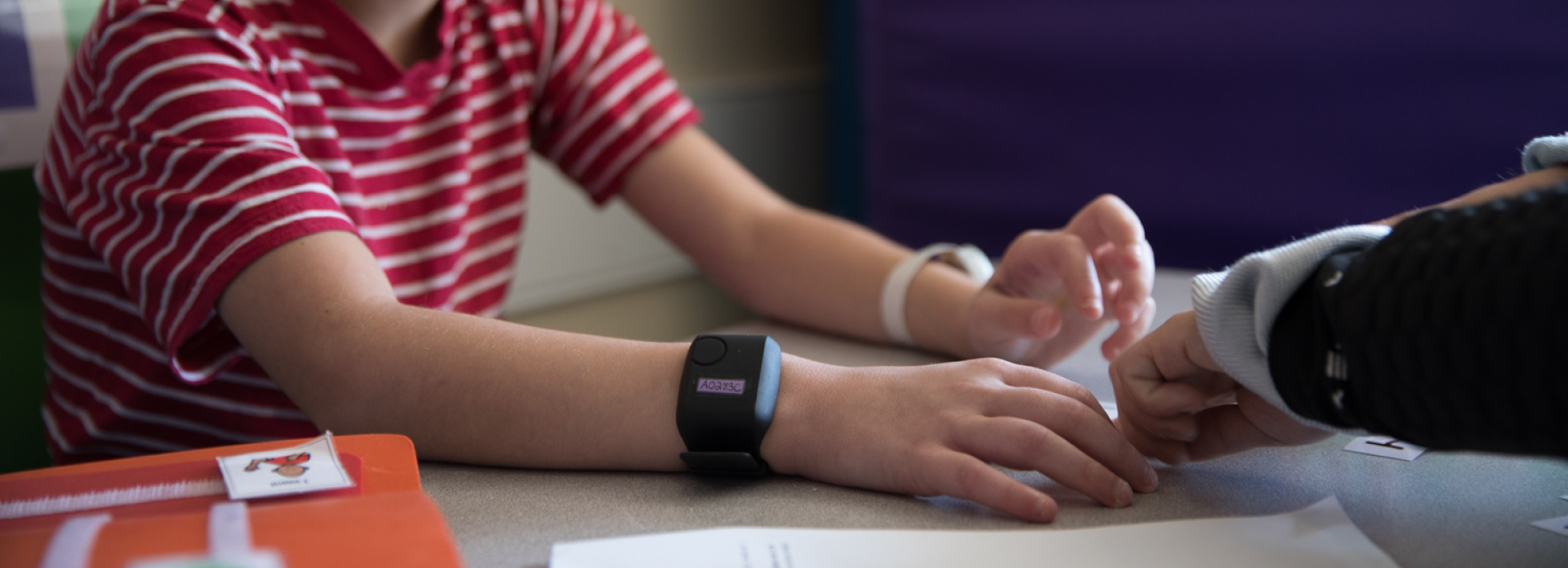
Autism, through the eyes of a computer
Autism traits are recorded predominantly by clinicians with their own subjective biases. Can technology do it better?
I
t’s a bright, warm Friday afternoon in June at Spring Harbor Academy, a private school in Westbrook, Maine. A faint aroma of cilantro and lime hangs in the air, traces of the guacamole the students made — and promptly devoured — half an hour ago in a cooking class. Sunlight streams in through a window, and three students now sit at separate tables, peacefully absorbed in their tablet computers. Accompanying each adolescent boy in this classroom is a teaching assistant wearing thick arm pads and a helmet with a face shield.The academy’s students are also residents of Spring Harbor Hospital, a facility with a dedicated unit for autistic children. However relaxed they appear now, they are prone to kicking, biting, scratching, pushing and hitting.
The boys take turns wearing a special device: a wristband with sensors that track their heart rate, sweat and movement. This device may help doctors predict the boys’ outbursts before they begin, the subject of an ongoing study at the hospital.
In one corner of the classroom, rocking back and forth, sits Julio, 16. Julio was not always aggressive. He was diagnosed with autism at age 3 and was docile until about a year ago, when he became increasingly moody and began lashing out, says his mother, Desirae Brown.
At first, he hit only himself. But in April, he chased his mother into the house with a knife. When she shielded herself from him on the other side of the front door, he smashed one of the door’s glass panes. Terrified, she instructed her daughter to call the police and held the door shut until they arrived and restrained him.
The incident pushed Brown to seek a spot for Julio at the hospital, a five-hour drive from his hometown of Caribou, Maine. “It’s just me and his sister there [at home],” she says, so she realized: “We have got to get some help.” When she found out about the study to predict aggression, she immediately signed Julio up.
The project is still in an early phase; the researchers have analyzed data from only 20 children so far. But in June, they reported that an algorithm trained on the data predicts aggression one minute in advance with 71 percent accuracy. “Obviously we want [the accuracy] to be as high as possible, but we were pretty happy,” says co-lead investigator Matthew Siegel, director of the Developmental Disorders Program at the hospital. Most aggressive episodes occur without warning, he says, so any advance notice means “you’re ahead of the game.”
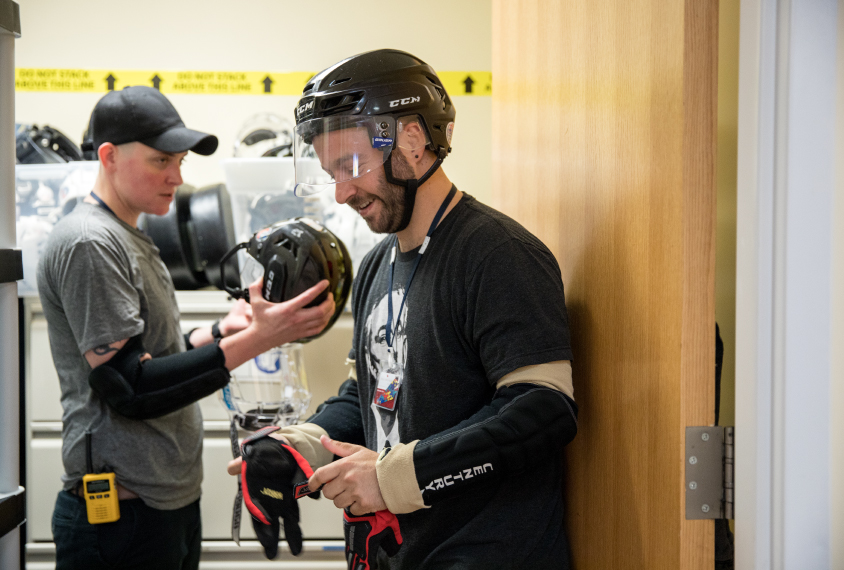
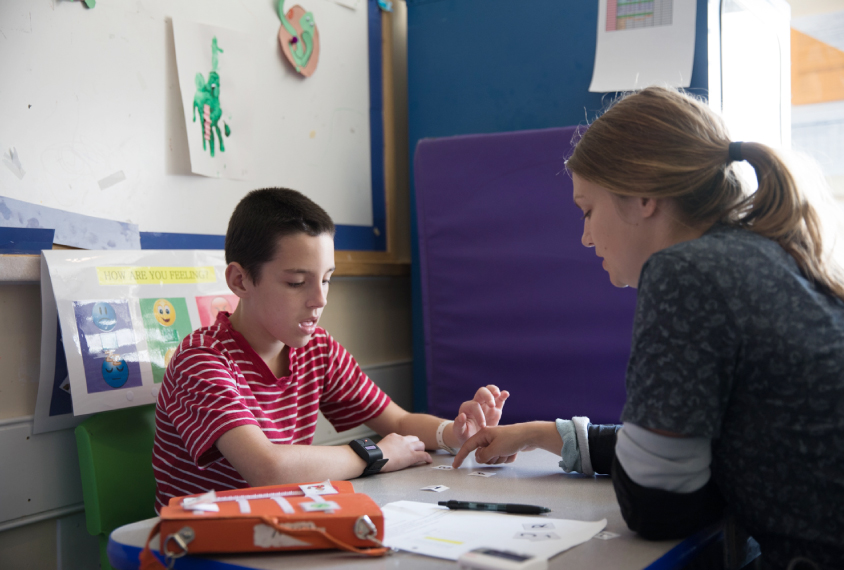
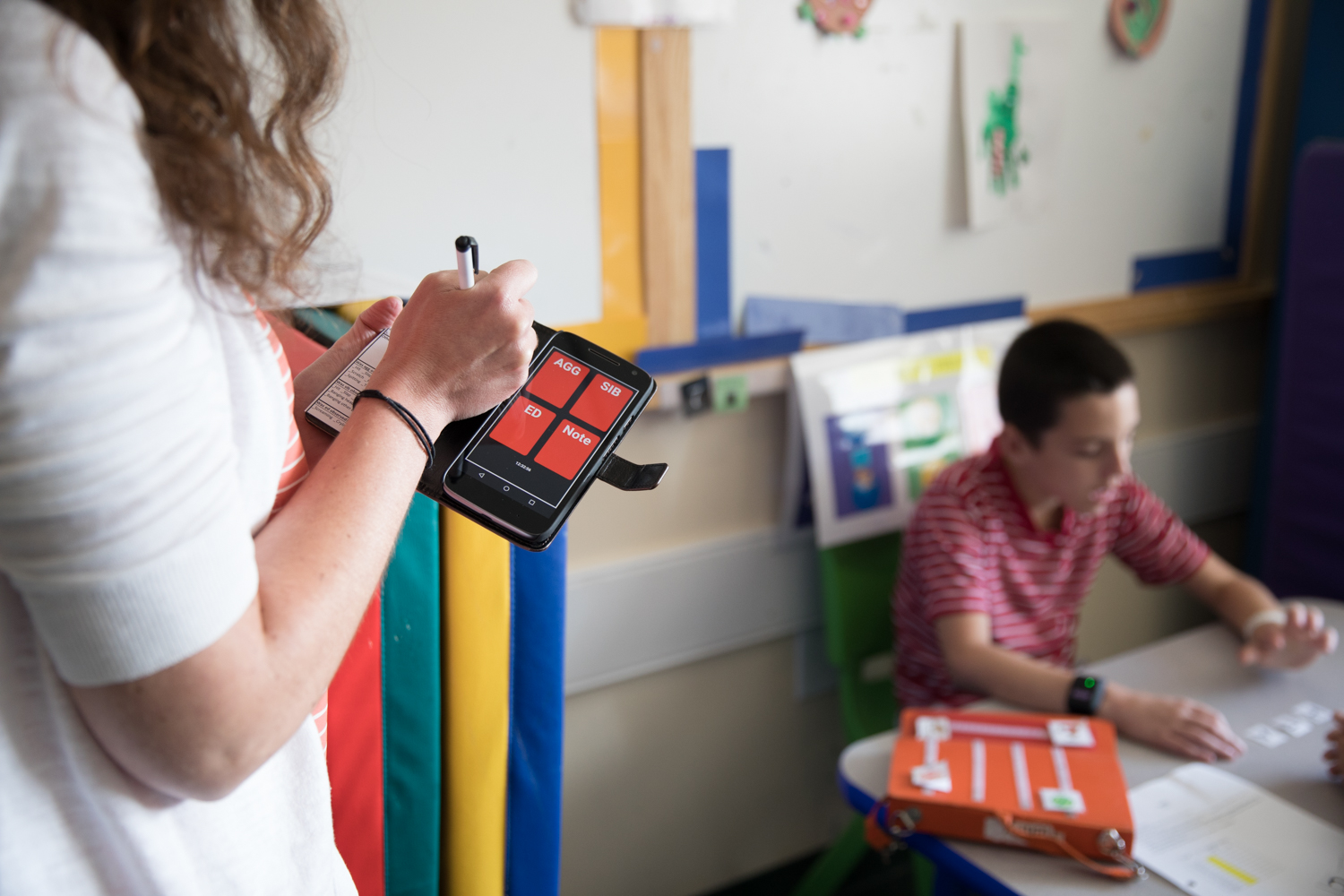
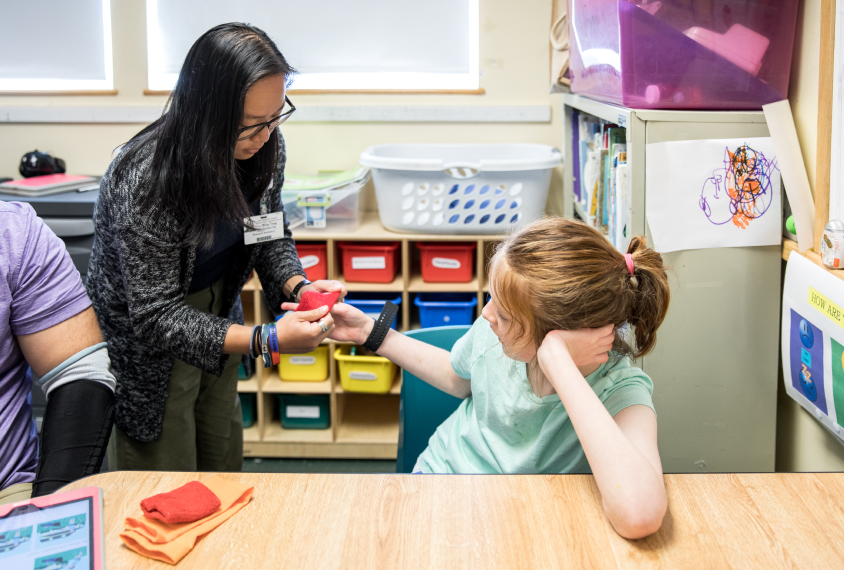
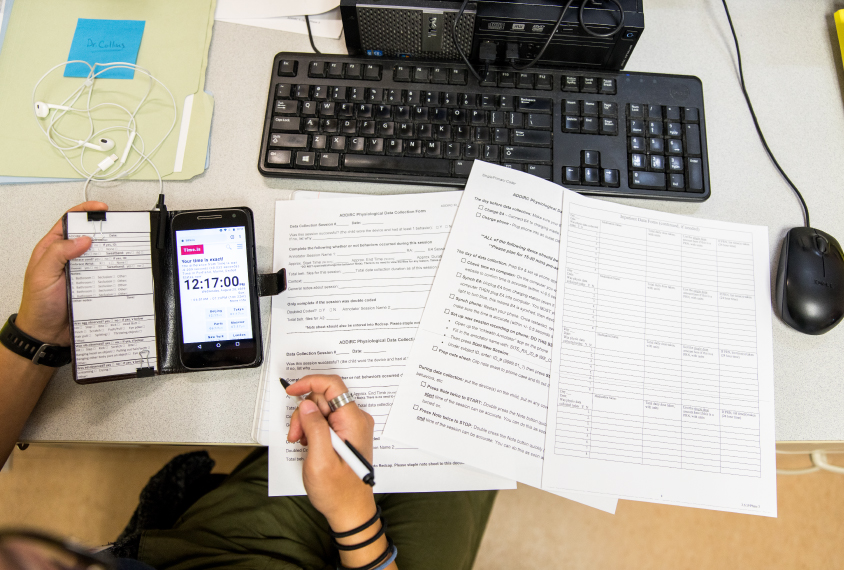
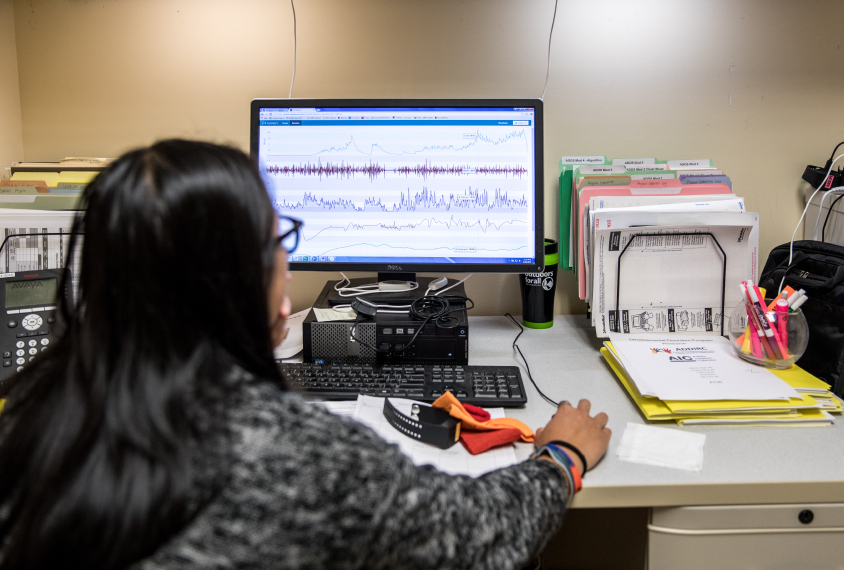
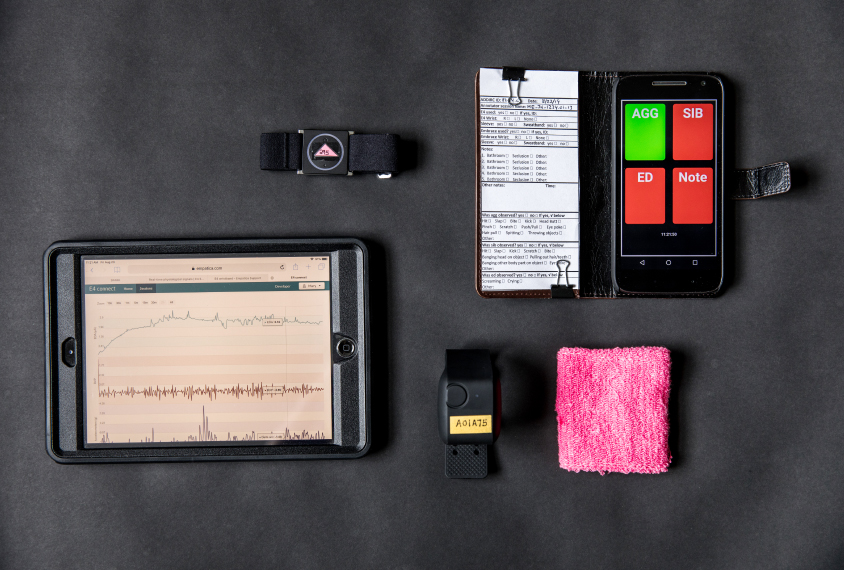
Suiting up: Teachers at Spring Harbor Academy put on protective gear before entering the classroom.
Tracking device: Autistic children at the academy take turns wearing a wristband that tracks their heart rate, sweat and movement.
On the record: A researcher logs each child’s behaviors in a smartphone app.
Safeguarding data: The children wear an elastic band around the wristband to prevent them from taking it off.
In sync: The clock on the smartphone is synchronized with the one on the wristband, so that data from the two can be compared.
Inspector gadget: A research assistant reviews data collected by the wristband’s sensors.
Technology array: The researchers use two different wristbands, along with a protective elastic band, a smartphone app and software to analyze the data.
Siegel and his colleagues are part of a growing cadre of scientists turning to wearable sensors, microphones, cameras and other devices to track autism traits. This approach, called ‘digital phenotyping,’ has gained widespread popularity over the past five years. It might have particular value for autism research: These objective measurements lie in stark contrast to the subjective observations of clinicians or parents that are typically used for diagnosis.
“We as a field have traditionally done deep phenotyping with clinician-administered testing and interviewing,” says Bob Schultz, director of the Center for Autism Research at Children’s Hospital of Philadelphia in Pennsylvania. “But in fact, all of it is opinion. And it’s hard to have a science of opinion.”
Digital phenotyping could improve the rigor of autism research, Schultz and others say, by enabling scientists to collect data from large numbers of people in natural settings such as homes and schools, rather than in clinics and labs. The work is still in its infancy: “There are hundreds, if not thousands, of studies that are going to be needed to understand the accuracy and risks of digital phenotyping,” Schultz says. But that hasn’t stopped researchers from exploring a range of applications — from assessing individual autism traits to tracking how they change with age or in response to treatments.
Some experts caution that these tools can never substitute for the judgment clinicians hone through years of experience — but should instead augment it. “Really what we want is more information that can go to clinicians,” says Catherine Lord, distinguished professor of psychiatry and education at the University of California, Los Angeles. (Lord developed the behavior-based tests that are considered the gold standard for autism diagnosis.) Schultz agrees with this caution but notes that digital phenotyping could shorten clinicians’ workload, enabling them to evaluate more people. “[Magnetic resonance imaging] did not replace the radiologist — we need their judgment,” he says. “But it’s a terrific tool to understand what’s going on in the brain.”
Getting to know you:
T
wo strangers sit across from each other. They kick off a simple conversation, exchanging names and interests. As they chat, they shift their body positions, leaning toward each other, away or to one side. Their facial expressions change, too. All the while, a homemade device sits between them, recording audio and video.This is the setup in Schultz’s lab, where his team is trying to detect aspects of social communication that distinguish autistic people from their typical peers. “We’re looking at how the interactions between two people unfold over time,” Schultz says. “None of our [current] evaluations do that.”
Schultz’s interest in digital phenotyping was borne out of frustration. During field trials of the latest diagnostic criteria for autism, trained clinicians at multiple sites used the criteria to diagnose autism. The results suggested that the experts agree only 69 percent of the time. “That’s not very good,” Schultz says. He decided to try to improve the statistics, giving clinical observations a technological assist.
The data from the cameras in his lab feed into a computer program that tracks 180 facial measures, such as movements of the eye, brow and corners of the mouth. Some of the differences between autistic people and neurotypicals might be so subtle that even a trained eye will not be able to see it, Schultz says. But “digital sensors capture all of it, and they don’t have biases.” The program uses a machine-learning algorithm — meaning it can identify patterns in data and then adapt in response to new data without being programmed. The algorithm scans the camera data looking for synchrony — between the movements of the participants’ faces, for instance, or their gestures.
That kind of synchrony is thought to be crucial for successful social interactions. Schultz has found that a lack of synchrony may offer one way to screen for autism. In a small unpublished analysis presented at a meeting in May, his algorithm could pick out autistic participants from controls in three-minute ‘getting to know you’ conversations with 89 percent accuracy. So far, however, his team has tested the method only on people who already have an autism diagnosis.
The team is also collecting data on other variables, including heart rate, tone of voice, rate and loudness of speech, words used and length of pauses in between. They are also analyzing the symmetry of bodily movements and the ability to imitate another person’s actions.
“[Schultz’] work is phenomenal,” says Geraldine Dawson, distinguished professor of psychiatry and behavioral sciences at Duke University in Durham, North Carolina. “Those kinds of quantitative, objective measures of behavior are essential for eventually being able to monitor change in a clinical trial, or to be able to screen for different conditions.”
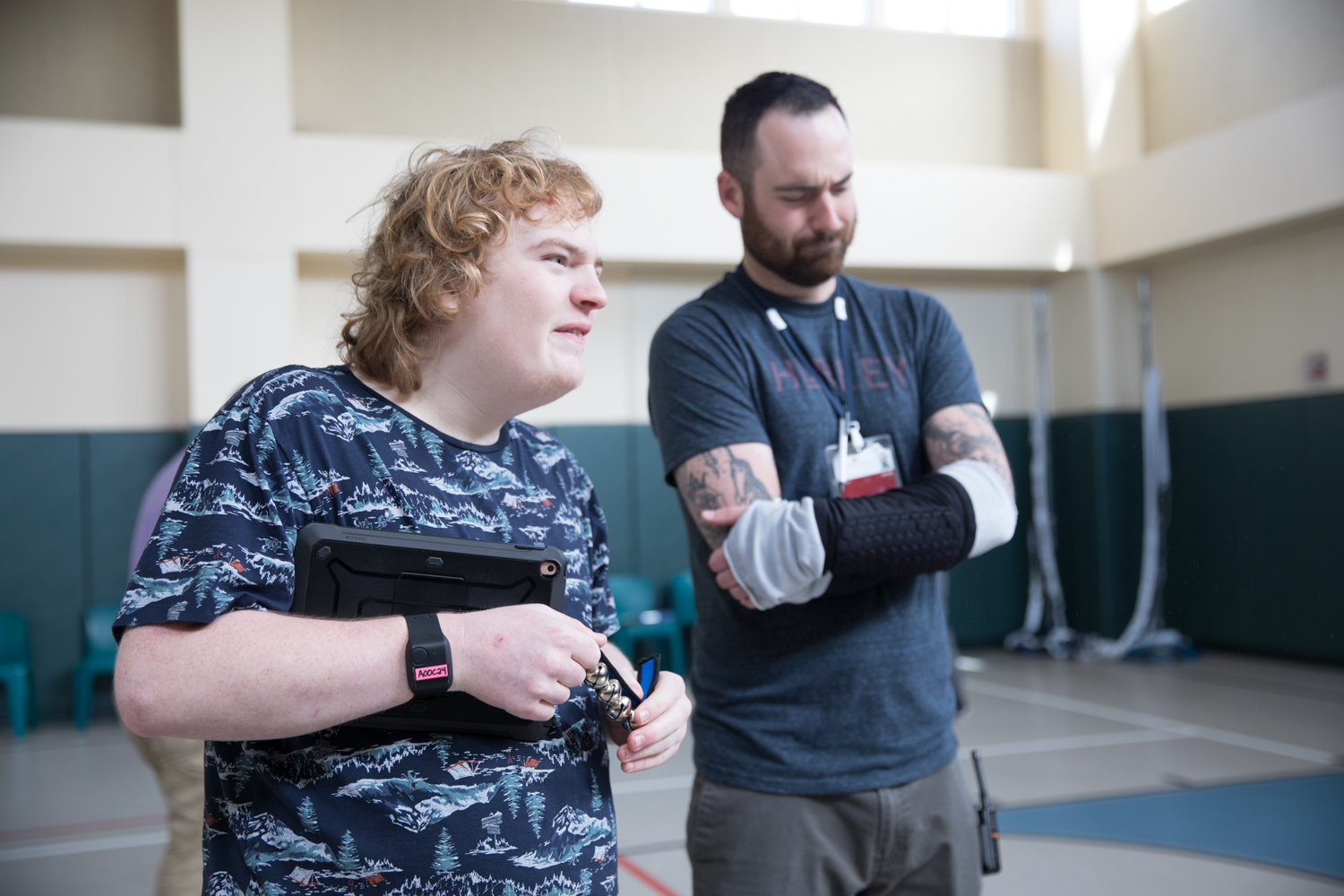
Dawson and her colleagues are also working in this arena: They are trying to get at objective behavioral measures via an app for smartphones and tablets. The app plays a series of short video clips and uses the device’s camera to track a child’s gaze, facial expressions and head movements. It also includes a game to gauge a child’s fine-motor skills; as the child taps the screen to pop bubbles, pressure sensors measure her speed, force and accuracy. The researchers have so far tested the app in 104 toddlers, 22 of whom have autism.
They are also collecting data from thousands of toddlers whose parents fill out a screen for autism, called the Modified Checklist for Autism in Toddlers, during routine clinic visits. Studies suggest that only about half of the 18-month-olds flagged by this screen go on to be diagnosed with autism. Dawson and her colleagues aim to see whether data collected through a version of the app can improve the accuracy of autism screening.
Tools like the app might deliver the greatest benefit to communities that do not have autism specialists. For example, one tablet-based app, called ‘START,’ has shown promise in Delhi, India, where many autistic children are thought to remain undiagnosed. “There are not many specialist clinical psychologists to go around,” says Bhismadev Chakrabarti, a neuroscientist at the University of Reading in the United Kingdom who is testing the app.
Like Dawson’s app, START uses a tablet’s sensors to track a child’s traits and behaviors, such as gaze patterns and motor skills. In a pilot study launched in 2017, non-specialist health workers in Delhi administered the app to 36 children with autism, 33 with intellectual disability and 41 controls, aged 2 to 7 years. The unpublished results, presented at a meeting in May, suggest that performance on the app’s tasks distinguishes the control group from the other two groups of children. Chakrabarti’s team has since translated the app into 11 languages and is testing it in Malawi.
Chakrabarti says the app is intended to identify any developmental areas, not just autism, in which a child might need help. “We really should not worry so much about the diagnostic label,” he says. “If a child has a motor functional problem, then that child needs to see support services which will give him or her motor functional support.”
Trouble ahead:
W
hether digital phenotyping can assist with screening and diagnosis is still an open question—but it might regardless lead to new insights about the condition. “We need to have a very precise measurement of what autism is, and discover the essence of autism,” Schultz says.For Julio and the other boys in Siegel’s study, this might mean that the researchers will be able to better understand features that contribute to aggression. The researchers are assessing the boys’ ability to control their emotions, and plan to test whether accounting for differences in emotion regulation among the study participants improves the accuracy of the predictive algorithm.
Digital phenotyping could also help clinicians track how autism traits change over time.
Lord is part of a team of researchers that is recording audio and video of autistic children’s standardized assessments in order to track their nonverbal communicative behaviors over time. In one setup, a researcher wears a pair of glasses with an outward-facing camera to record a child’s movements and gaze. A second camera in the room captures 3D information, so researchers can track the objects a child looks at in the room. An algorithm analyzes these data to measure how often the child makes eye contact with the researcher. The team is developing another algorithm to detect any instances when the child either initiates or responds to a bid for attention.
“Are there patterns in the child’s gaze and gesture, as well as other types of movements of the head, arms and torso, that might be able to predict not only autism symptoms, but also changes in language?” says co-lead investigator Rebecca Jones, a neuroscientist at Weill Cornell Medicine in New York. “The hope is to use the information to look at change in language over time, as well as overall changes in milestones.”
Digital phenotyping tools might also help clinicians evaluate the effectiveness of experimental autism treatments, which typically fail to pass muster in clinical trials. “We have a very troublesome catch-22 in the autism field,” Dawson says. “Is it because the drug didn’t work? Or is it because our assay wasn’t sensitive enough to pick up change?” Technology that could detect subtle changes associated with treatment would help, she says.
Not that any of this is simple for technology either. Even for an application as straightforward as Siegel’s — predicting aggression — there are a number of significant barriers. For example, the algorithm doesn’t yet make predictions in real time, which it will have to do to prove useful. Another concern is that the algorithm might make an inaccurate prediction — a false positive — or miss an outburst, delivering a false negative and a false sense of safety. “A false positive is not so bad,” Siegel says. You might just prompt someone to take some deep breaths they did not need to. A false negative, however, Siegel says: “That’s not so good, we definitely want to limit that.”
A bigger potential problem is that the pilot data might not hold up to further study. The solution is to make sure the algorithm works just as well with an entirely new set of data. That’s something Siegel and his colleagues are working on, collecting wristband data from more than 200 autistic children at three sites.
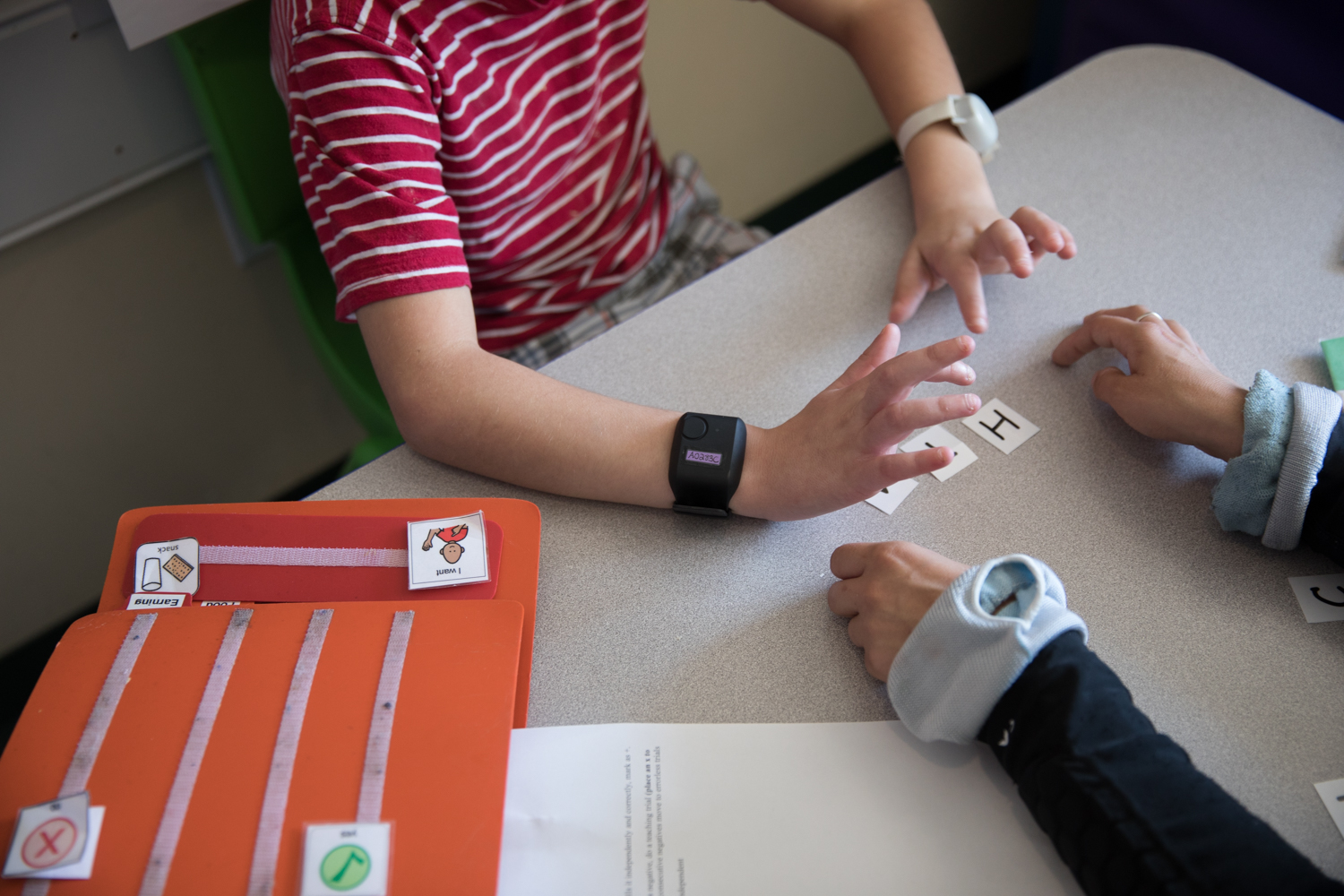
They envision an elaborate system that uses wristband signals to alert wearers — and those around them — when trouble is brewing. For example, it could flash an image of a favorite character to remind the wearer to breathe deeply. Or it might give a caregiver time to move others out of harm’s way and calm the autistic person down by, for example, helping her take a series of deep breaths. “While you’re sitting down and taking deep breaths, it’s hard to do the non-desired behavior,” Siegel says. It also slows the autistic person’s heart rate and helps her to relax.
This sort of warning system might have averted Julio’s attack on his mother in June. “That would be so helpful, letting us know, ‘Hey, he’s getting upset,’ because he’s not able to tell me,” Desirae Brown says.
Julio returned home in mid-July. Since then, he and Brown have been managing his aggression with coping skills they learned at Spring Harbor Hospital. Brown reminds him to take deep breaths when she senses he’s getting agitated, for example. And he earns tokens from his mother for good behavior — maintaining his composure during shopping trips, for example — that he can exchange for time on his computer.
Without a device yet available, though, Brown still keeps a close eye on him. “We live in an area where there’s not a whole lot of help,” she says. The unpredictability of her son’s outbursts means that she no longer takes him out to eat at restaurants. One of her biggest fears is that he’ll have an episode while she’s driving and unable to keep a close eye on him or respond quickly. “I’ve got to constantly watch him,” she says. “The wristband would be able to say, ‘Hey, you might want to pull over.’”
Recommended reading
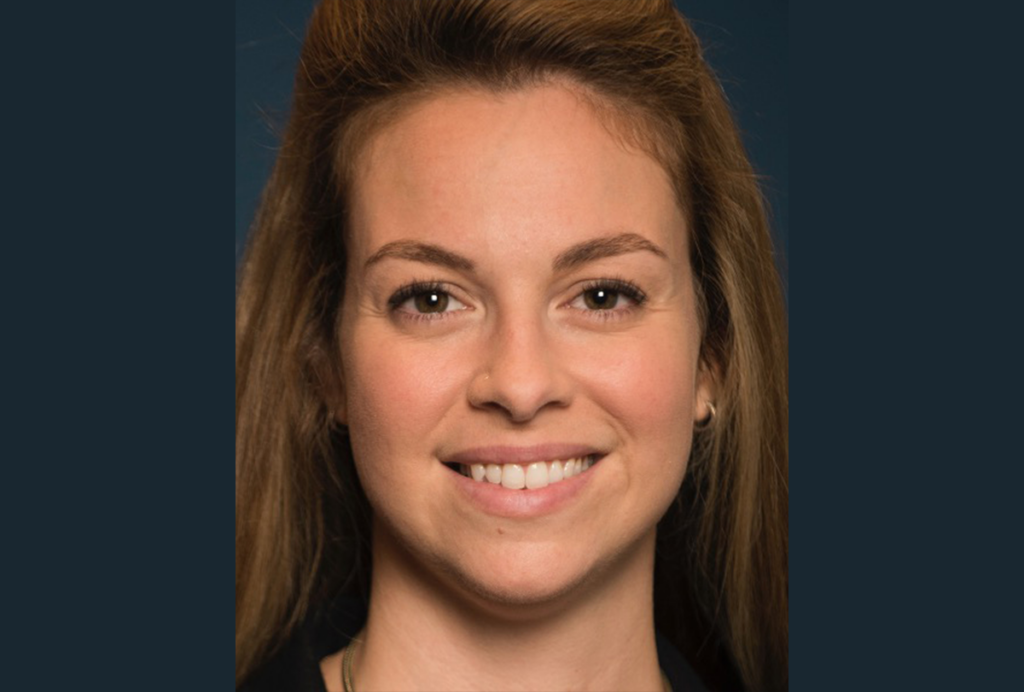
Autism program chief among National Institutes of Health layoffs
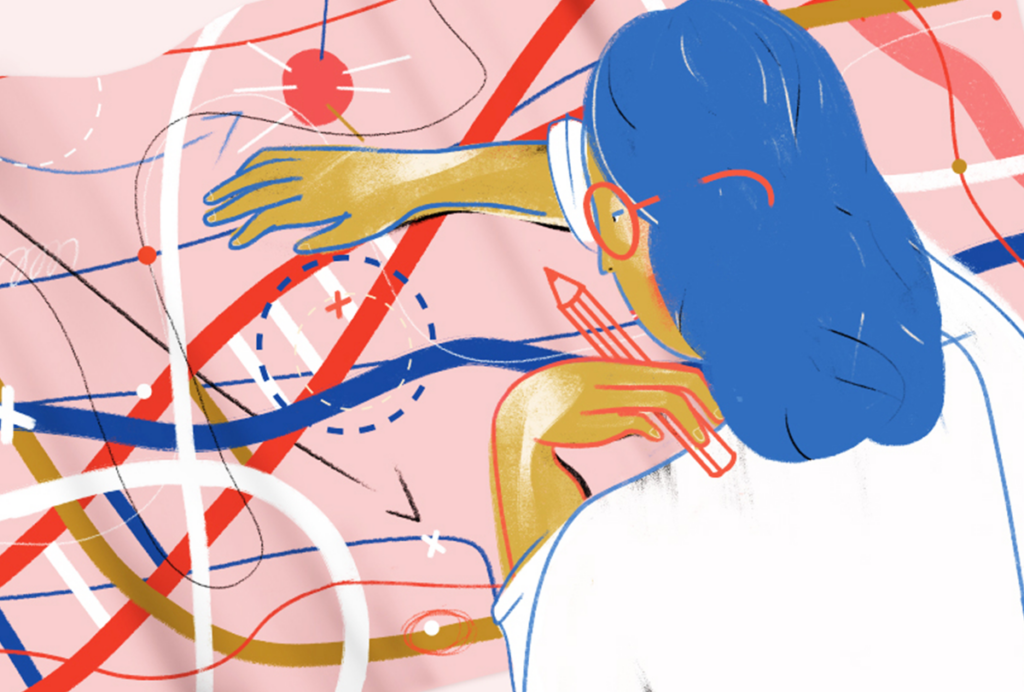
Familiar autism-linked genes emerge from first analysis of Latin American cohort
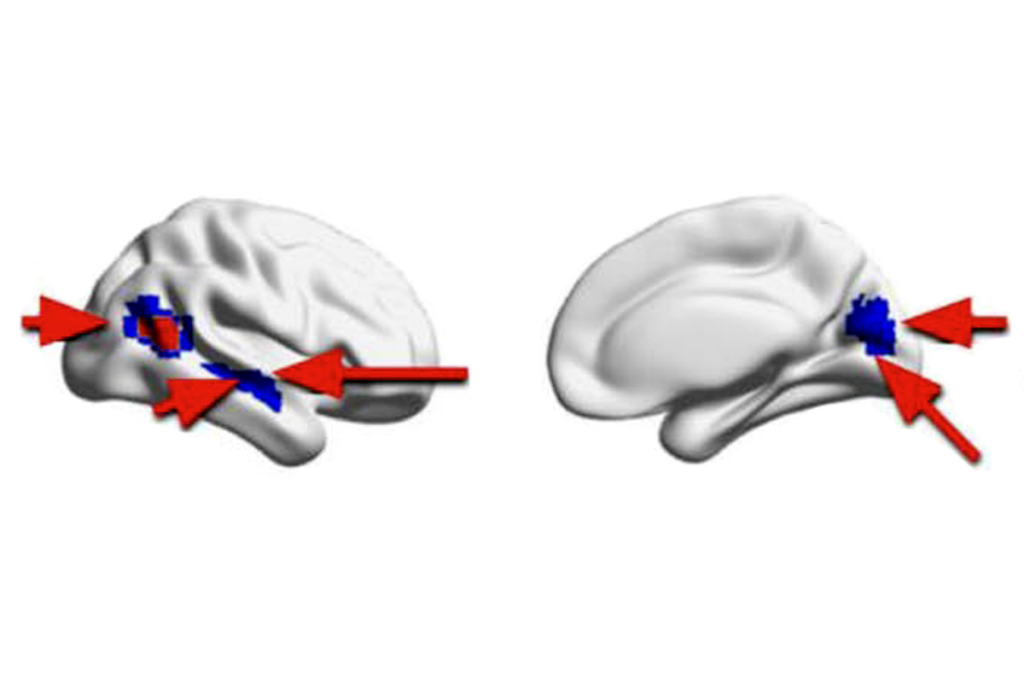
Depression perception; MYT1L mice; brain signal variability
Explore more from The Transmitter
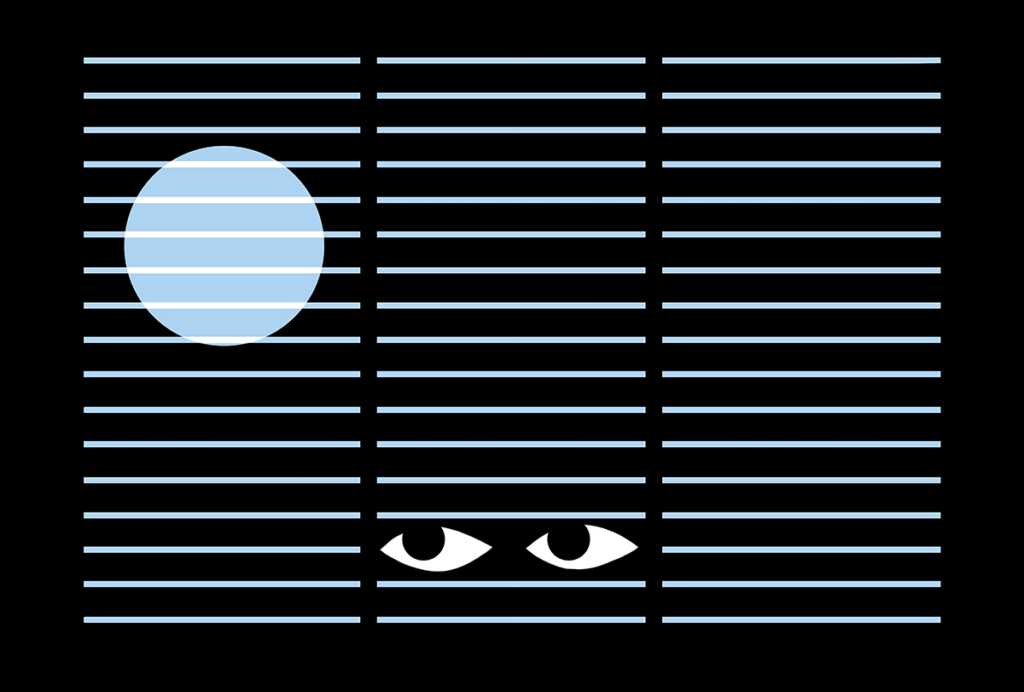
This paper changed my Life: Bill Newsome reflects on a quadrilogy of classic visual perception studies
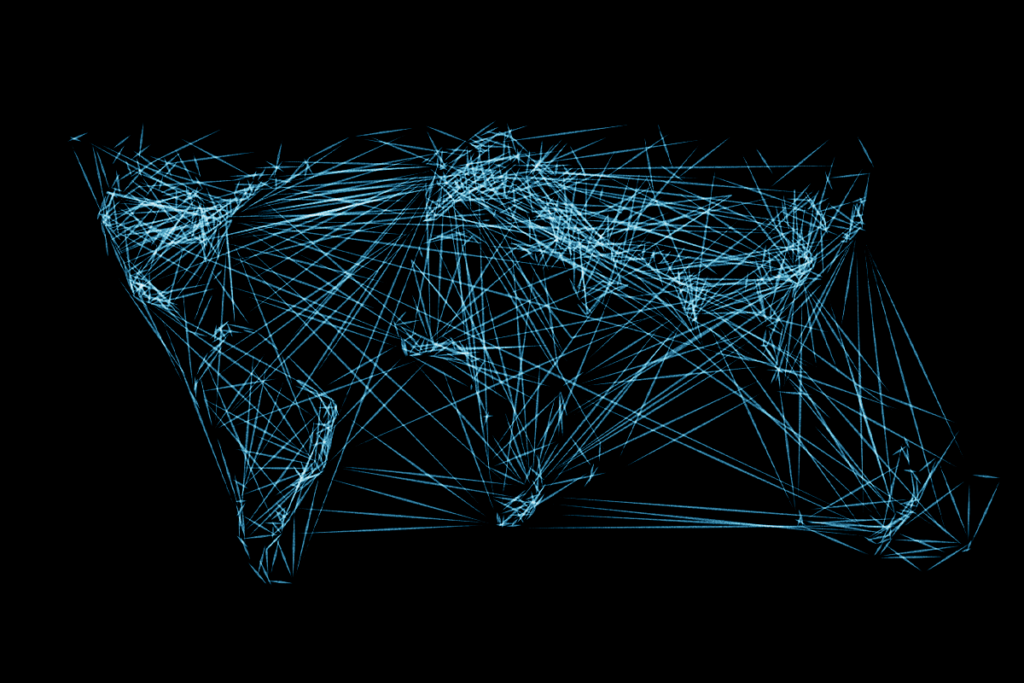
Science must step away from nationally managed infrastructure
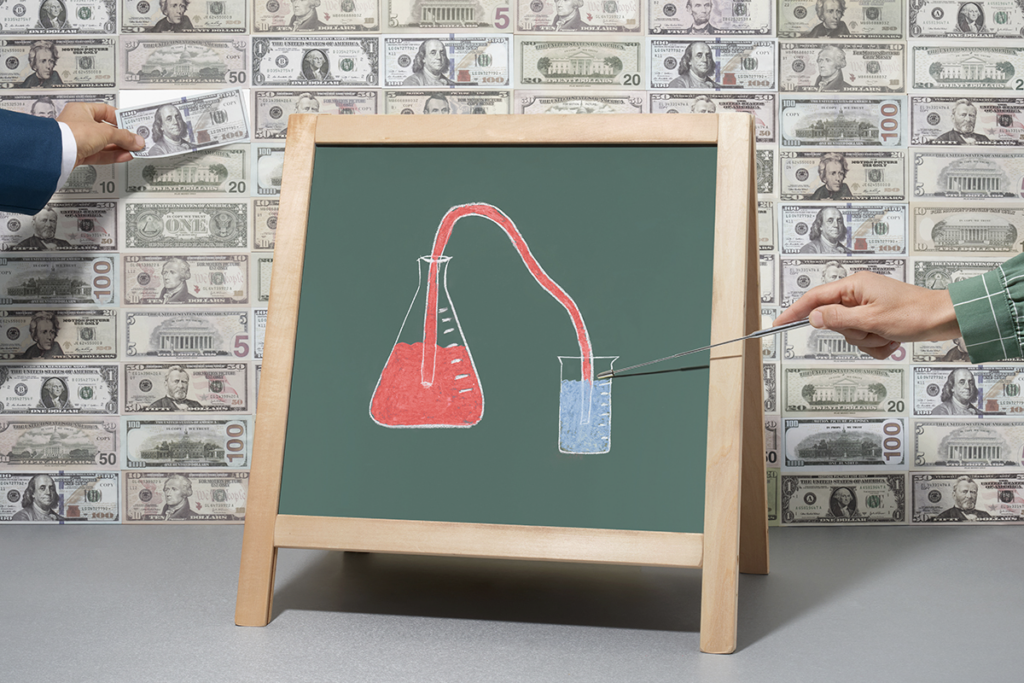