What are we talking about? Clarifying the fuzzy concept of representation in neuroscience and beyond
To foster discourse, scientists need to account for all the different ways they use the term “representation.”
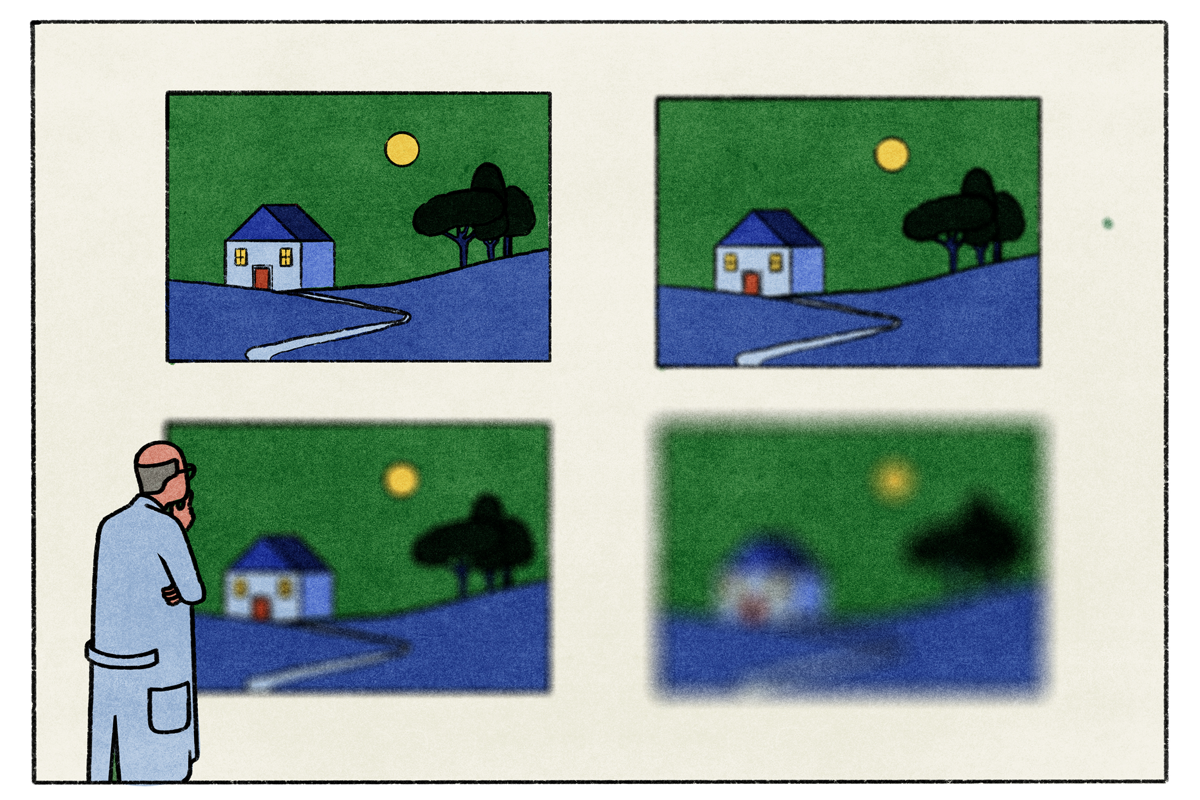
The notion of representation in brain and cognitive sciences is ubiquitous, vitally important, and yet fuzzy. This holds both within neuroscience and beyond, including in cognitive science, artificial intelligence, linguistics, psychology and philosophy of the mind. What people mean when they use the term varies considerably, ranging from a simple correlation between a neural response and a stimulus to a true offline model of the world. Indeed, the members of our group hold many different, often opposing, views on how best to define the concept of representation. To enable clearer usage and facilitate discussion, we hope as a group to develop a catalog of the various uses.
For many, representation is central to the very idea of a science of the mind. This view was already well enough established in 1983 for linguist Noam Chomsky to write: “It is fair to define cognitive psychology as the study of mental representations — their nature, their origins, their systematic structures, and their role in human action.” Consider also this entry from the Encyclopedia of Philosophy: “Mental representations are the coin of contemporary cognitive psychology, which proposes to explain the etiology of subjects’ behavior in terms of the possession and use of such representations.” A common application is within computational models of cognition, where models can independently draw on discrete representations, recombine them in a compositional manner and operate on them to enable planning, reasoning and problem-solving.
Cognitive scientists use the term representation to posit an entity that lies at the basis of cognition, but neuroscientists instead use it to indicate that behaviorally relevant information is detectable in single neurons, circuits and neural populations. This more casual or correlational usage of the term makes fewer theoretical commitments, but as a result it is often not clear what additional work it does beyond referring again to the mere association itself.
These types of correlations are quite easy to detect, and new findings question whether all of them should be referred to as representations. For example, recent studies have found that activity across the brain, even in early sensory neurons or areas, correlates with a variety of properties, including ongoing actions, choices and behavioral engagement. Is the activity of these neurons causally relevant to cognitive function in every case — meaning, for example, does that neural activity actually contribute to the animal’s decision — or is it just epiphenomenal? Regardless, this recent work showing correlations everywhere raises questions about whether simple correlation is a strong enough basis for representation.
Even in the realm of cognition, there have been attempts that seem to forego mental representations altogether and instead emphasize connectionist and dynamical systems views. Such views often, but not always, eschew the requirement for an overt representation as a vehicle with content that exists in some correspondence with the world. Instead, behavior is the output of a distributed network operating on an input. A central pattern generator, for example, can drive locomotion without having to explicitly represent a leg anywhere inside its circuitry. Larger networks could operate as a scaled-up version of this.
Proponents of such views may still want to apply the term “representation.” For example, single cells in a central pattern generator that correlate with leg kinematic variables such as position and speed could be said to represent them. But mere correlation does not seem like a compelling basis for attributing representation. There may also be a parallel lesson to draw in AI: Peering into artificial neural networks and finding responses that look like the ones found in the primate cortex has led some to posit similar representations. But do similar responses in a specific layer mean that a similar overall representation is present? Again, as in the single-cell case, it is not clear what applying the term “representation” would mean, beyond indicating that task-relevant information can be found distributed throughout the network.
W
hat to do? One temptation is to remain vague about what the term “representation” means, to avoid impeding its use in any discipline-specific research. Another temptation is to deflate its meaning to its most minimal form: Use it whenever there is information in neural data, single neurons or neural populations that correlates with task features in the broadest sense. Alternatively, perhaps the term should be reserved for only the most maximal form: referring to a discrete type of true neural state with flexible abstract content, which can be activated absent its original cause — i.e., a representation that is in fact used as a representation.Some argue that this last type of representation should be considered categorically distinct from other, looser senses of the term. It might even be that this is what distinguishes uniquely human thinking. Still others, largely in allergic response to this more fleshed-out notion of representation, have called for the term’s elimination from the scientific literature (A 1990 paper by Walter Freeman and Christine Skarda is an unambiguous example, but see also 2019 work by Rafael Nunez and his colleagues, a 2019 article by Romain Brette, a 2017 paper by Daniel Hutto and Erik Myin, Alva Nöe’s 2006 book on perception and Anthony Chemero’s 2011 book on cognitive science).
Because the options are either problematic or controversial, we propose that a catalog of the different senses of representation would greatly facilitate communication in cognitive science. Such a taxonomy would enable scientists to choose descriptors of varying levels of specificity and inspire researchers to more carefully consider when, how and whether they use it, and to communicate what they mean more explicitly. The taxonomy need not favor any particular theory of representation, nor even assume its existence; rather, it would help set the terms for discussion surrounding an otherwise ambiguous and confusing term. We submit that there is a growing will in neuroscience, philosophy and the cognitive sciences to engage in just such a project (see also a 1987 essay by Ernst von Glaserfeld and a June 2023 paper by Luis Favela and Edouard Machery).
In this essay series, writers with very different perspectives on the concept of representation will outline their views relating to the topic. We hope this exploration will help spark reflection and discussion.
The RPPF is hosted at Trinity College Dublin in Ireland and is funded by an Institutional Strategy Support Fund grant from the Wellcome Trust.
Tomás J. Ryan (Trinity College Dublin)*
Rosa Cao (Stanford University)
David A. Haig (Harvard University)
Yohan J. John (Boston University)
Celeste Kidd (University of California, Berkeley)
Kevin J. Mitchell (Trinity College Dublin)
Melanie Mitchell (Santa Fe Institute)
Lorina Naci (Trinity College Dublin)
Timothy J. O’Donnell (McGill University)
James R. O’Shea (University College Dublin)
Fionn O’Sullivan (Trinity College Dublin)
Rebecca Wheeler (Trinity College Dublin)
Daniel C. Dennett (Tufts University)
Mark Sprevak (University of Edinburgh)
John W. Krakauer (Johns Hopkins University)
* denotes joint lead authorship, alphabetically
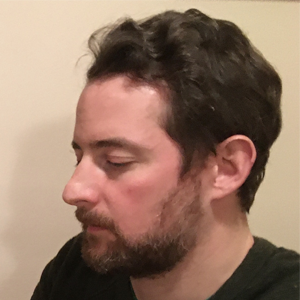
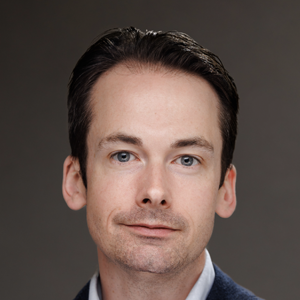
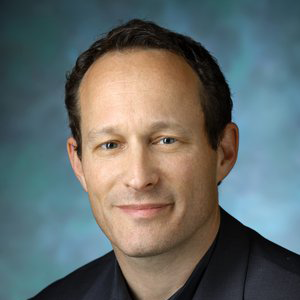
Recommended reading
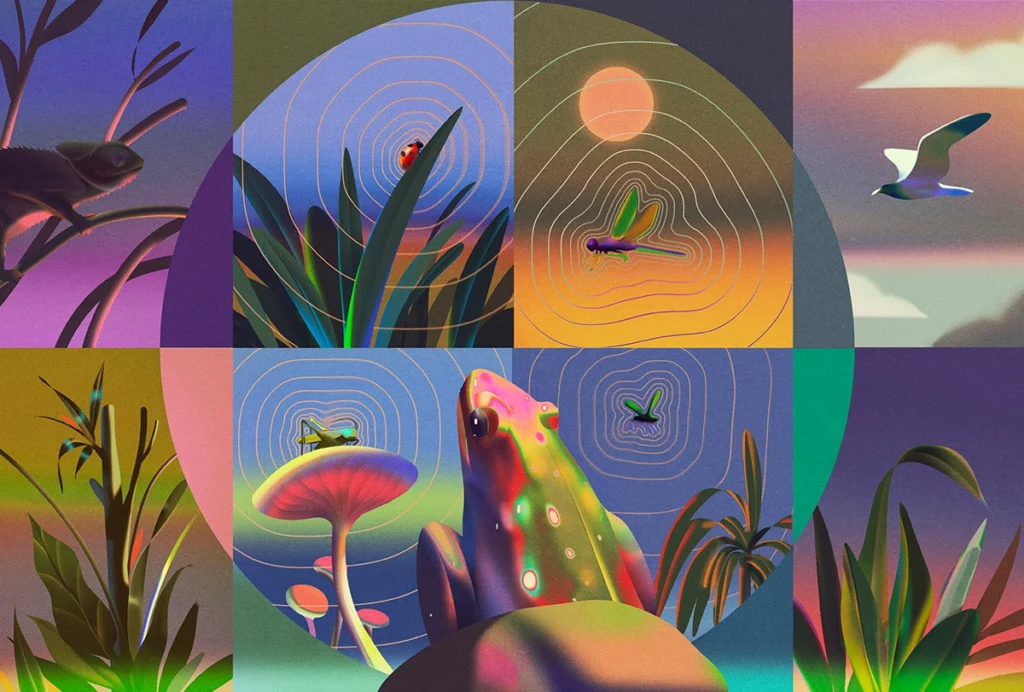
When do neural representations give rise to mental representations?
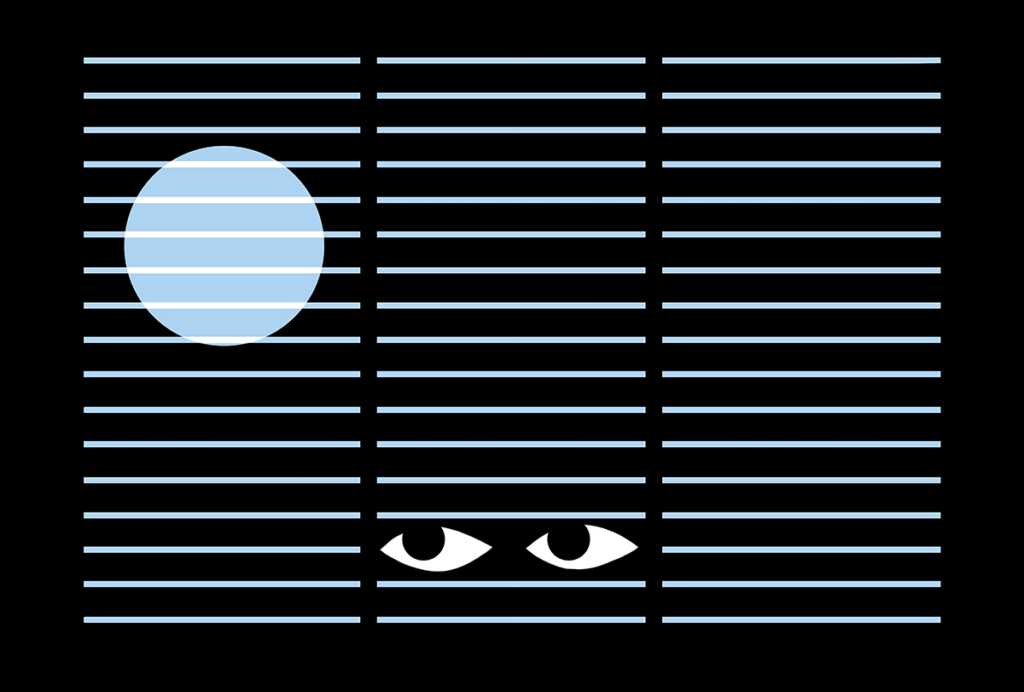
This paper changed my Life: Bill Newsome reflects on a quadrilogy of classic visual perception studies
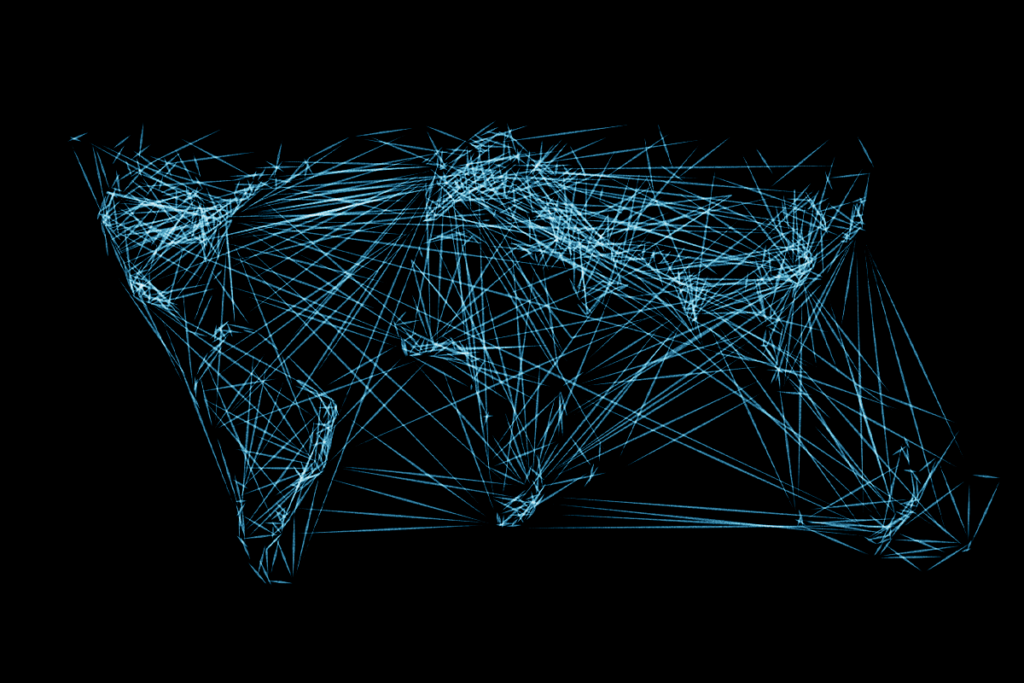
Science must step away from nationally managed infrastructure
Explore more from The Transmitter
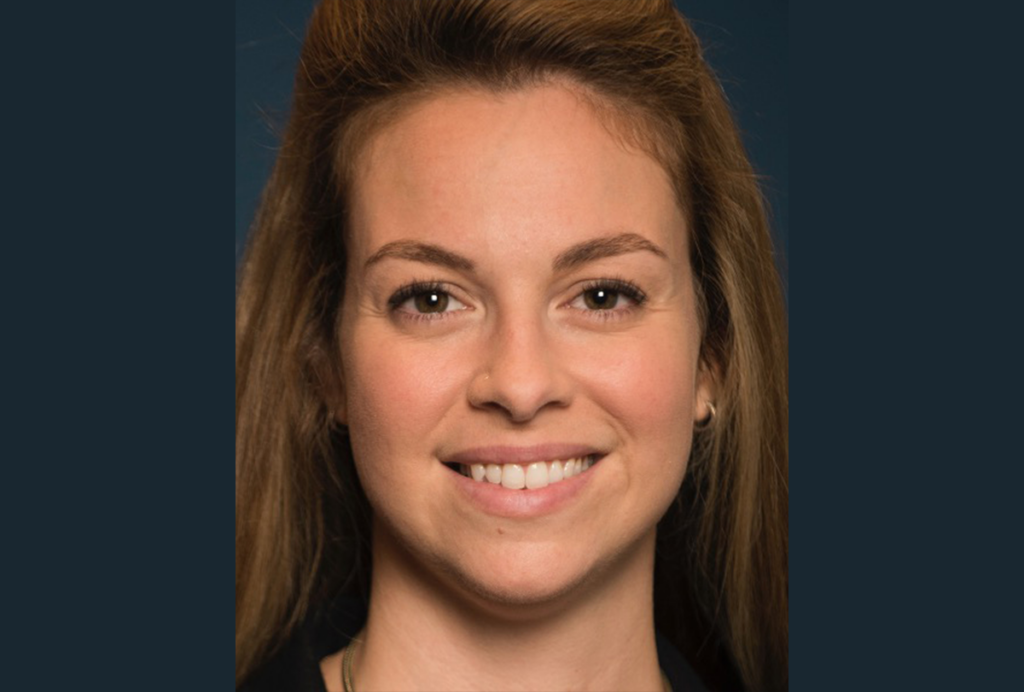
Autism program chief among National Institutes of Health layoffs
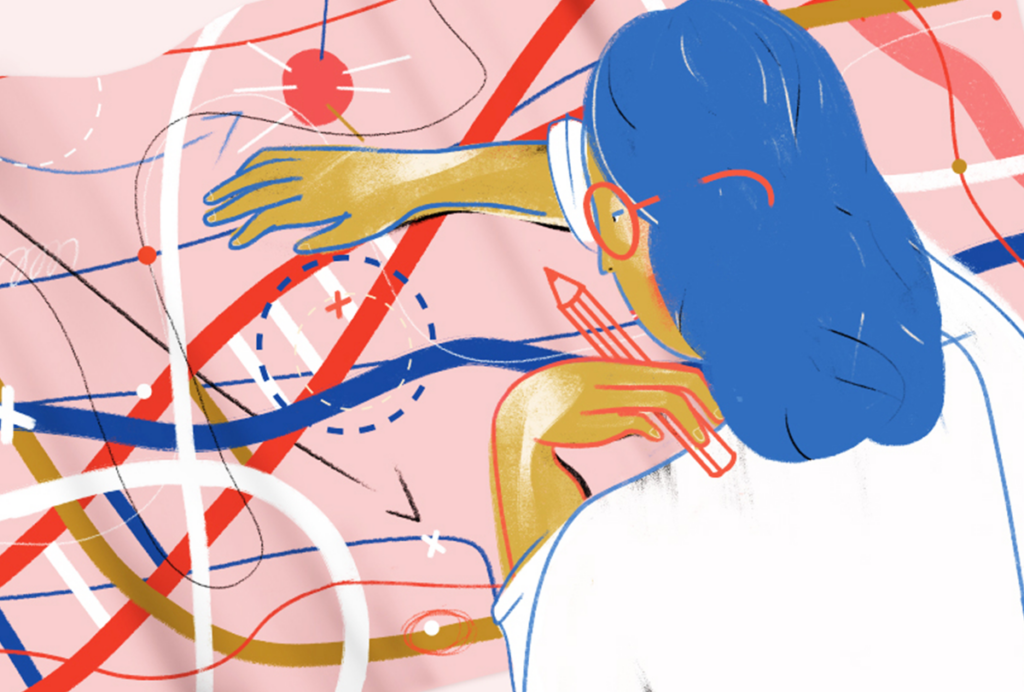
Familiar autism-linked genes emerge from first analysis of Latin American cohort
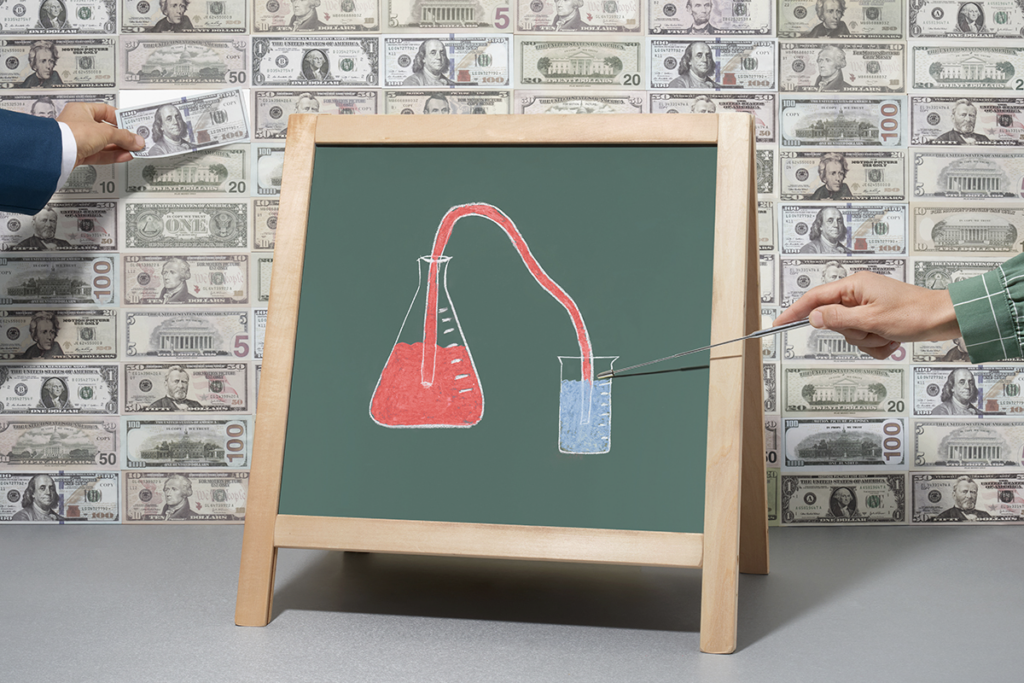