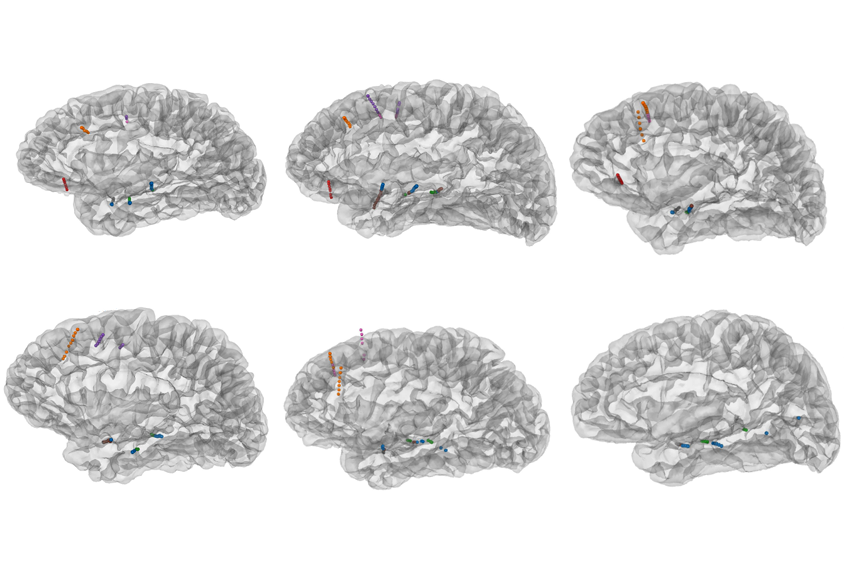
AI model helps decode brain activity underlying conversation
A text-predicting chatbot parses text from conversations in a way that parallels brain-activity patterns associated with speech production and comprehension.
Dialogue is a semi-choreographed dance — a sequenced exchange in which each person must first comprehend the words of their speaking partner and then plan and execute a response. These steps — understanding language, producing speech, and the transitions in between — have distinct brain-activity patterns, according to a new preprint, the first study to use implanted electrodes to record activity in people’s brains while they engage in natural conversation.
“Our major contribution to the field is that these are natural conversations,” says study investigator Jing Cai, instructor in neurosurgery at Harvard Medical School.
Although some studies have explored what happens in the brain when a person listens to speech, and to a lesser extent when they speak, brain goings-on during unrestricted, natural conversation are almost wholly unmapped — largely because the tools to track it are only now emerging, says Sydney Cash, associate professor of neurology at Harvard Medical School, who co-led the study. “The ability to do this is just catching on.”
The way the brain processes linguistic information is strikingly similar to how a natural-language-processing (NLP) model computes the same verbal exchanges, the study also shows. NLP models are the type of artificial-intelligence model used in chatbots such as ChatGPT to determine the intended meaning of text and generate responses.
The result suggests that combining NLP with brain data analysis may provide a powerful avenue for studying language, says Christian Herff, assistant professor of neuroscience at Maastricht University in the Netherlands, who was not involved in the work. What’s more, “including semantic information about speech in these algorithms might also improve accuracies.”
I
To home in on the slice of brain activity that reflects the semantic aspects of the conversation rather than, say, the acoustic or motor components of speech, the researchers also ran the text of the same conversations through an NLP called GPT2. The NLP was pre-trained to process language through a layered structure, in which the layers are essentially a series of processors. As in previous work, the different layers turned out to process different aspects of information — with the lower layers focused more on word-level aspects of language and higher layers geared more toward contextual and thematic aspects.
Across different brain areas, about 10 percent of activity captured by electrodes during conversation correlated strongly with activity in the artificial neurons, or nodes, in the NLP model, which are organized by layer. Among this subset of brain signals, ones that corresponded to understanding and planning speech correlated with higher layers in the NLP, and ones that corresponded to saying individual words correlated with lower layers. “Our results showed remarkable differences” in NLP layers that govern speech production versus speech planning and comprehension, Cai says.
“We don’t want to imply that this particular [NLP] model is a replication of neural activity,” he says. “It’s rather that the general form of that processing seems to have parallels in both the humans and the machine.”
The researchers’ analysis of brain activity showed that different electrodes across many brain areas were active depending on whether a participant was speaking or listening. “Overall, we found very few electrodes that are showing selectivity to both speaking and listening. They are pretty well separated,” Cai says.
The EEG recordings also captured a difference in brain activity between these two conversational modes. Signals tended toward lower frequencies when a participant was just about to speak but toward middle frequencies when they were listening.
Lower frequencies are linked with top-down processing (in other words, starting with more conceptual information and trickling down to sensory and motor features), previous work has suggested, and higher frequencies reflect bottom-up processing, Cai says. “Our hypothesis is that for speaking, we know what we want to say and are broadcasting this signal to the motor region and to the whole brain, whereas for listening we need to have the sensory [input].”
R
Last month, another team trained an NLP on fMRI brain scans of people listening to podcasts. The resulting machine partially decoded thoughts into words and raised the specter of mindreading.
But so far, those and other efforts have involved only language comprehension, not production, Herff explains, because facial movements people make when they speak generally skew the recordings. An invasive approach piggybacked onto clinical need, such as the one Cash and his colleagues used, “I think is the most ethical way of doing it,” Herff says.
There is no doubt that two people in conversation change or align with each other’s brain activity, says Emily Myers, professor of speech, language and hearing sciences at the University of Connecticut in Storrs. Cash and his colleagues’ approach might be able to monitor those shifts. “There are really interesting questions to ask about how those dynamics align with each other and sync up with each other,” she says.
Cash says he hopes the group can build on the technique they used and look beyond the semantic aspects of language to explore some of the other dimensions. For example, the visual or emotional context of a conversation, or the facial expressions and timbres that occur during it, all contribute to meaning. Understanding their interplay in conversation could point to interventions for people with autism or other communication issues, he says.
Recommended reading
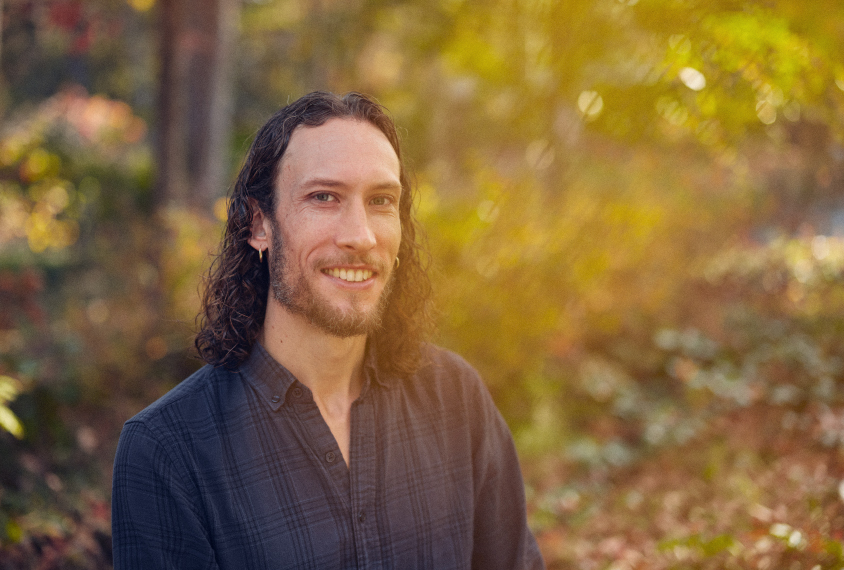
Asleep in the Mouse House with Graham Diering
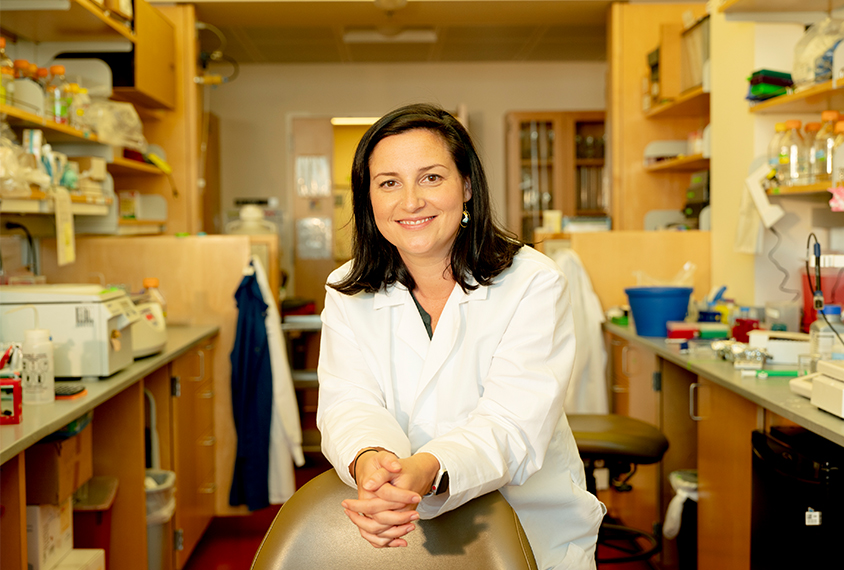
How Helen Willsey broke new ground, frogs in hand
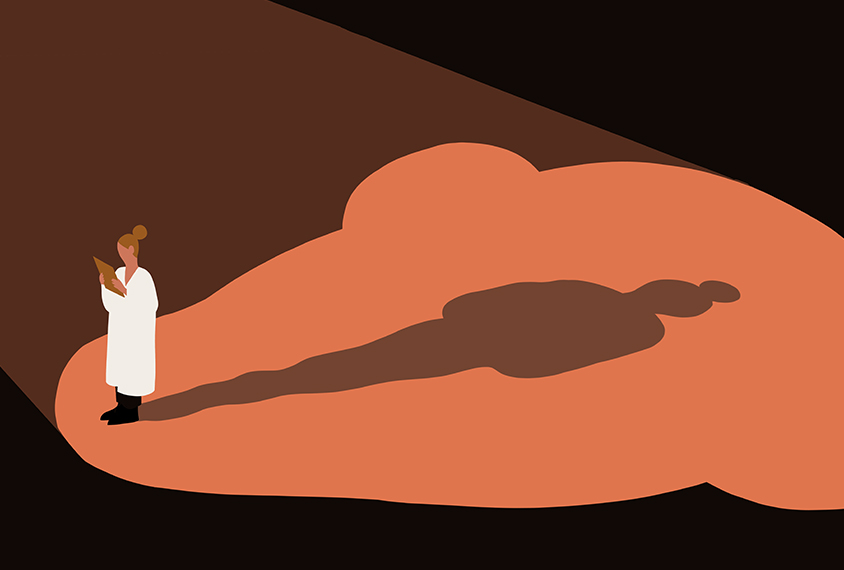
Why monkey researchers are seeking the spotlight: Q&A with Cory Miller
Explore more from The Transmitter
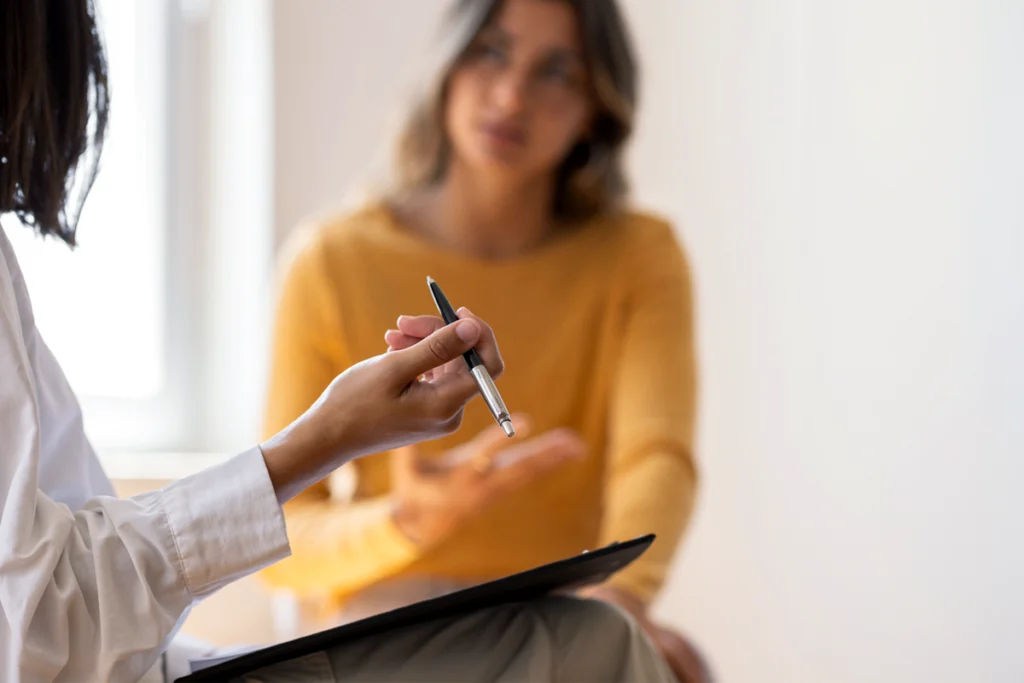
Autism prevalence increasing in children, adults, according to electronic medical records
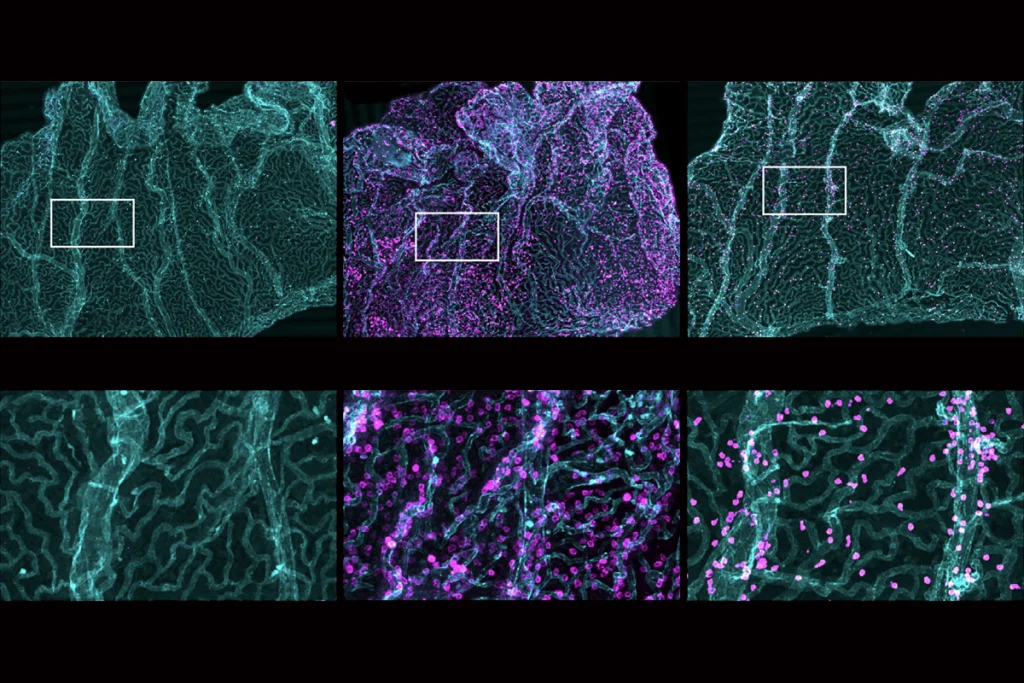