A call for open-science approaches in autism research
Sharing data and tools is universally efficient, but the study of autism in particular presents challenges that can benefit from an open-science framework, says Randy Buckner.
-
Open science is essential to autism research. By ‘open science’ I mean the sharing of data with the research community and committing to making tools and approaches available to all research scientists, even those with competing ideas and from disciplines outside autism research. Sharing data and tools is universally efficient, but the study of autism in particular presents challenges that can benefit from an open-science framework.
One answer to this challenge is an initiative I am involved with called the Brain Genomics Superstruct Project. This project coordinates independent neuroimaging research in the Boston area, ensuring consistent protocols and data collection and enabling sharing among researchers. The initiative has generated a database of neuroimaging data from thousands of individuals, which has allowed us to identify links between brain function and behavior. This would not have been possible to do with smaller studies.
One reason autism in particular would benefit from openness is that a major component of the genetic risk for autism involves rare genetic variants. The traditional laboratory structure does not allow access to enough individuals with the same genetic variant to study its effect in depth. And it is unlikely that any single laboratory will have access to multiple individuals with different rare variants, which can provide insight into common functional pathways.
The second challenge is that the study of autism requires diverse areas of expertise. Linking molecular disturbances to the complex symptoms that emerge in people requires input from many levels of analysis. Relevant groups are led by clinicians, molecular biologists, geneticists, anatomists, systems neuroscientists, psychologists and individuals who are building new tools using physical and computational sciences.
Open science changes the mode of interdisciplinary exchange, because data and tools become available to large numbers of investigators, including those with backgrounds not typically associated with autism research.
Multiple approaches:
The benefits of open science for autism research are clear, but it’s unclear how best to facilitate open-science projects — often of the ‘big science’ type — and how to balance them with the innovative work best conductedinlocal laboratories.
The most direct approach to sharing data is when individual laboratories provide their data openly to the community. Other approaches to making large datasets available are beyond the scope of individual laboratories. These approaches can be divided into three categories: centrally organized, confederated and centrally facilitated.
The first approach uses central organization and funding to build the necessary infrastructure to collect and openly disseminate a needed dataset or resource to the community.
A historic example is the Alzheimer’s Disease Neuroimaging Initiative, which includes extensive longitudinal imaging measures and biomarkers for more than 1,000 individuals. Run much like a clinical trial, the effort is centrally organized, with protocols and quality-control procedures disseminated to 57 clinical sites. Each site enrolls its own participants and feeds the data back to the central repository. The initiative immediately makes the data openly available to the community and has led to numerous publications.
The Simons Variation in Individuals Project (Simons VIP), funded by SFARI.org’s parent organization, is another example of centrally organized sharing. The project is enrolling hundreds of volunteers with rare deletions or duplications of the 16p11.2 chromosomal region, and collecting extensive psychological and neurological measures, along with neuroimaging data, from each individual.
The program flies the participants to one of two locations with identical magnetic resonance imaging and magnetoencephalography scanners. This ensures uniformity in the data acquisition.
Confederated approaches fall at the opposite end of the spectrum, aggregating data through a decentralized model. Such grassroots efforts combine complementary data from many different, small investigator-initiated projects.
A recent example in the neuroimaging community is the 1000 Functional Connectomes Project and its offshoot, the International Neuroimaging Data-Sharing Initiative.
Many laboratories were collecting structural and functional connectivity data, but with a relatively small sample size. The initiative pooled data from 35 international centers and released 1,414 functional neuroimaging datasets to the community, which have become a central reference for the field.
Decentralized approaches can rapidly aggregate data from tens of thousands of participants. The downside is that fully decentralized approaches usually yield incomplete or incompatible datasets, because each of the contributing efforts evolved on its own.
Centrally facilitated approaches are hybrid models with features of both of the above. They take advantage of existing investigator-initiated research projects, but they also use central facilitation to obtain compatible data that can be aggregated.
Layered efforts:
The Brain Genomics Superstruct Project is an example of this approach. We set up a central organization to entice some of the researchers conducting the more than 3,000 neuroimaging studies each year in the Boston area to use matched scanning protocols. We also provided an easy way for investigators to securely upload data to a central repository, provided kits for saliva collection (for DNA), and directed study volunteers to a website that collects detailed clinical data.
The project is named ‘superstruct,’ which means to build on top of an existing structure — in this case, the many ongoing investigator-initiated research projects. The key to the project’s success is to keep the burden of effort low enough so that it can be easily incorporated into existing studies.
In three years, the project has yielded a database of more than 3,500 individuals who have compatible neuroimaging, behavioral and genetic data. This is the largest functional neuroimaging dataset collected to date. These data, which initially investigated variation in the general population, have generated insight into how individual differences in brain function relate to anxiety and social abilities.
For example, we have discovered that young adults who dislike social situations and have poor social skills have anatomic atypicalities in medial prefrontal brain regions associated with emotion regulation and behavior. The data are being used as a normative reference to understand autism.
The downside of centrally facilitated approaches is that the overall amount of data that is collected is limited. This is because we want to keep the amount of work involved reasonable, so that many different laboratories are able to participate.
Many historic discoveries begin with an inspired individual toiling for years or even decades on his or her own vision. Open-science projects have to be constructed carefully so as to still foster this initiative, which is the engine that drives scientific progress.
The balancing act is tricky because, despite all of their merits, open-science initiatives can be detrimental to individual investigators, especially when they are built from a centralized infrastructure within a ‘big science’ culture.
For example, funding given to large initiatives can be at the expense of the smaller research projects of individual investigators. In these lean times, when scientific funding is low, this means productive local laboratories could go unfunded.
However, there is not a zero-sum exchange between large open-science initiatives and funding for local efforts: Funding agencies and philanthropic organizations are more likely to invest in a field that is well organized and collaborative. Large datasets also allow discoveries to be replicated and generalized, which attracts research investment.
Local resource:
A more subtle challenge is to provide a way for junior investigators, particularly students and postdoctoral fellows, to explore their own ideas and to receive proper credit within the culture of open science. This may not be a problem for some types of open science, such as novel computational approaches to large datasets. But for other types, the individuals collecting the data may become invisible.
Large open-science initiatives can also undermine the work of local laboratories simply because they are so prominent. Once a large open-science community effort is announced, local efforts get compared to it.
It is not uncommon to hear in a grant review, “Isn’t effort X already doing that?” even when the local project is quite different and answers questions the large effort does not. The loud voice of a centralized effort can unintentionally drown the softer voices of innovative local investigators.
There is no simple solution for balancing the merits of open-science projects with the desire to foster individual initiative. But we should consider some guiding principles.
First, apply big-science approaches only when they are needed. This means working hard to limit the scope of big-science initiatives to their deliverables, and curbing the tendency for such efforts to become lobbying blocks for perpetual funding. We have a responsibility to allow other projects to grow.
Second, protect contributing scientists’ interests. The goal of making data broadly accessible to the community needs to be balanced with a responsibility to foster the intellectual development and careers of young investigators. Granting authorship to all contributing individuals is not enough. Opportunities for intellectual initiative need to be built in. For example, giving contributing investigators a head start in analyzing collected data or the opportunity for leadership within the project’s sub-goals.
And finally, if a large amount of funding is given to a single big-science project, it should be balanced by more modest funding allotments to many individual projects. Similarly, project funding could reward investigator-initiated efforts that are able to accommodate the add-on components that build toward unique open resources.
The centrally facilitated approach to open science is appealing because it inherently balances a shared objective with the goals of local investigators.
Our responsibility is to encourage diversity in the research community — diversity in the hypotheses explored, the approaches employed and the individuals supported.
Randy L. Buckner is professor of psychology at Harvard University.
Recommended reading
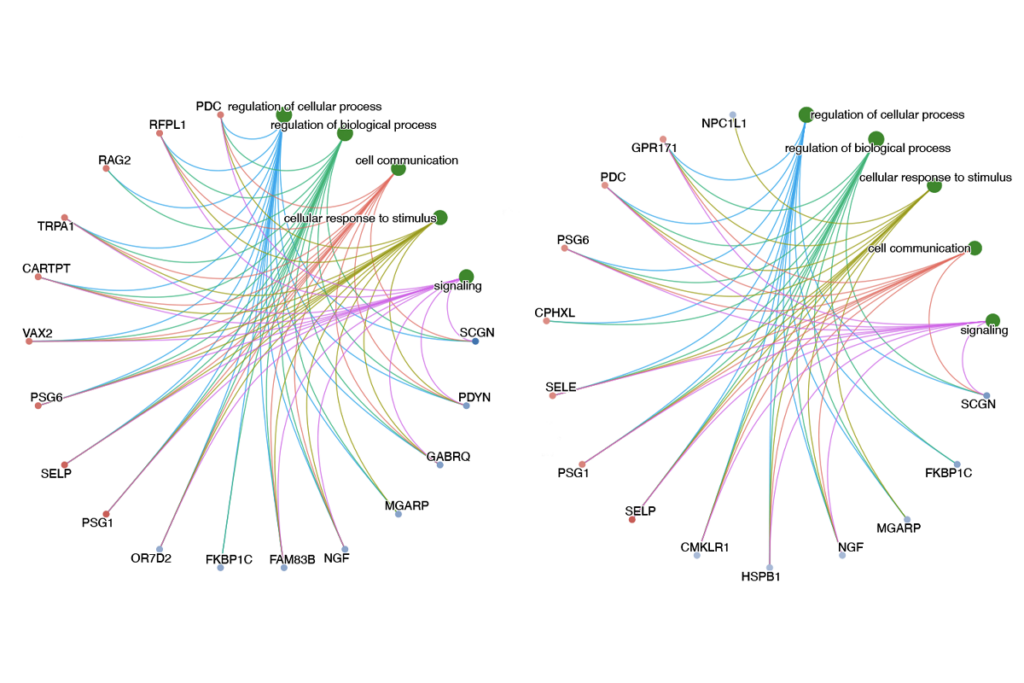
New tool may help untangle downstream effects of autism-linked genes
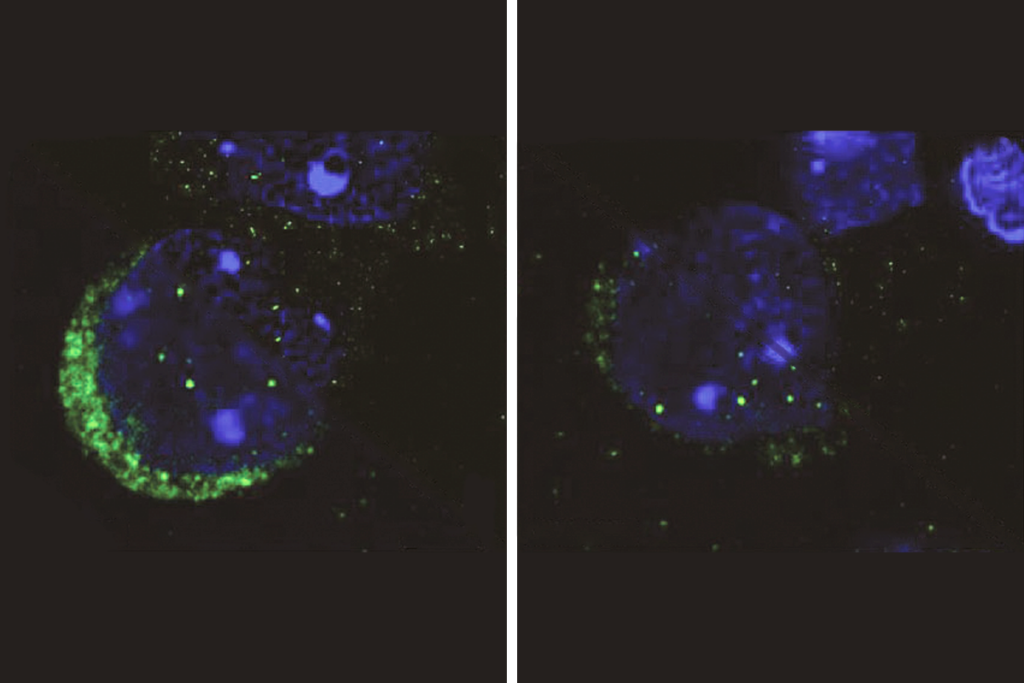
NIH neurodevelopmental assessment system now available as iPad app
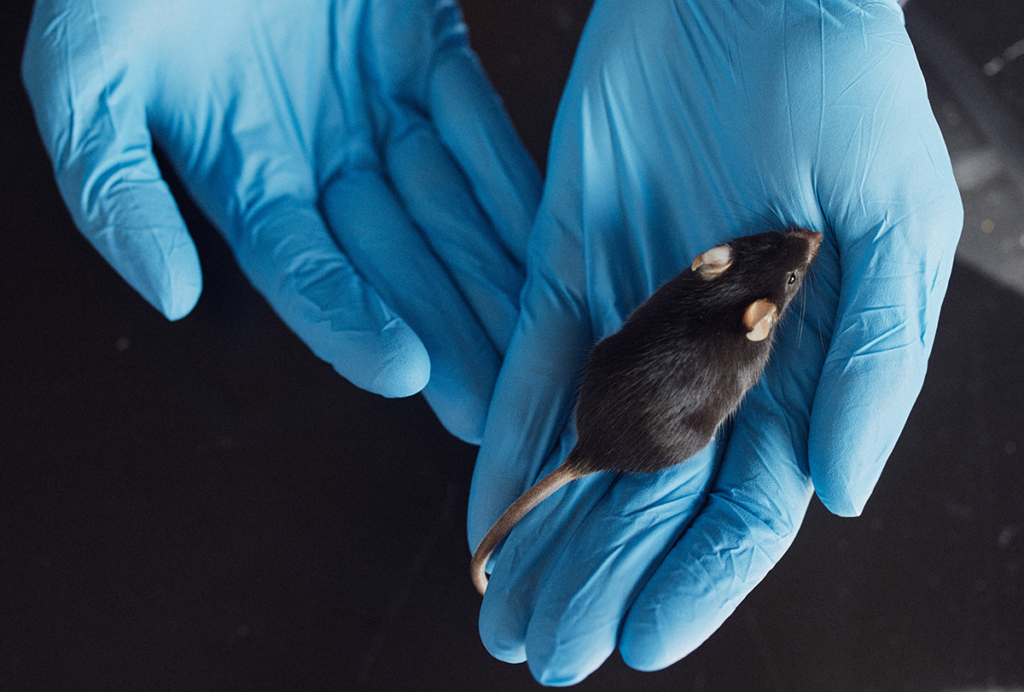
Molecular changes after MECP2 loss may drive Rett syndrome traits
Explore more from The Transmitter
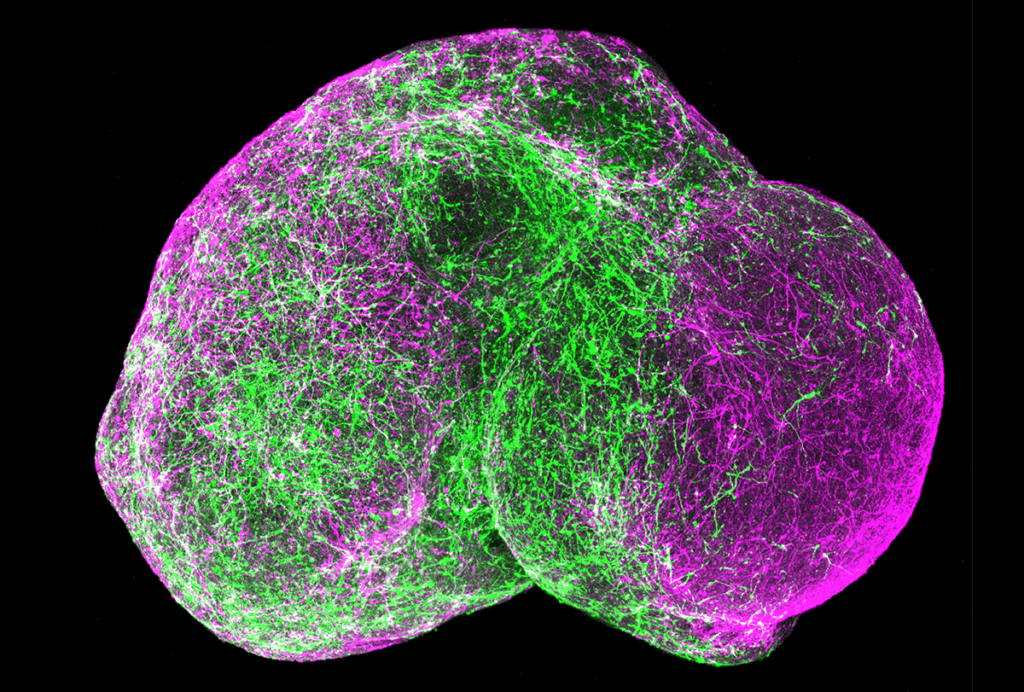
Organoids and assembloids offer a new window into human brain
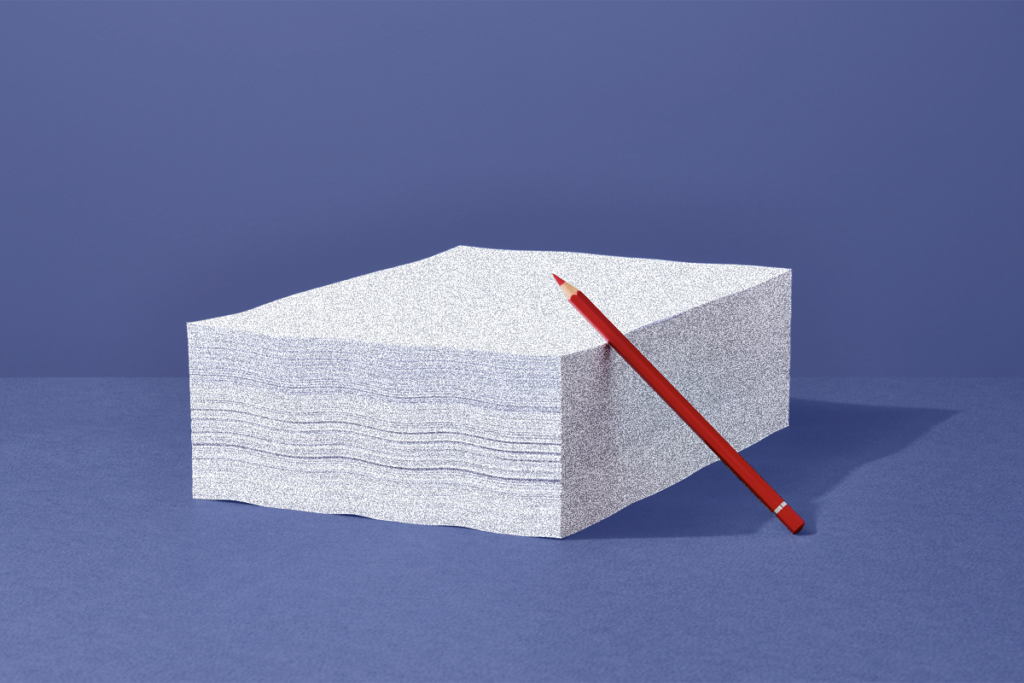
Who funds your basic neuroscience research? Help The Transmitter compile a list of funding sources
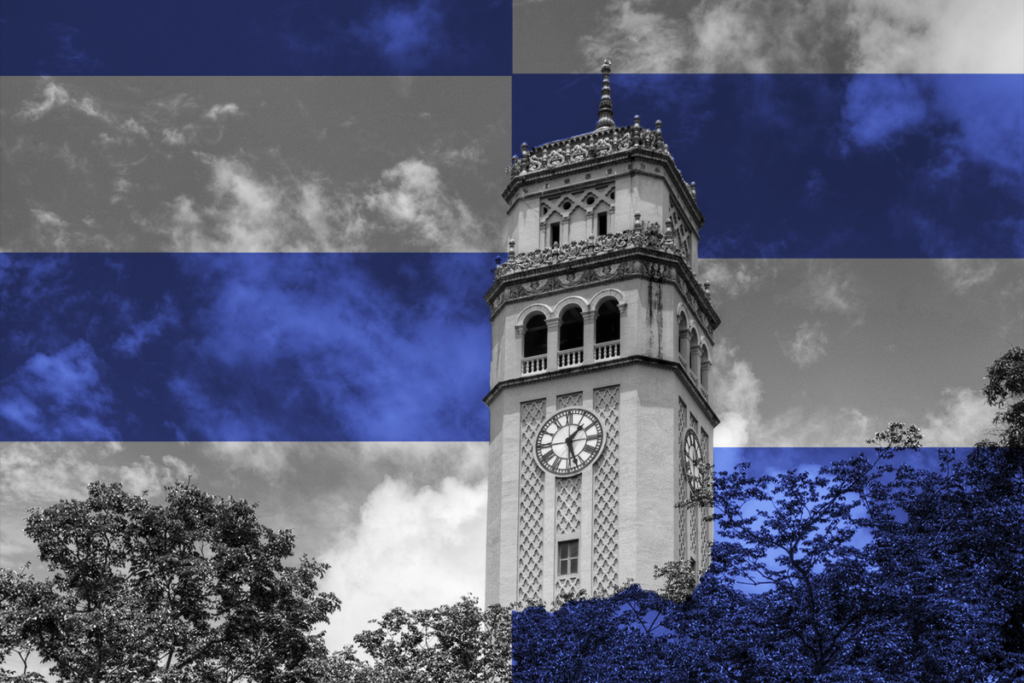