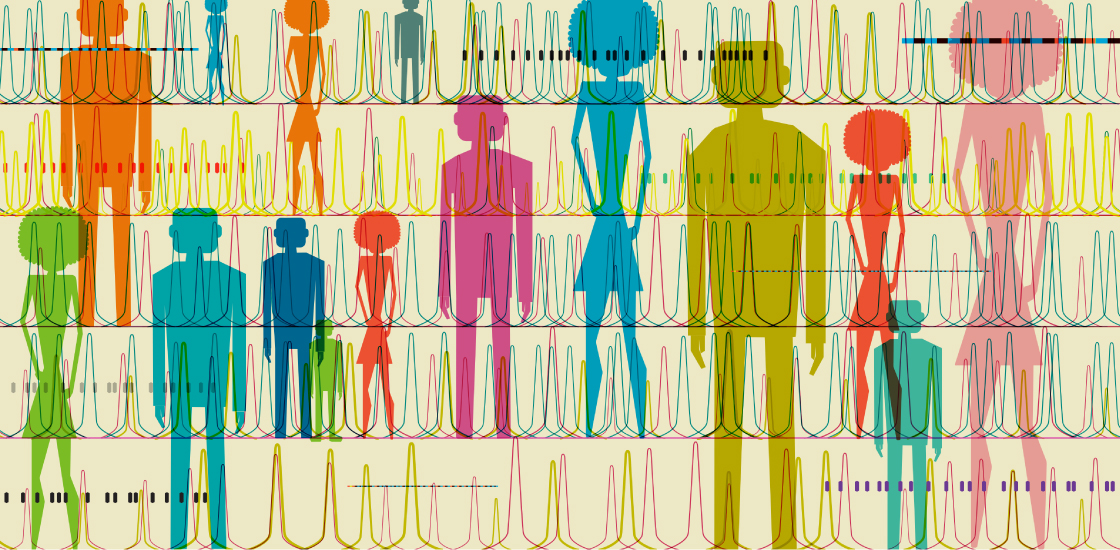
Over the past 10 years researchers have made tremendous progress in understanding the genetic risk factors for autism; however, many genetic factors still remain to be discovered and, importantly, validated. Most of the efforts to find autism risk genes to date have been focused on the 1 percent of the genome that codes for proteins. In the next decade, the field is moving to explore the rest of our ‘genomic iceberg’ — the 99 percent of DNA that does not code for proteins.
Gene jockey
Racing to connect genetics and neuroscience
How we best use this new large volume of data to discover the remaining genetic risk factors for autism is a matter of intense debate, particularly in light of our historical challenges. We still have hundreds of candidate genes that need to be rigorously validated as definitive risk factors. To find noncoding aberrations tied to autism, some scientists say we should comb the genome with no particular genes in mind; others contend that vetting known suspects is the way to go.
My opinion is, if we can remember the lessons from our past, there is room for both approaches.
When I think about new directions for autism genetics, I can’t help recalling my start in this field. It was the dawn of 2004, and I was doing my second graduate program rotation in the laboratory of Matthew State, then an assistant professor of genetics and child psychiatry at Yale University.
At that time, there were two main types of candidate genes for autism: ‘syndromic’ and ‘non-syndromic.’ Syndromic genes underlie related conditions, such as fragile X syndrome and tuberous sclerosis, that have a single genetic cause and recognizable clinical presentation. Large numbers of individuals with these conditions meet the diagnostic criteria for autism. But it isn’t certain that the autism in these individuals is identical to ‘idiopathic autism,’ or autism of unknown cause.
Non-syndromic candidate genes can be split into roughly three classes. The first class encompasses those genes that underlie non-syndromic genetic conditions. These include forms of intellectual disability without a defined clinical presentation; many individuals with this diagnosis also meet the criteria for autism.
The second class, biologic candidates, is made up of genes nominated based on a clinical measurement or someone’s best guess about autism’s biologic mechanisms. The third category, case reports, or so-called ‘N-of-1’ stories, are those in which researchers have identified a single individual with an unusual genetic finding, such as a break in a chromosomal region that disrupts one or more genes.
Combined, the number of autism candidate genes from these three groups grew into the hundreds by the end of my graduate studies.
However, the problem that we faced in the field in the 2000s — and still do today — was in moving past these initial observations to determine which of the candidates are really involved in autism. Many a Ph.D. thesis, postdoctoral fellowship or research grant was spent chasing down a false start or a dead end.
High-tech tools:
In the past few years, we have developed new ways of nominating and validating candidate genes. Although we have made tremendous progress in vetting these genes, the candidates still greatly outnumber the known genes. Because results from genetic tests generally include only the known genes, our quest for them has major clinical implications.
By early 2010, new technologies that allowed massively parallel sequencing of DNA and the selective capture of the protein-coding parts of the genome — the ‘exome’ — were becoming widely available. These technologies let scientists sequence the exomes of individuals with complex disorders at single-base resolution.
We and others adopted these approaches to perform unbiased genome-wide scans for new autism candidate genes. We focused on families that include a single individual with autism to try to identify spontaneous, or de novo, mutations in single genes. Our hypothesis was that these mutations would explain some autism that suddenly appears in families.
These studies identified thousands of new mutations in genes, many of which made sense as candidate risk genes. However, for most genes, the studies identified only a single mutation in a single individual. We also learned that there are likely to be hundreds of autism risk genes operating in this paradigm.
Lacking knowledge of more individuals with those genetic risk factors, we were back in our old predicament: having many interesting candidates but unsure about which ones deserve large investments in time, talent and treasure. New approaches and larger cohorts would be needed to figure out which candidate genes are true autism risk genes as opposed to chance events.
Validating variants:
In 2012, we developed an approach to establish bona fide risk genes for autism. It combined a computational model to estimate the likelihood of random de novo mutations with ultra-low-cost targeted resequencing of 50 to 100 candidate genes. By finding additional mutations in candidate genes, our validation framework enabled statistically rigorous implication of genes for autism risk.
Using this approach, we showed that six genes have more new mutations than expected by chance. Collectively, these genes are disrupted in about 1 percent of people with autism. The most commonly mutated gene, CHD8, has been called the first ‘high-confidence’ risk gene for autism.
The strategy we laid out is simple and robust. It relies on the principle that de novo mutations should be randomly distributed throughout the genome. Given per-gene mutation rates, we can identify genes that have more mutations than expected by chance.
Importantly, this approach provides a confidence level that de novo mutations in a gene are associated with autism. Researchers designing a study on autism risk genes can decide if they want the strength of evidence to be 1 in 1,000 or 1 in 1,000,000, depending on the resources available. This and similar approaches have now been repeatedly used to validate many candidate genes for autism and other developmental conditions.
New methods are integrating genetic variants other than de novo mutations to bolster the case for a candidate gene. For example, the ‘transmission and de novo association method’ (TADA) can layer different sets of genetic data to boost the chances of finding several different types of genetic hits on the same gene. TADA has identified 65 candidate genes as moderate- to high-confidence autism risk genes. With this approach, too, researchers can set their appetite for risk for follow-up studies.
Bright future:
A few new efforts — including SPARK, for which I am a site investigator — are under way to partner researchers with tens of thousands of families. These efforts offer us the power to identify the most common candidate autism risk genes in the next three years. The strength of evidence for several hundred of these genes may be strong enough to move them from the candidate gene column to bona fide autism risk genes using methods like those I described.
This will be a tremendous accomplishment. But still, we will be left with hundreds of candidate genes in need of vetting. We may be able to confirm many of these genes using functional approaches — for instance, using so-called mini-brains, or ‘organoids,’ that allow the mimicking of human brain development in a culture dish — as they will be too rare individually to be validated based on genetics alone. Importantly, we need to continue to develop statistical approaches that integrate the contributions of all classes of genetic variation and that work for families with one or more individuals who have autism.
The field is moving forward with whole-genome studies involving thousands of families to discover genetic risk factors within DNA that does not code for protein.
And now a debate is raging among geneticists. Some scientists believe we should be integrating all we have learned from exome studies to identify noncoding regulators of candidate and bona fide risk genes. For example, we might aim our search at certain regions of the genome known to be actively expressed during brain development.
If we adopt this approach, we face several questions: Which genes and regions should be our focus, and at which times in development? Where in the brain should we be examining these genetic regulators? Importantly, do we know enough yet to answer these questions?
Others, the ‘agnostics,’ remember the many dead ends from the past resulting from hubris. They argue that rigorous and unbiased methods are needed to correct for chance findings from the vast number of results from an entire genome. It may take tens of thousands of genomes to unravel these complexities.
Which of these two approaches is best depends on the question we are asking.
If we are trying to identify novel regulators of a known autism risk gene, restricting our analysis to certain suspect regions makes sense. However, we should be prepared to demonstrate that changes to these regulators are in fact meaningful. To do so, we will need to show that a genetic variant can alter the function of the genome in a biologically relevant context.
If we are trying to identify novel classes of risk factors in an unbiased manner, we should think critically about which independent comparisons we are making between genetic regions and continue to develop methods to control for the large number of statistical tests being performed.
We should also remember that for the next few years, studies using these approaches are likely to be underpowered — meaning that the probability of detecting all but the strongest genetic effects will be low. We should try not to lose heart over negative results. And, equally important, we should avoid being overly optimistic about positive, but still exploratory, findings.
If we can remember our past, the future for autism genetics will be a bright one.
Recommended reading
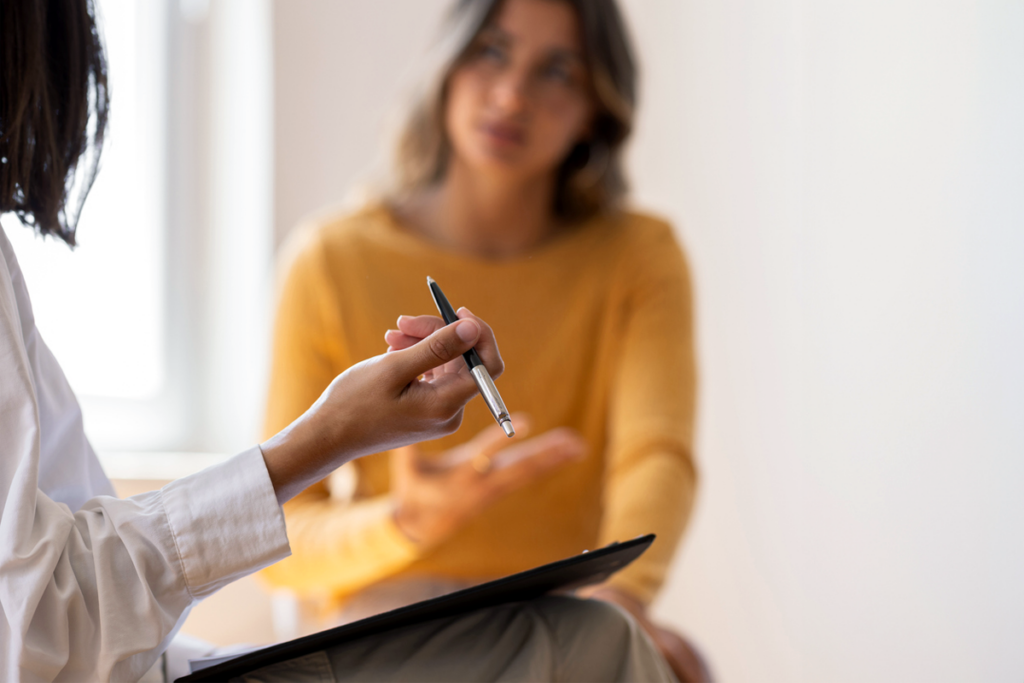
Autism prevalence increasing in children, adults, according to electronic medical records
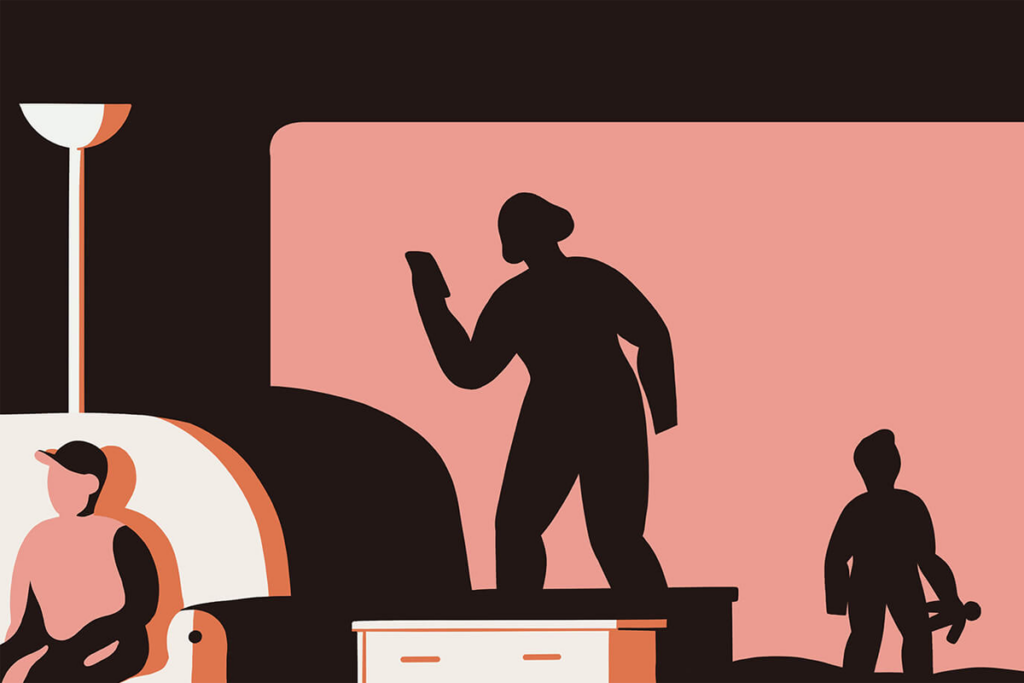
Executive function; screen time; Rett syndrome therapy
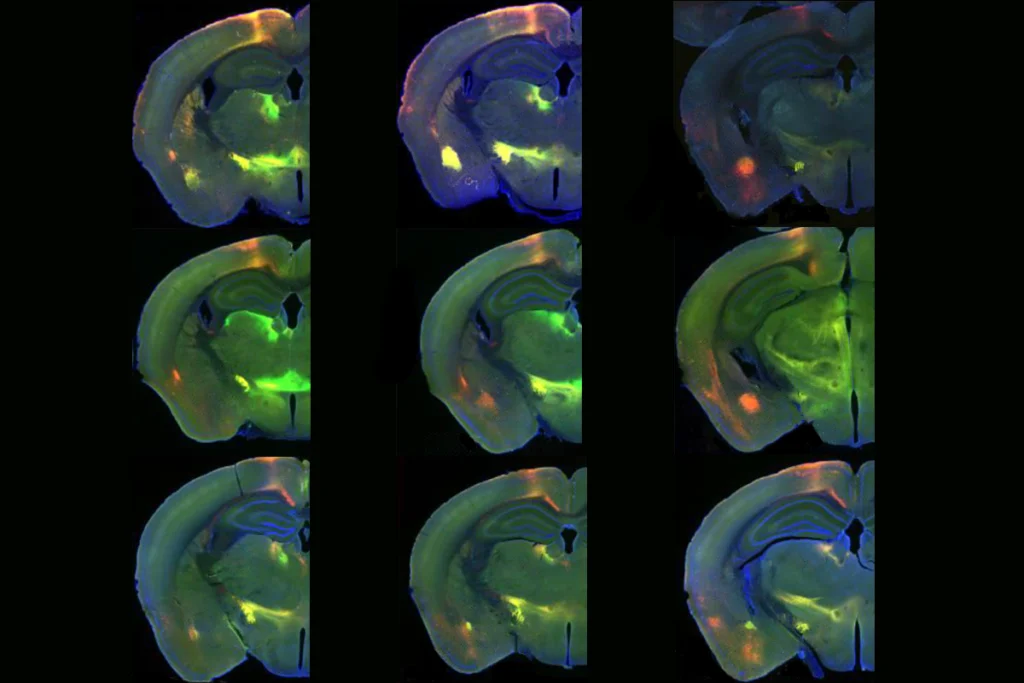
Newfound gene network controls long-range connections between emotional, cognitive brain areas
Explore more from The Transmitter
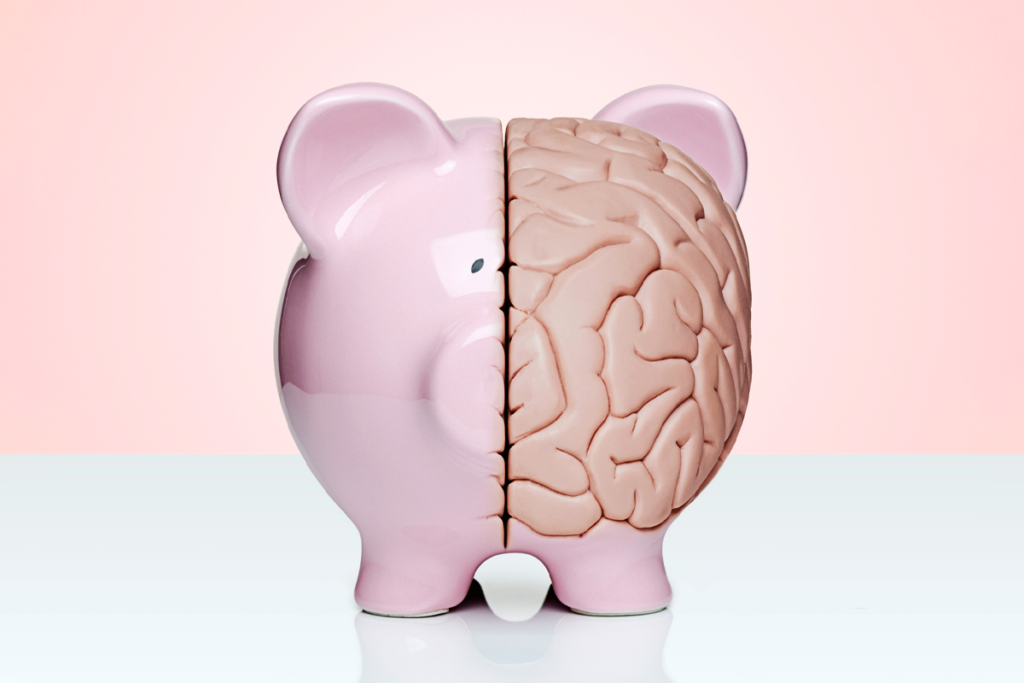
Neuroscientists reeling from past cuts advocate for more BRAIN Initiative funding
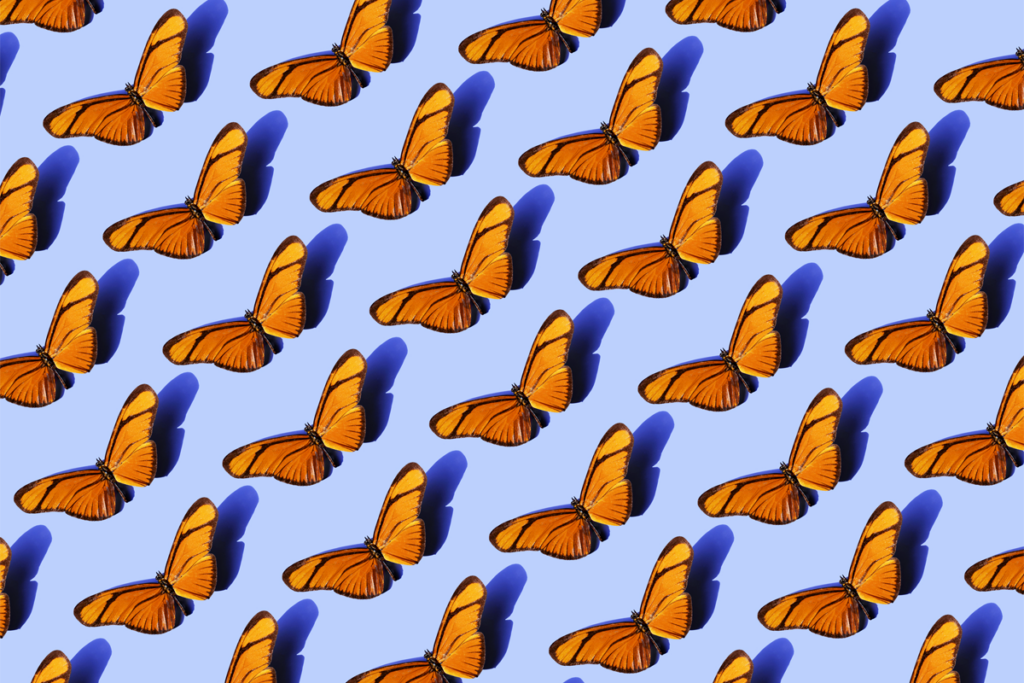
‘Huge influx’ of neuroscientists migrates to Bluesky
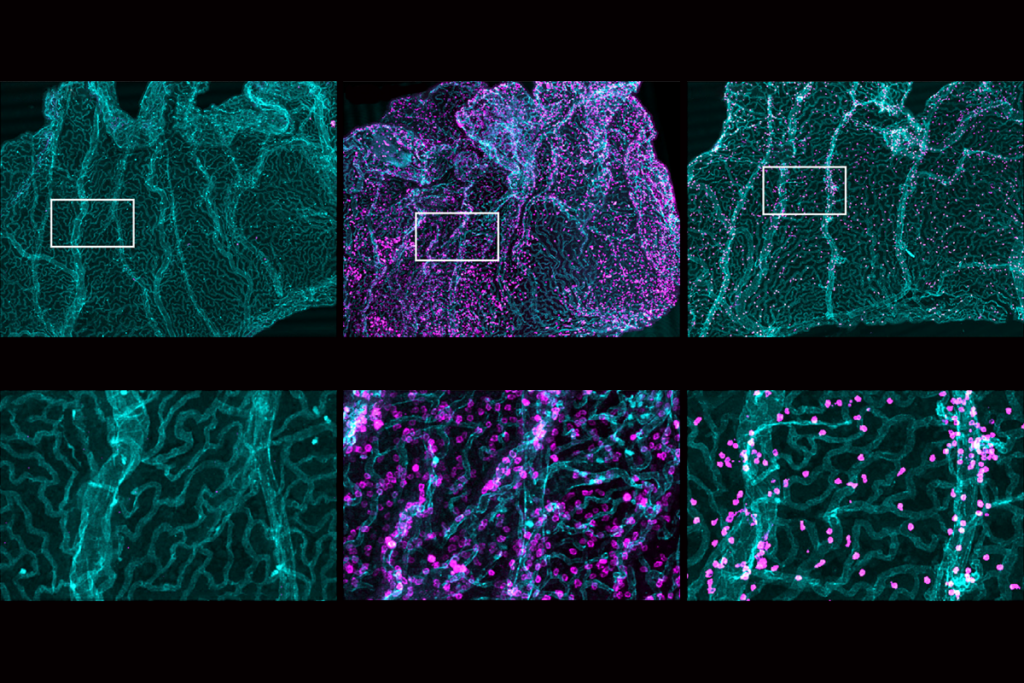