Can an emerging field called ‘neural systems understanding’ explain the brain?
This mashup of neuroscience, artificial intelligence and even linguistics and philosophy of mind aims to crack the deep question of what “understanding” is, however un-brain-like its models may be.
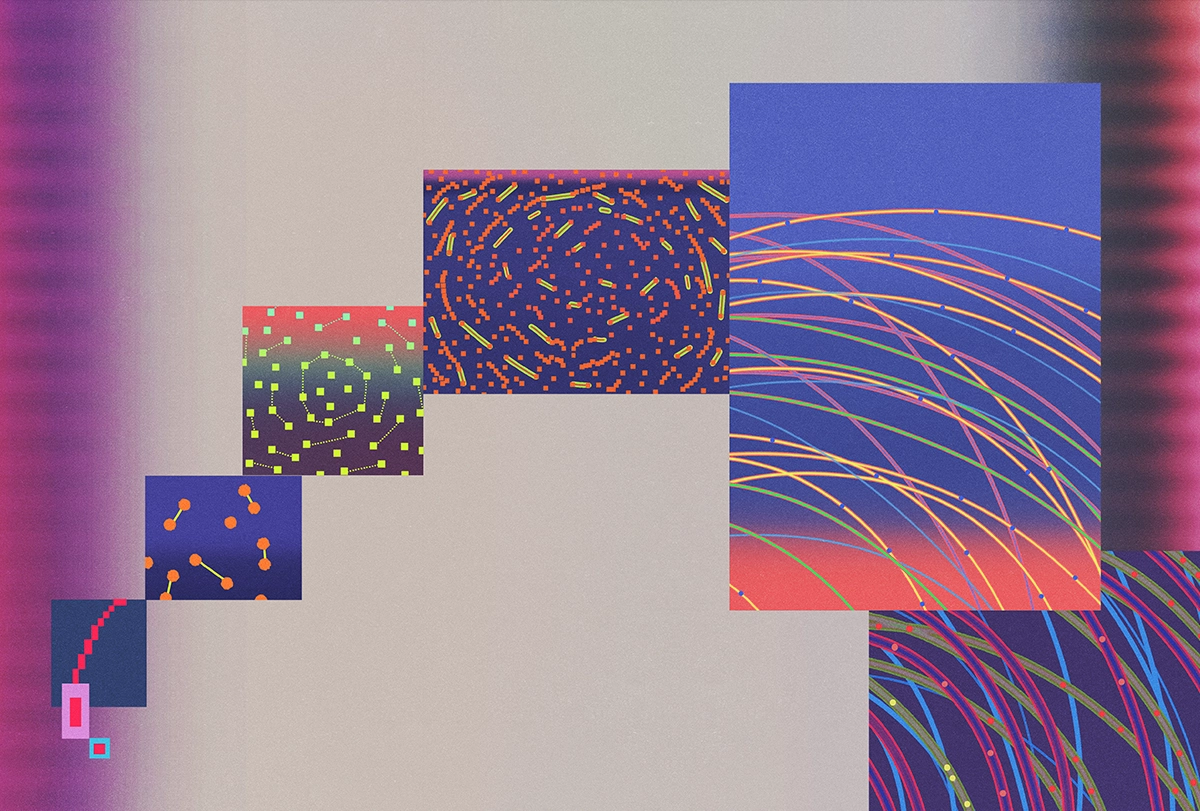
Had you stumbled into a certain New York University auditorium in March 2023, you might have thought you were at a pure neuroscience conference. In fact, it was a workshop on artificial intelligence—but your confusion could have been readily forgiven. Speakers talked about “ablation,” a procedure of creating brain lesions, as commonly done in animal model experiments. They mentioned “probing,” like using electrodes to tap into the brain’s signals. They presented linguistic analyses and cited long-standing debates in psychology over nature versus nurture.
Plenty of the hundred or so researchers in attendance probably hadn’t worked with natural brains since dissecting frogs in seventh grade. But their language choices reflected a new milestone for their field: The most advanced AI systems, such as ChatGPT, have come to rival natural brains in size and complexity, and AI researchers are studying them almost as if they were studying a brain in a skull. As part of that, they are drawing on disciplines that traditionally take humans as their sole object of study: psychology, linguistics, philosophy of mind. And in return, their own discoveries have started to carry over to those other fields.
These various disciplines now have such closely aligned goals and methods that they could unite into one field, Grace Lindsay, assistant professor of psychology and data science at New York University, argued at the workshop. She proposed calling this merged science “neural systems understanding.”
“Honestly, it’s neuroscience that would benefit the most, I think,” Lindsay told her colleagues, noting that neuroscience still lacks a general theory of the brain. “The field that I come from, in my opinion, is not delivering. Neuroscience has been around for over 100 years. I really thought that, when people developed artificial neural systems, they could come to us.”
Artificial intelligence, by contrast, has delivered: Starting with visual perception a decade ago and extending to language processing more recently, multi-layered or “deep” artificial neural networks have become the state-of-the-art in brain modeling, at least for reproducing outward behavior. These models aren’t just idealized versions of one aspect of the brain or another. They do the very things natural brains do. You can now have a real conversation with a machine, something impossible a few years ago.
“Unlike the computational models that neuroscientists have been building for a long time, these models perform cognitive tasks and feats of intelligence,” says Nikolaus Kriegeskorte, professor of psychology and neuroscience at Columbia University, who studies vision at the university’s Zuckerman Institute.
That shift has transformed day-in-day-out scientific methodology. As described at the NYU meeting, working with these systems is not altogether unlike doing an experiment in humans or macaque monkeys. Researchers can give them the same stimuli and compare their internal activity more or less directly with data from living brains. They can create do-it-yourself Oliver Sacks-style case studies, such as artificially lesioned machine brains that recognize all four members of the Beatles but can’t tell a drum from a guitar. Direct interventions of this sort, so useful in distinguishing causation from correlation, aren’t possible in a human brain.
These successes are all the more striking considering that the workings of these systems are so un-brain-like. What passes for neurons and synapses are radically dumbed-down versions of the real things, and the training regimen is worlds apart from how children usually learn. That such an alien mechanism can still produce humanlike output indicates to many scientists that the details don’t actually matter. The low-level components, whether they are living cells or logic gates, are shaped into larger structures by the demands of the computational task, just as evolution works with whatever it has to solve the problem at hand.
“As long as architectures are sufficiently decent, representations are affected much more by the data and training process,” says Andrew Lampinen, a cognitive psychologist at Google DeepMind. “It suggests that predicting and comprehending language are bottlenecked through similar computations across a wide range of systems.”
Given this similarity, scientists should begin to explain brain function in terms of network architecture and learning algorithm, rather than fine-grained biological mechanisms, argued Lindsay, Kriegeskorte and a long list of other eminent computational neuroscientists in a 2019 paper. The machine-learning pioneer Yoshua Bengio, professor of computer science at the University of Montreal and a co-author of the paper, puts it this way: “Neuroscience tends to be more descriptive, because that’s easy; you can observe things. But coming up with theories that help explain why, I think, is where machine-learning ways of thinking and theories—more mathematical theories—can help.”
V
isual neuroscientists have led the way in merging AI and neurobiology, as well they might. The first hardware neural network from the mid-1950s was expressly designed to mimic natural visual perception. That said, over the ensuing decades, AI researchers often gave up any pretense of biological verisimilitude, especially in how they trained their networks. By the 2010s, when image-recognition networks began to identify images as well as people (albeit only on narrowly defined tasks), it wasn’t clear how true to life they really were.To answer that question, neuroscientists at the Massachusetts Institute of Technology soon developed a basic experimental paradigm that those studying other forms of perception and cognition have since adopted. The first step was to show visual stimuli to monkeys and measure their brain responses. Then they trained a range of artificial neural networks on the same stimuli and extracted their “representations”—output encoding the highest abstractions the networks produced. To compare the brain data with this output—typically just a vector of numbers that have no particular biological meaning—called for a “mapping model,” distinct from the artificial neural network itself. Researchers can build such a model by assuming that the simulated and real brains match on some subset of the data, calculating the relation between them, and then testing this relation on another subset of data.
The approach revealed that monkey brains and artificial networks respond to the same visual stimuli in similar ways. “It was the first time we had models that were able to predict neural responses,” says Katharina Dobs, a psychology researcher at Justus Liebig University Giessen. And this congruity was not merely not ad hoc. These systems were designed and trained to recognize images, but nothing required them to do it like a natural brain. “You find this astonishing degree of similarity between activations in the models and activations in the brain, and you know that so did not have to be,” says Nancy Kanwisher, professor of cognitive neuroscience at MIT. “They’re completely different. One is a computer program, and the other is a bunch of biological goo optimized by natural selection, and the fact that they end up with similar solutions to a similar problem is just, to me, astonishing.”
Since then, these networks have changed the scale at which computational neuroscientists do research. Rather than put forward one model of visual perception and defend it, a paper might compare a dozen at once. In 2018, a group of researchers set up the website Brain-Score.org to rank vision models—now more than 200 of them, each representing some intuition for how the visual cortex might work. All perform at a human level on some task, so the rankings represent finer-grained aspects of the models, such as whether they make the same errors our brains make and whether their reaction times vary as ours do. “That gives us a powerful framework for adjudicating among models,” Kriegeskorte says.
These artificial neural networks have opened up new ways to tackle old questions in vision science. Even though the networks are often as opaque as the brain itself, researchers at least have direct access to their artificial neurons—they are just variables in the machine. Lindsay and her colleagues, for example, turned to an artificial network to explore “grandmother neurons,” or the decades-old idea that some brain cells may fire only when you see your grandmother or some other specific person. Lindsay’s team confirmed that an artificial network trained on images had neurons that fired only when certain objects were present. But when they traced the information flow through the network, they found that these neurons had nothing to do with the network’s overall ability to identify people or things; it was just an accident that they responded so selectively.
“Looking in these artificial neural-network models, we actually see that the way a neuron responds to an image doesn’t necessarily tell you the role it plays in classifying things,” Lindsay says.
A
rtificial neural networks have also made it possible to probe deeper into the hierarchy of visual processing experimentally. Textbook neuroscience methods measure the response of neurons in early processing layers such as the retina, the lateral geniculate nucleus and the primary visual cortex. They show that neurons respond to simple stimuli such as lines and gratings of specific orientations. But these methods struggle to characterize cells in late layers that pick up broader and more complex geometric patterns. “As you go deeper into the visual system, it’s harder to find some simple feature like that that explains how the neurons are responding,” Lindsay says.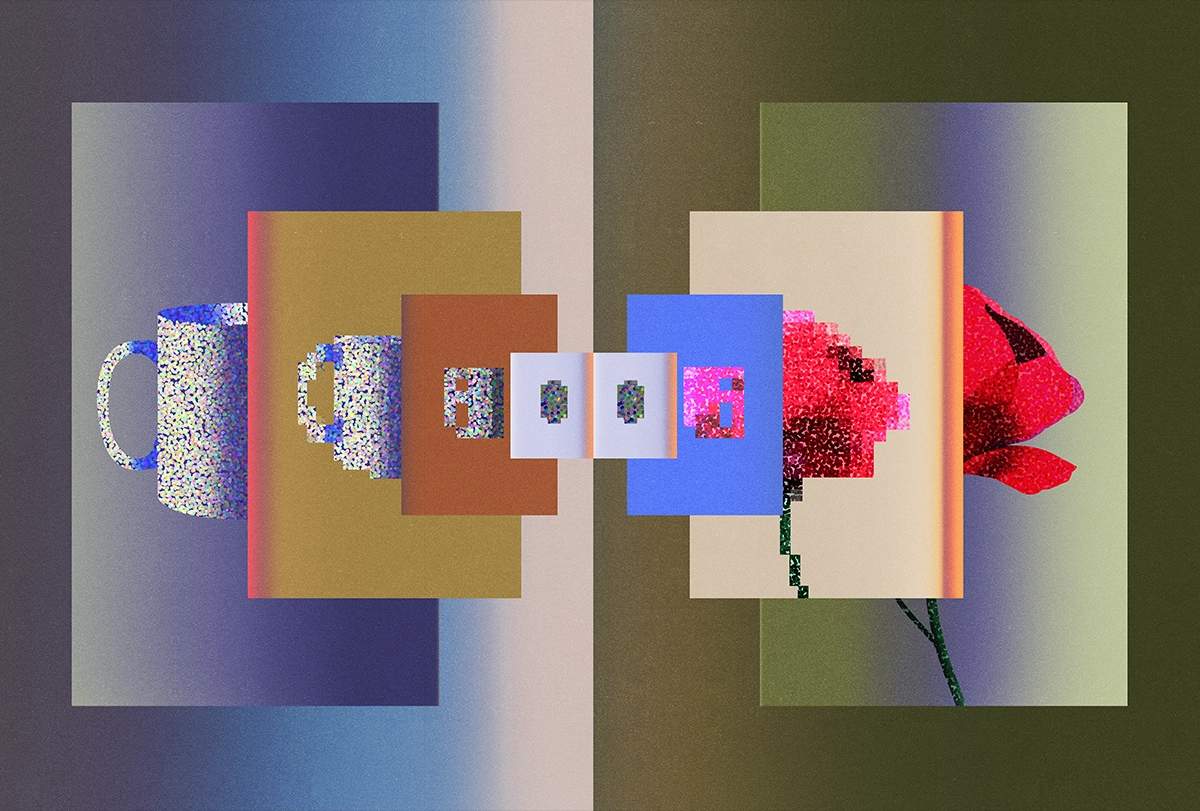
An artificial neural network, however, can find those features. Researchers might train the machine on images of, say, blue coffee cups and blue flowers. These objects look almost the same at the pixel level and evoke similar responses in the early layers; only in a late layer do their differences become apparent. The high-level representations that the machine develops should match those of a brain, Lindsay says. “You just think of it as a data-analysis tool—a different way of representing the data—and then look for that representation in the brain. You could argue that that’s more about language and less about the brain.”
In fact, using artificial neural networks, researchers can even watch the brain in action in realistic settings, or what is known in the jargon as an “ecologically valid experiment.” In a traditional stimulus-response experiment, they have to sedate a mouse to eliminate noise from their measurements of its brain’s response to some simple stimuli. How much better it would be, Lindsay says, to let the animal run around, collect eye-tracking and other behavioral data, and then pass it through a network to look for patterns that might not be immediately obvious. “It relinquishes some of the need for control,” she adds.
Artificial neural networks are shedding light on another long-standing puzzle about hierarchy in visual neuroscience—namely, why visual processing is functionally specialized. Brain imaging shows that certain areas in visual cortex respond more to faces than to other types of objects. “We’ve known that for decades, since the emergence of fMRI, but what we don’t know is ‘Why? What’s special about faces?’” Dobs says. There are broadly two possibilities, she says: Our brains either are born with specialized face-recognition abilities or learn to specialize from seeing lots of faces early in life.
To try to find out, her team built a network of more than 100 million tunable parameters and trained it both to identify 450 different types of objects and to put names to faces for 1,700 celebrities. The network was hierarchical, like the visual cortex, although the layers did not match up one-for-one with their biological counterparts. Then the researchers went through the network lesioning parts of it. Disabling some units hobbled the network’s performance on either faces or objects, but not on both, indicating that those units specialized in one or the other. And a lesion in an early layer, which processes basic geometry, hurt the network equally on both tasks, suggesting the specialization occurred deep.
Apart from exposing the network to lots of celebrities, the researchers did not intimate there was anything special about faces—no need to read emotions, for example. The brain must acquire its specialized face-recognition abilities from experience, they concluded, and a brain or artificial network develops such specialized modules whenever it has to juggle two or more tasks. “It’s a result of trying to do both of these tasks well,” Dobs says. As a test, she and her colleagues also trained the network to categorize foods. “There was no evidence for functional specialization of food processing in the visual cortex, so we thought, OK, we don’t expect it to find it in networks, either,” she says. “When we did that, however, to our surprise, we found segregation.”
Since then, other teams have discovered that the human brain, too, has specialized areas for food recognition, and Dobs and her team are looking for still other examples. “If you have lots of experience with cars, there’s probably also some neurons in your brain that will specialize to process cars,” she says. They haven’t checked yet for three-way specialization on faces, cars and generic objects, she says, but they have found that networks can learn to identify car makes and models as surely as they do faces. And car networks exhibit an automobile counterpart to the face-inversion effect, according to a paper they published last year: Just as we find it harder to recognize upside-down faces, the network had trouble with upside-down car pictures.
A
s challenging as understanding vision has been, language is even harder. Kanwisher recalls: “Six, seven years ago, I used to toss off, in an introductory lecture to undergrads, big, wide, open mysteries, beyond the cutting edge, like: How could a bunch of neurons possibly hold the meaning of a sentence? Whaaaaat? How do we even think about that?” Textbook neuroscience methods are nonstarters. Researchers can map a cat’s visual cortex in detail, but not its language regions—it doesn’t have any. At best, animal models get at only narrow features of language. Central American singing mice have impeccable conversational etiquette but are not known for their use of the subjunctive.Whereas visual neuroscience and image-processing systems developed in tandem, language neuroscience used to have only a loose connection to technology. The field began to adopt artificial neural networks in the 1980s, but these early systems did not attempt to understand or generate language in general. They simulated particular faculties that theorists thought might be learned rather than innate, such as constructing verb tenses, and were known more for stirring up controversy than settling it. “Language neuroscience, the field, has been quite unsatisfyingly informal for a very long time,” says Evelina Fedorenko, professor of neuroscience at MIT.
With large language models such as GPT, researchers are now making up for lost time. At the mechanistic level, these models are even more un-brain-like than image-recognition models. The areas of the brain associated with language are thought to be a thicket of feedback loops, whereas language models are feedforward systems in which data flows from input to output without looping around. These systems, however, do have special “transformer” layers—the “T” in GPT—that perform some of the roles that feedback would, such as tracking the context of words, and that, according to recent work, resemble some aspects of brain biology. “They’ve been related to—and shown to improve on—prior models of the hippocampus,” Lampinen says. Nonetheless, they are, like all artificial neural networks, a caricature at best, he adds.
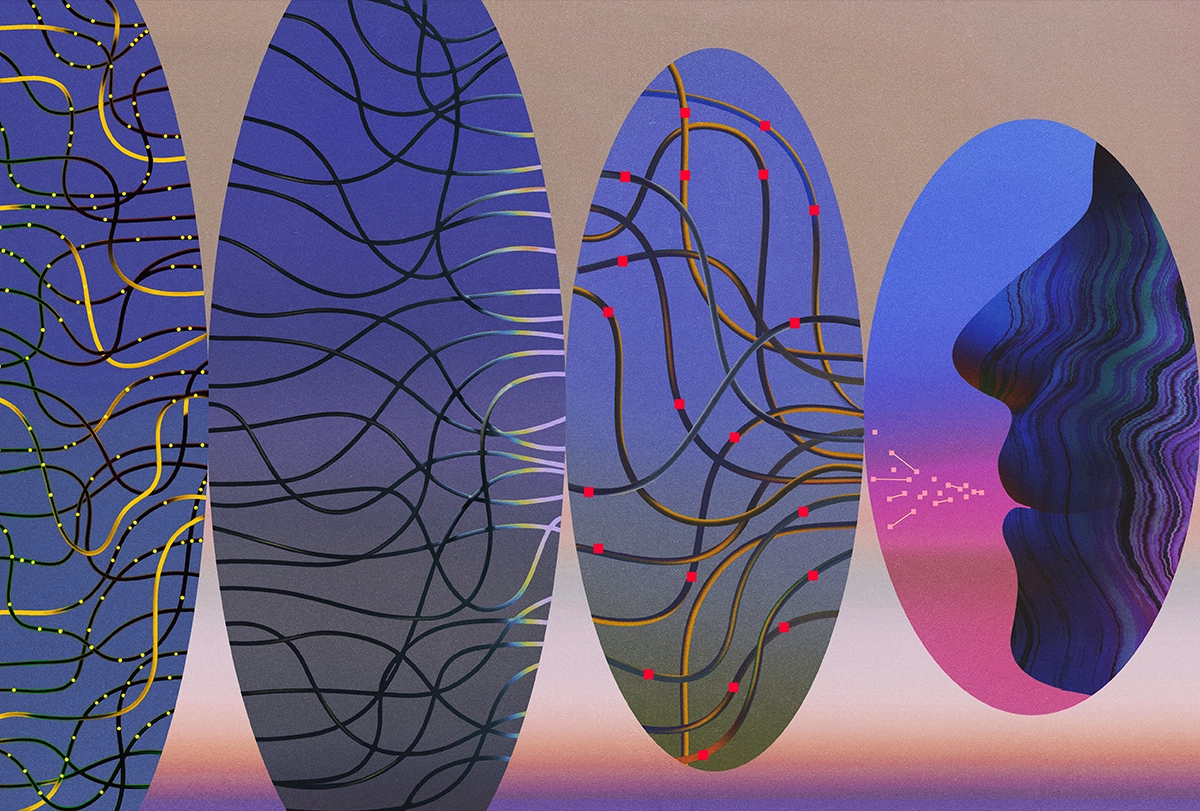
For all that, large language models are uncannily good at simulating the brain. In 2021, Fedorenko and her colleagues began to apply the techniques their vision colleagues had been using for a decade. They trawled the literature for brain responses in people reading and listening to sentences, measured using fMRI imaging or intercranial electrodes implanted for epilepsy. They trained a battery of different language models on those same sentences, and created a mapping model between human and machine neural activity. And they discovered that the networks not only produced humanlike text, but did so in a broadly humanlike way. Among the various systems they tested, GPT-2, a precursor of today’s ChatGPT, was especially adept at mimicking people. GPT is, at its most basic, a fancy autocorrect algorithm that predicts what word should come next, based on what came before. Our brains’ language areas may do the same, the researchers concluded.
In other ways, too, the differences between brains and machines are not as significant as they appear, work from Fedorenko and her colleagues shows. A common argument in support of the idea that these models must learn differently from humans is that they require so much more data. But in fact, large language models achieve proficiency after about 100 million words, comparable to what a child hears by age 10, according to work last year from doctoral student Eghbal Hosseini in Fedorenko’s lab. But what if a network were trained in a more staged way, like a child, instead of dumping the whole internet on it? “You don’t talk to a 1-year-old about the general theory of relativity or whatever, or transformers, for that matter,” Fedorenko says. “You start by talking about simple concepts and simple ways; you give simple, short sentences.” A more realistic educational strategy might make systems that are even better reflections of humans.
B
rain-Score.org now ranks language models as well as vision ones. Stacking models up against one another—another tactic borrowed from visual neuroscientists—has shed some insight on when existing systems better reflect humans. Fedorenko and Hosseini, for instance, devised “controversial stimuli”—sentences for which different models produce different representations. “You’re trying to construct a set of stimuli that allow you to tease these models apart,” she says. The good news is that they found a lot of such stimuli. The bad news was that none of the models matched the human response to those sentences. “We kind of found a ‘blind spot’ of the models,” she adds.One possible conclusion was that the models should all be thrown out, but Hosseini dug deeper. He constructed a set of uncontroversial stimuli, on which the models agreed. And he found these did match human data. So, when the models agreed with one another, they also agreed with human data, and when the models disagreed, they also disagreed with humans. “We were, like, OK, maybe we’re on to something here,” Fedorenko says. “Maybe it’s not just a bad experiment.” She hopes these correlations will let them isolate what makes a model work well, or not.
Having established that large language models are not half-bad at representing the brain’s language processing, Fedorenko and other teams are seeking answers to the puzzles that fill their textbooks. For instance, when we parse a sentence, does the brain rely mainly on formal grammatical structure, or does it consider the meaning of words? For a paper published in April, two of Fedorenko’s graduate students fiddled with sentences in various ways to see whether they affected models’ match to brain data. In this work, they were not giving those rearranged sentences to humans, but simply using the humans as a reference point to study what was happening inside the model.
Minor changes such as dropping “the” or swapping successive words had little effect, they found. Those changes may violate grammatical conventions, but didn’t touch the meaning of words. But mangling a sentence in a way that did affect meaning, such as changing nouns and verbs, had a big impact on the models. For example, take the well-known sentence containing all 26 letters, “The quick brown fox jumped over the lazy dogs.” A lightly perturbed variant is, “Quick brown fox jumped over lazy dogs.” Clearly, our brains form the same mental image from the perturbed sentence as from the original. The model does the same, the researchers found. The representation it forms evidently encodes a meaning that is sufficiently high-level as to be insensitive to the little words.
But if you enter the variant, “The quick brown jump foxed over the lazy dogs,” the model deviates from the human data, indicating that it is producing a vastly different representation from before. Structurally the sentence hasn’t changed—it is still <article> <adjective> <adjective> <noun> <verb> <prepositional phrase>—so the model must be relying on additional, semantic information: that a fox can jump, but a jump can’t fox. “It’s all, in part, contra this view that has been advocated by people from the Chomskyan generative grammarian program, which has long emphasized syntax as the core [of] everything about language and meaning is just kind of secondary,” Fedorenko says.
A huge challenge of this field is to dissociate language from the rest of cognition: logic, social cognition, creativity, motor control, and so on. Large language models don’t (yet) have all of that. Although they do have a vast memory and some reasoning capacities, whereas plugins or special-purpose modules provide some of those other functions, they are mostly still just models of the language areas of the brain—something that you have to keep reminding yourself as you use ChatGPT and other systems. When they hallucinate information, it is not their failing, but ours: We are forcing them to answer a query that is outside their narrow competence. “One of the things we’ve really learned from the last 20 years of cognitive neuroscience is that language and thought are separate in the brain,” Kanwisher says. “So you can take that insight and apply it to large language models.”
As fraught as that can be for people expecting to get reliable information from these systems, it is also their great power for neuroscience—as many at the NYU meeting noted. They are close enough to the human brain to let neuroscientists make direct comparisons, yet distinct enough to help them look beyond the human example and search for the general principles governing perception and intelligence. Already these systems suggest that intelligence is universal—not limited to humans or even to other mammals but found whenever cognitive systems have enough computational power to extract the salient features of the world they have been exposed to.
Recommended reading
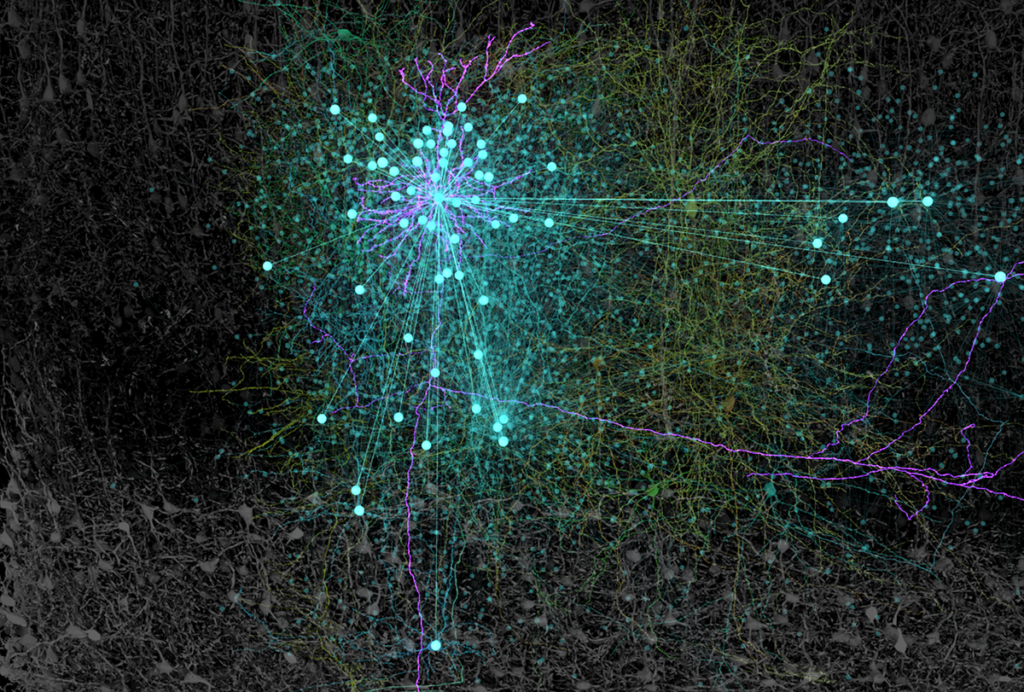
What are recurrent networks doing in the brain?
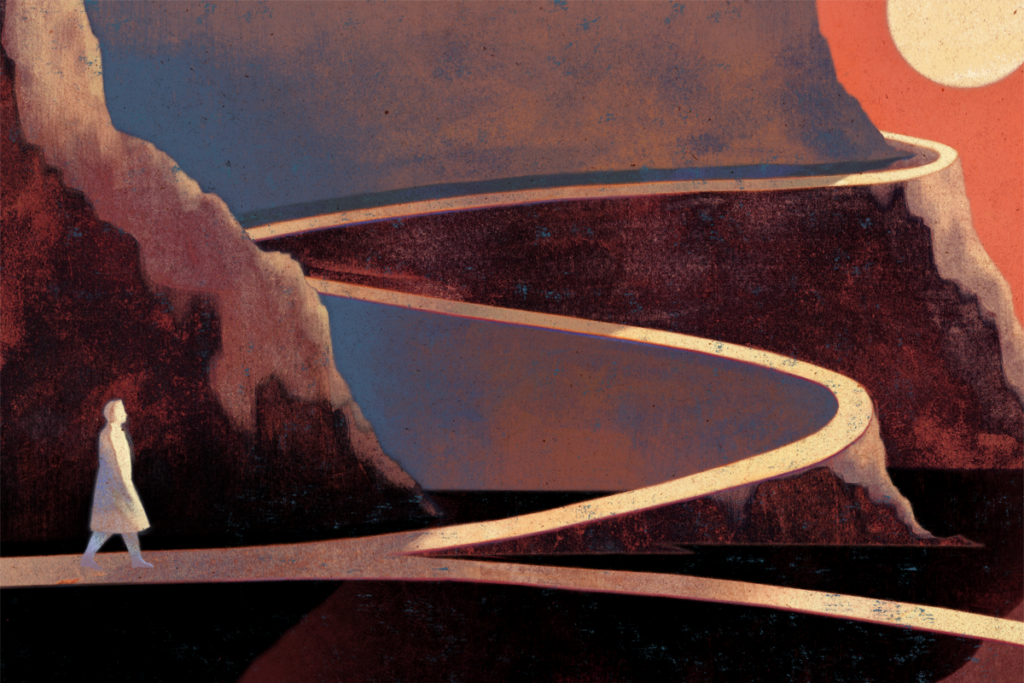
Static pay, shrinking prospects fuel neuroscience postdoc decline
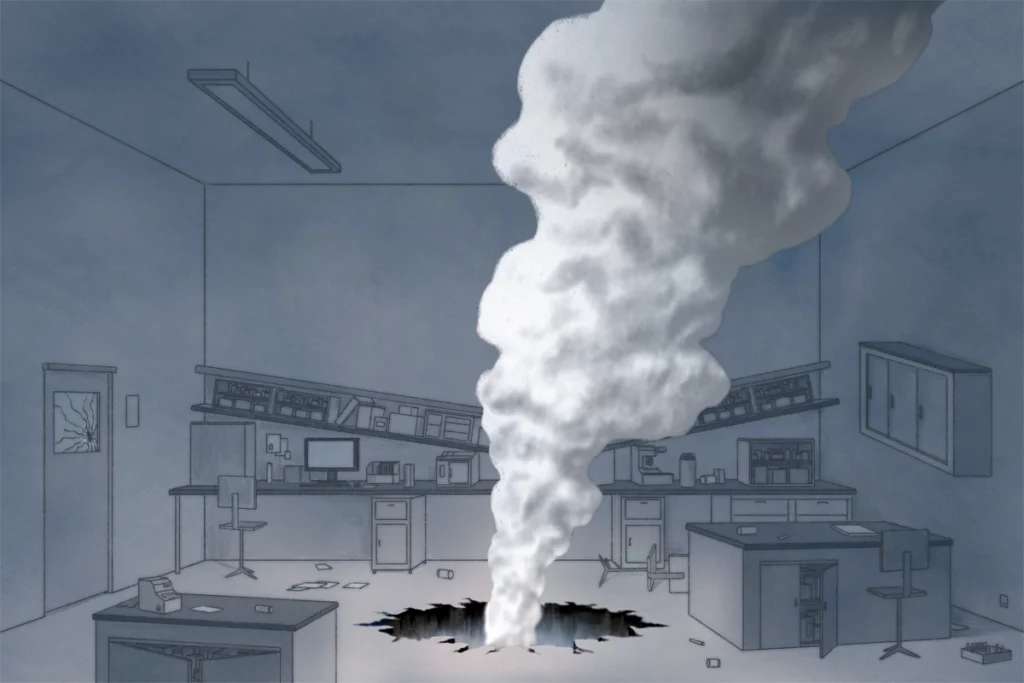
A scientific fraud. An investigation. A lab in recovery.
Explore more from The Transmitter
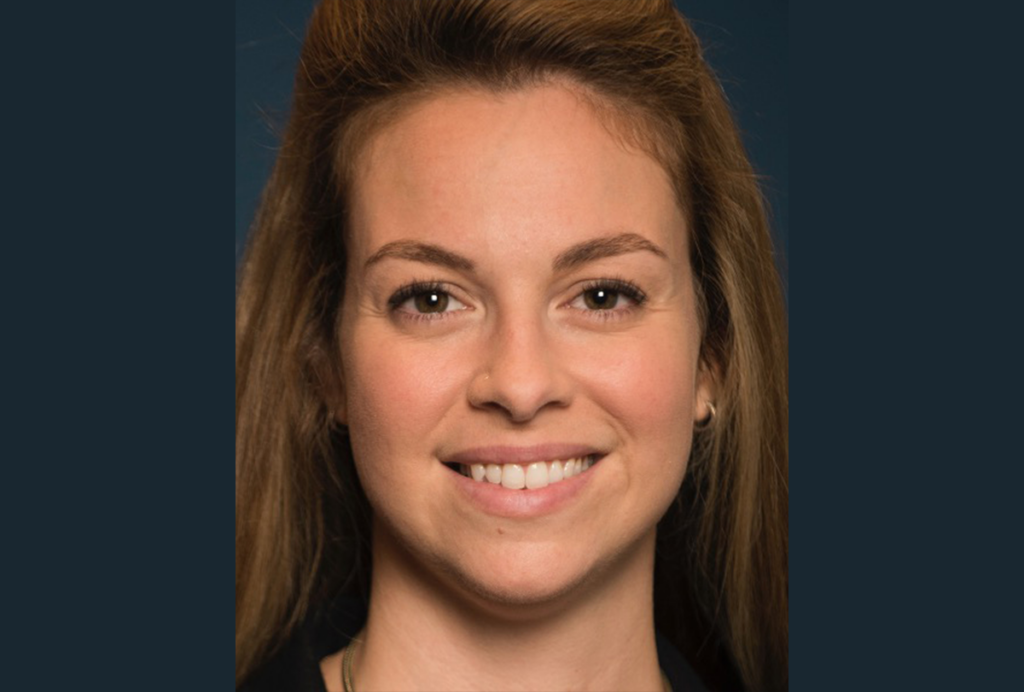
Autism program chief among National Institutes of Health layoffs
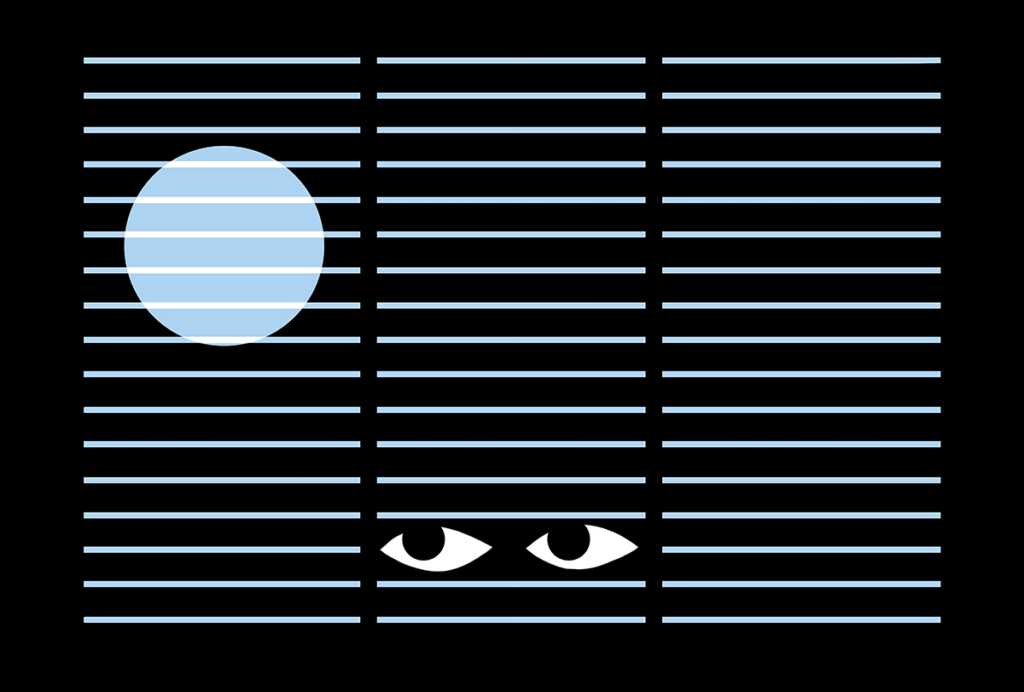
This paper changed my Life: Bill Newsome reflects on a quadrilogy of classic visual perception studies
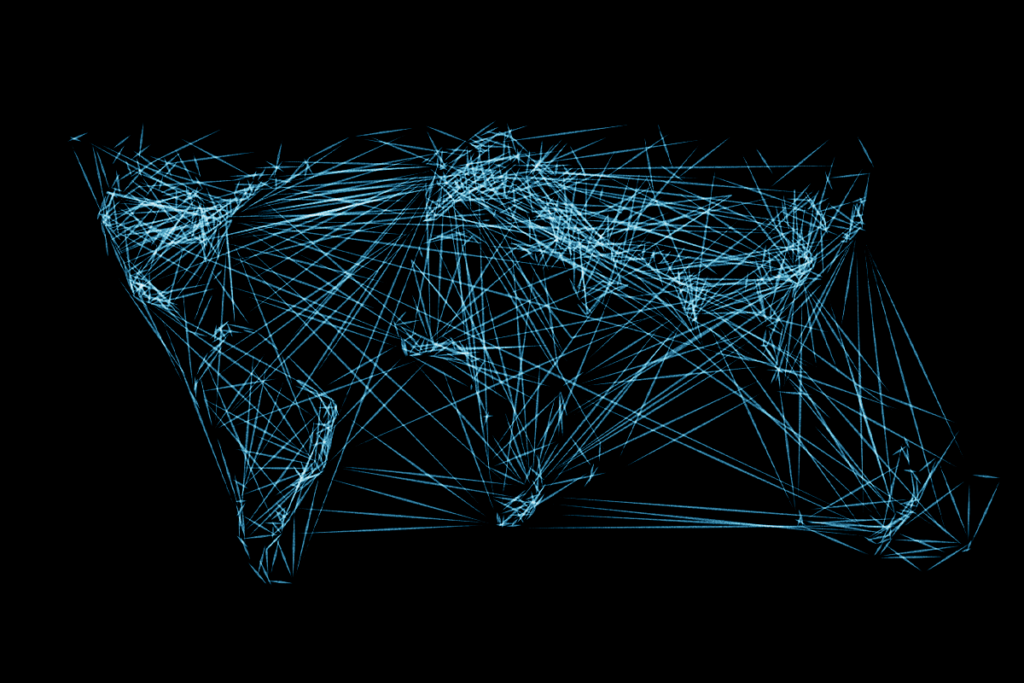