The S-index Challenge: Develop a metric to quantify data-sharing success
The NIH-sponsored effort aims to help incentivize scientists to share data. But many barriers to the widespread adoption of useful data-sharing remain.
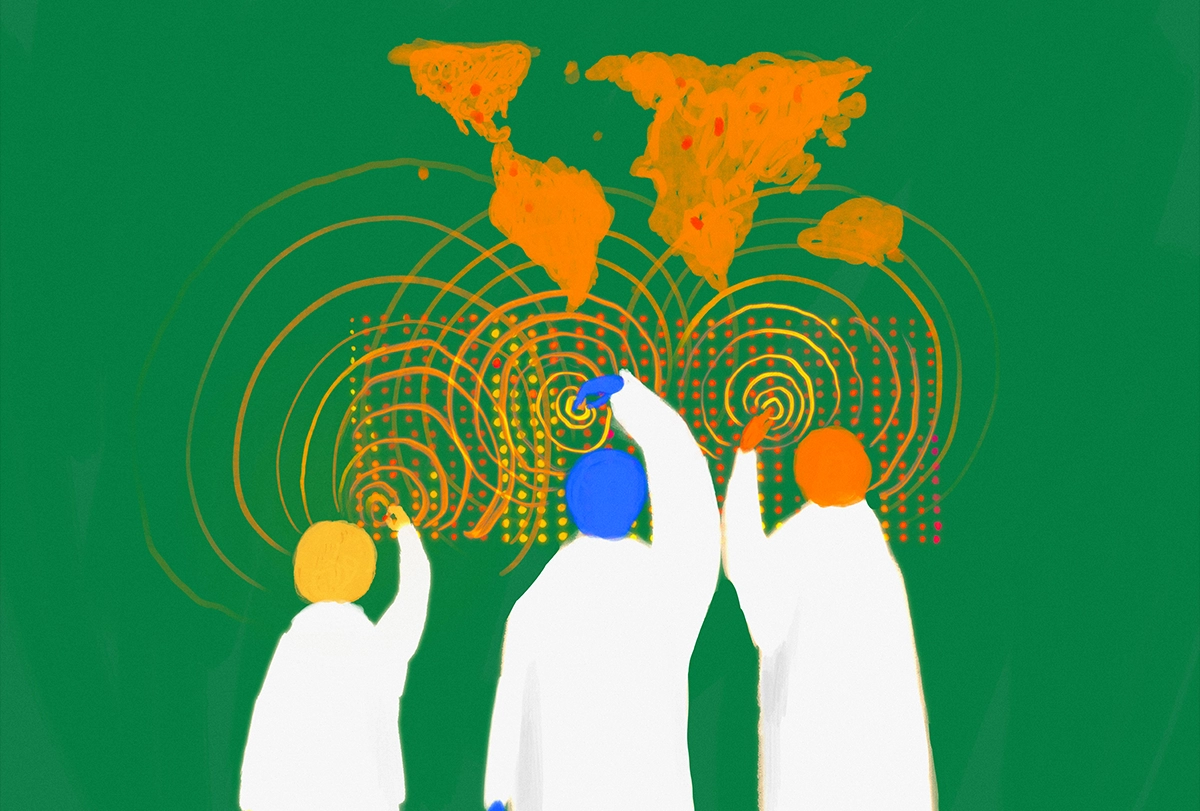
There is a growing recognition in the neuroscience community that efforts to improve data-sharing are, at least in principle, a good idea. Sharing the data generated by experiments is critical for reproducibility, and it enables reuse of data that may have taken years to collect. Sharing the code used to transform data into scientific results is also critical, both to boost reproducibility and to reduce the amount of time trainees spend developing these tools.
But sharing neuroscience data in a useful way is difficult, often requiring extensive effort and garnering limited official recognition or reward. As a result, little incentive exists to spark broad behavior change in the community. That may soon change, thanks to a new National Institutes of Health-sponsored effort, the Data Sharing Index (S-index) Challenge. Announced last month, it provides a $1,000,000 award to the individual or group with the best idea on how to quantify sharing. The goal is to provide a simple metric that would allow both hiring and promotion committees, as well as funders, to recognize and reward sharing.
I think this is a great idea. These challenges encourage creative brainstorming about new incentives and will hopefully move us toward the behavior change we need. The million-dollar prize recognizes the difficulty involved: The index would ideally consider not only how many datasets or software repositories a group shares, but how useful they are to the community. At the same time, the S-index would address only a small part of the overall problem. Major hurdles still remain—most notably, limited funding to support data-sharing tools and limited technical expertise to adopt them.
T
he hurdles to data-sharing are many. To make shared data useful to others, someone first needs to decide which data (raw, preprocessed or just the numbers that go into the figures of a paper) should be shared—choices that greatly influence the utility of the shared data. They also have to package up the data, including all the metadata that contains the key experimental elements, in a way that is comprehensible to others. The same is true for code; writing code designed to derive a result and writing code that is useful to others can be very different things, and much of the code written in a laboratory is incomprehensible to anyone other than the author (and sometimes even to them.)Some groups have recognized that sharing enables data reuse and collaboration within their lab and between their lab and other labs, but these benefits are not sufficient for most to make sharing a priority. Instead, data-sharing is typically an afterthought: Only once a result is ready for publication, or perhaps when it’s accepted for publication, does the substantial work of sharing begin. And even then, often no one checks to see if the shared data and code are comprehensible and usable, further relegating sharing to an afterthought.
Funders and others have launched various efforts to try to remedy this problem. Funders, both public and private, are requiring more specific statements about which data will be shared and how they will be shared. Journals are requiring that authors deposit data and code that, at least in theory, would enable a reviewer or a reader to replicate the main results of a paper.
The S-index will, hopefully, further incentivize researchers to think about sharing data and code from the outset—and how to do so usefully. To that end, the S-index will need to offer a way to track when datasets or code are used by others, as well as how often that use turns into one or more publications. Sorting all that out is not going to be easy, but scientists are creative people, and I am optimistic that a workable index can be developed.
But recognizing the efforts involved in data-sharing with an official metric will address only a small part of the overall problem. Many labs simply do not have the expertise to build or adopt a software infrastructure for sharing, and we are still far from a state where a lab head could easily adopt a framework that solves their sharing problems. The BRAIN Initiative, along with private funders, including the Kavli Foundation and the Simons Foundation, have addressed this issue by funding the development of software to make data-sharing easier for everyone. (The Transmitter is funded by, but editorially independent of, the Simons Foundation.) These efforts have supported the hard work of many members of our community who have helped establish data repositories, data standards and open-source tools for sharing and data analysis.
Unfortunately, these repositories, standards and tools require continual support to ensure they remain up to date, and getting that support is difficult. Bringing these tools into use in each laboratory is also hard, and current funding levels are not enough to enable labs to hire the data scientists they would need for this task. These challenges can perhaps help us understand why data-sharing plans often aren’t enforced, and why most journals and reviewers typically don’t check to see whether shared data and code are comprehensible and usable.
As a result, sharing is still largely an unfunded mandate placed upon lab heads who are already struggling to find time to do science in ever more challenging circumstances. My hope is that the S-index Challenge will be successful, that funders will recognize the importance of supporting these efforts, and that the next generation of scientists are given the tools and support they need to maximize their S-index.
Recommended reading
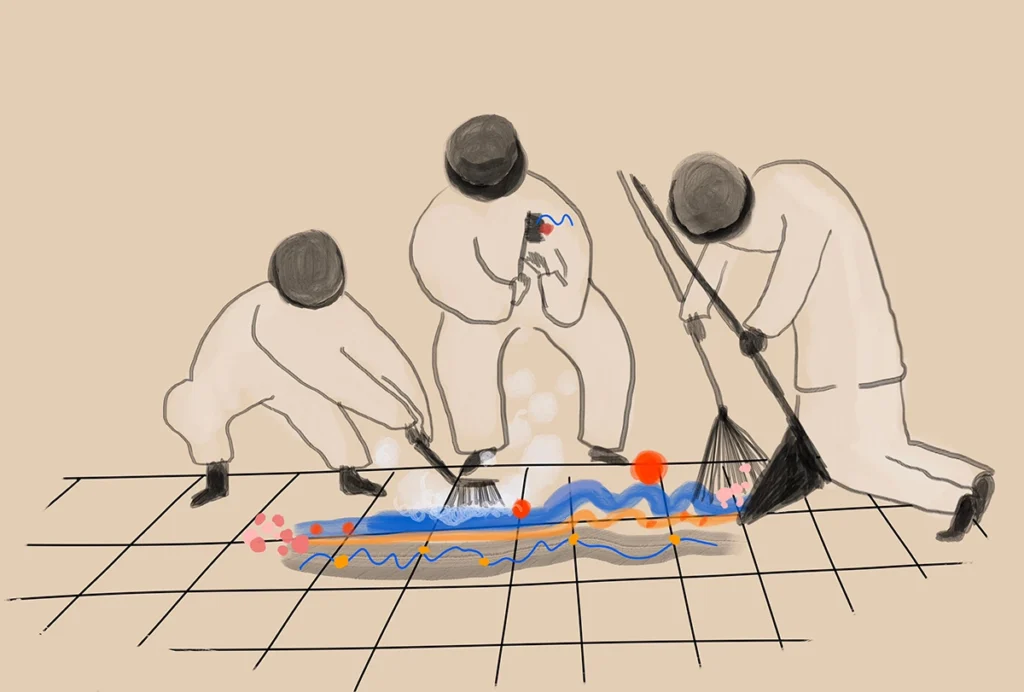
A README for open neuroscience
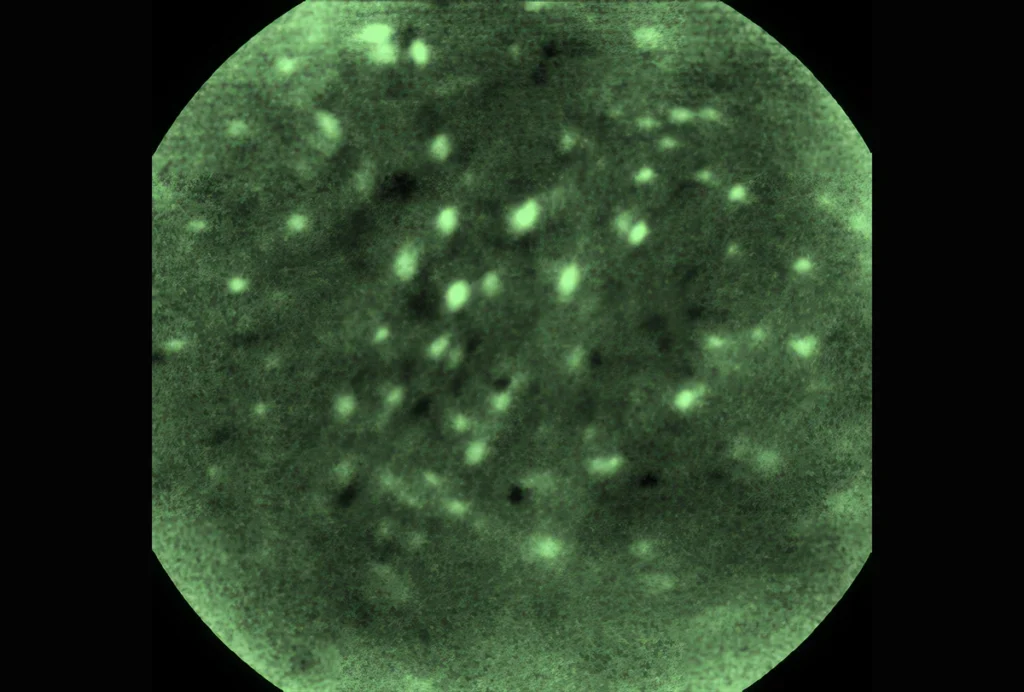
Designing an open-source microscope
Explore more from The Transmitter
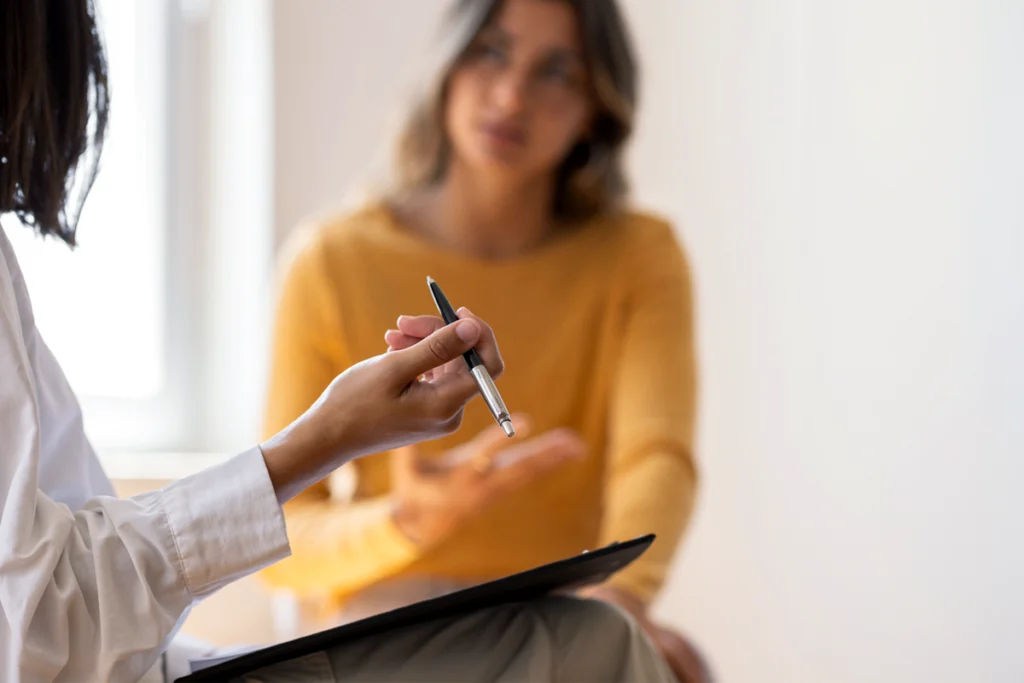
Autism prevalence increasing in children, adults, according to electronic medical records
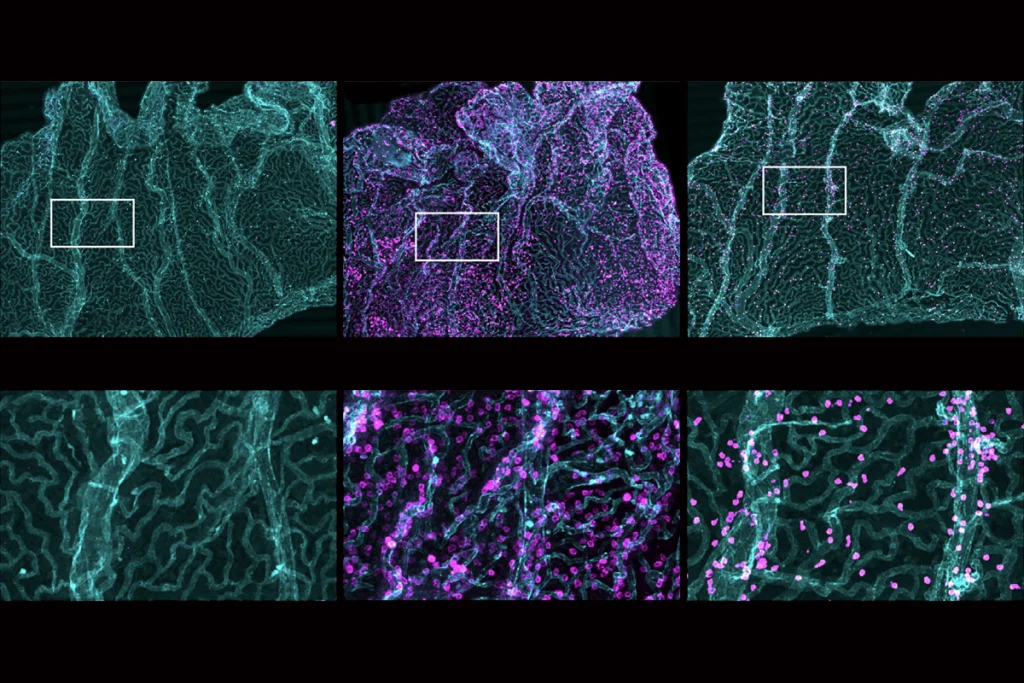