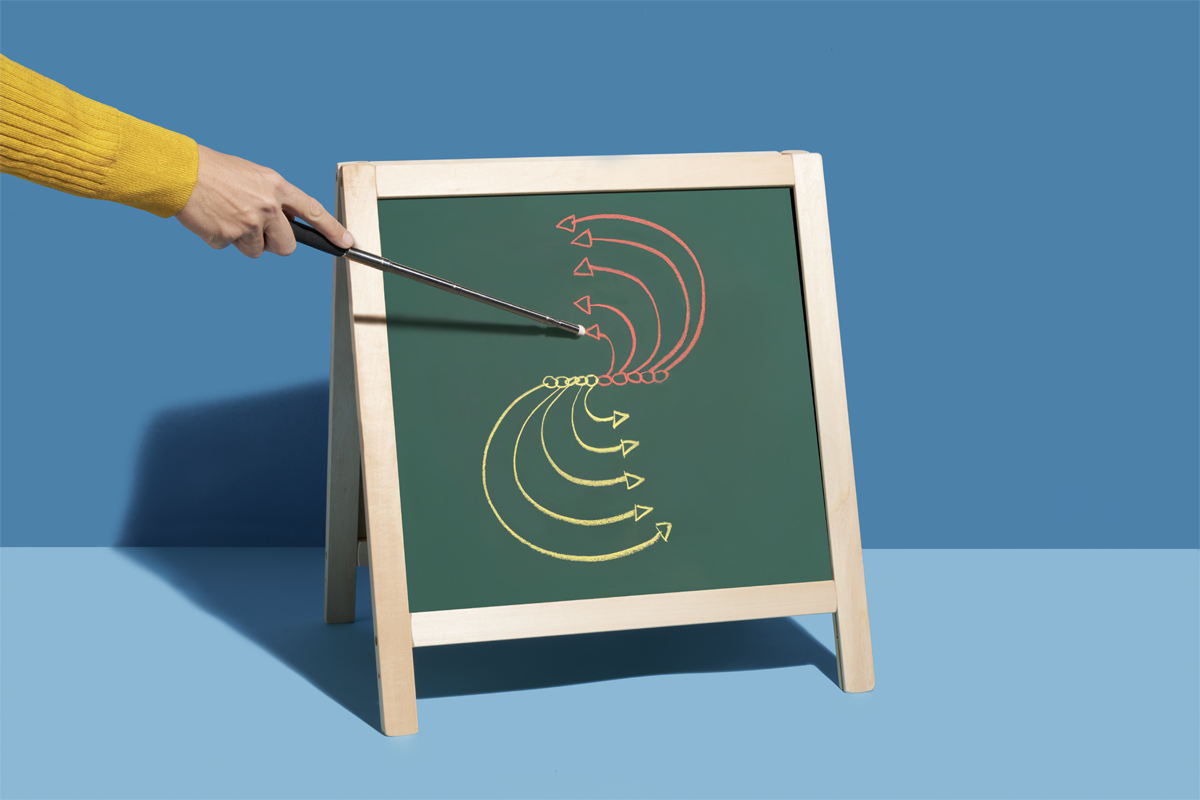
How to teach this paper: ‘Neural population dynamics during reaching,’ by Churchland & Cunningham et al. (2012)
This foundational paper, with more than 1,500 citations, is an important departure from early neuroscience research. Don’t be afraid of the math in the first paragraph.
With almost 1,500 citations, “Neural population dynamics during reaching,” published in Nature in 2012, is a foundational paper in systems and computational neuroscience. It presents the first evidence as well as a thorough theoretical footing for the idea that populations of neurons generate movement in a rather stunning way: with dynamics that rotate. This paper would be a great fit for systems or computational neuroscience classes, or an interesting applied example for a mathematics class. Given its focus on movement, it would also be a great choice for a class focused on motor systems or disorders of movement and could be tied to a discussion about brain-computer interfaces.
This paper is an important departure from early neuroscience research, which focused on individual neurons that represented information by changing their firing rate. Such neurons — largely studied in the visual system — were tuned to specific features, such as the orientation of a black and white bar, a direction of movement, or even pictures of Jennifer Aniston. But for a long time, movement researchers struggled to find neurons that reliably responded to only one feature of a movement. In the visual cortex, each neuron had a role; in the motor cortex, each neuron seemed to be doing everyone else’s job.
That’s where Krishna Shenoy’s team came in. Shenoy turned to more complex computational methods to help translate the neural activity in the motor cortex. Specifically, he championed a class of “dynamic” methods that ultimately became central to understanding how the brain produces movements and are the heart of “Neural population dynamics during reaching.”
Paper overview
A great pedagogical feature of this paper is that it first takes a broad look at the neural activity underlying different movements as a means of pointing readers to a surprising observation: Neural population activity looks similar for different kinds of movement. Some movements, such as crawling, are visibly rhythmic, so this is a nice entry point to understand why movements may be generated by oscillating neural activity. By focusing first on the leech example given in the paper (perhaps bolstered by William Kristan’s papers cited in that section), students can develop an intuition for what “rhythmic” means in this context.
From there, we can make the extension to oscillating firing rates in different kinds of movements, such as walking or reaching. Although these movements aren’t oscillatory, their neural population response is — weird. Mark Churchland, John Cunningham and their colleagues use this observation to motivate a deep dive into more data. Shenoy gave an elegant, convincing talk about this transition from representational frameworks to dynamic systems in 2013, which I strongly recommend watching for background.
As an instructor, you might choose to stop at the first figure; it is rich enough in theory and analysis, yet very simply diagrammed (and with a cute leech drawing). But figures 2 through 5 show raw electrophysiology (single- and multi-unit arrays as well as electromyography) data from monkeys as they are reaching, which can be useful for students to see. They also illustrate another unexpected feature of the data: Rotations don’t seem to depend on the actual movement direction and don’t relate to the actual reach path of a monkey.
As the finale, the paper presents a model that illustrates that activity created by a generator model (which emulates a dynamical system) is more closely matched to the neural data than are EMG or two other kinds of simulated data models, which only take into account the features of movement, such as velocity, acceleration or direction. There is a lot packed into the final figure; as an instructor, how much you explain these models depends on your own comfort level and how relevant they are to your course. For most neuroscience courses, it’s sufficient to say that when you simulate data, you’re doing so because it allows you to generate activity with known properties or that can perform a particular task, so that you can see what happens when you pass such data through your analysis. For the Churchland paper, the only data that produce rotational dynamics are the neural data that the researchers have recorded and the generator model. In later work, David Sussillo, Churchland, Matthew Kaufman and Shenoy showed that a recurrent neural network would also spontaneously adopt brain-like, quasi-oscillatory patterns.
Sticky points
Oh hello, math.
This paper does something that is either a very bold move for a neuroscience article or a very big faux pas, depending on your relationship with math: It includes equations in the first paragraph. From a student perspective, this can be quite daunting and an immediate reason to think, “There’s no way I’m going to understand this paper.” (Most biology students fear math.) But educators and students alike should not fear the math in this paper. Those equations are actually saying something very simple.
Let’s start with Equation 1, which serves as the null hypothesis of the paper. It represents the prevailing idea in the field: A single neuron represents different features of a stimulus. In this equation, a neuron’s firing rate is predicted by some combination of the parameters of a stimulus. For a limb movement, this might be the intensity of muscle contraction or the direction of the limb. As an instructor, it is up to you whether you would like to explain the nuances of this equation, and it may be a nice opportunity to review how mathematicians speak.
The second equation is the dynamical system equation, and even though it’s shorter, it’s conceptually more complex. Now we’re trying to compute the firing rate of all neurons (the population code). The derivative of the population code is determined by some unknown function that takes into account the population activity, plus some external time-varying input.
The final equation, found at the end of the results, is similar to Equation 2, but written in the spirit of linear algebra. Given the observations in this paper, the authors conclude that just one matrix can capture the dynamics of neural activity underlying reaching, regardless of the properties of the reach.
How much you dig into this math depends a bit on the scope of your course — although dynamical systems is the theoretical backdrop, it’s not central to understanding the concepts of this paper. If you or your students desire a deeper dive, the obvious choice is the book “Dynamical Systems in Neuroscience” (2010).
Dimensionality reduction
Beyond interpreting the equations in the paper, there is also the big, flat, and rotating elephant in the room: dimensionality reduction. The paper, and this entire body of work, uses a version of principal component analysis (PCA) to project population activity into a lower-dimensional space where rotational features can be seen and measured. Unless you’re teaching an upper-division computational neuroscience, modeling or mathematics course, you’re probably not going to dive into the math behind PCA. That said, I think it’s entirely possible to build an intuition for it using graphical explanations, such as the one below. Cunningham and Byron Yu, a neuroscientist at Carnegie Mellon University in Pittsburgh, Pennsylvania, also have a very accessible review article on the topic, and Neuromatch Academy has a dimensionality reduction tutorial with videos and code, as well as a tutorial on discrete dynamical systems.
The scientists behind the paper
Krishna Shenoy, the principal investigator behind this paper, made major contributions to the fields of neural dynamics and brain computer interfaces. He died of pancreatic cancer earlier this year. If you would like to learn more about him and his work more broadly, please read “A scientist’s quest for better brain-computer interfaces opens a window on neural dynamics” or this obituary by Mark Churchland and Paul Nuyujukian.
Shenoy’s team originally wrote the paper with the model first, but after leaving many people quite puzzled at their well-attended Society for Neuroscience conference poster, they reworked their story. Churchland and Cunningham rallied at the benches outside of the conference, staring over San Diego Bay, and decided they needed to reverse the order: unexpected results first, model second.
Now, 11 years after the paper’s publication, Churchland reflects fondly on the ideas they presented and how they shifted the field:
“To me, the real beauty of the original idea is that it made sense of a bunch of diverse facts, including otherwise-confusing features of single-neuron responses. The hypothesis thus had appeal to me long before we were able to test that final prediction … At that time, it took a long time to get someone to understand both the essence and the appeal of the hypothesis. They were only willing to invest the time if they saw a novel result, presented first, that demanded an explanation.”
This paper was, at its core, a team effort, and not only between the seven authors. Churchland also credits Larry Abbot and Evan Schaffer with helping to develop the ideas of the paper, as well as the approach to sharing it with other scientists.
Future lessons
This paper spawned an entire field of scientists looking at the rotational dynamics in not just motor systems, but also cognitive ones. Ultimately, many still wonder why these dynamics are there, and what their role is. If you’re looking for some food for thought on the purpose of these rotations, see my article “Discovery of rotational dynamics.” This could also open up an interesting discussion about the links between structure, function and computation.
One great way for students to engage with this paper is to work with similar data themselves. This interactive notebook provides students with an introductory implementation of PCA using Python, and Yu has developed a problem set for MATLAB. Finally, Churchland and others have since built on this work, asking questions about what happens when trajectories of networks get tangled, and he spoke about it in 2020 in a lecture at the Bernstein Center for Computational Neuroscience in Munich, Germany.
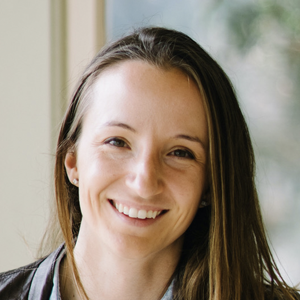
Ashley Juavinett
University of California, San Diego
Recommended reading
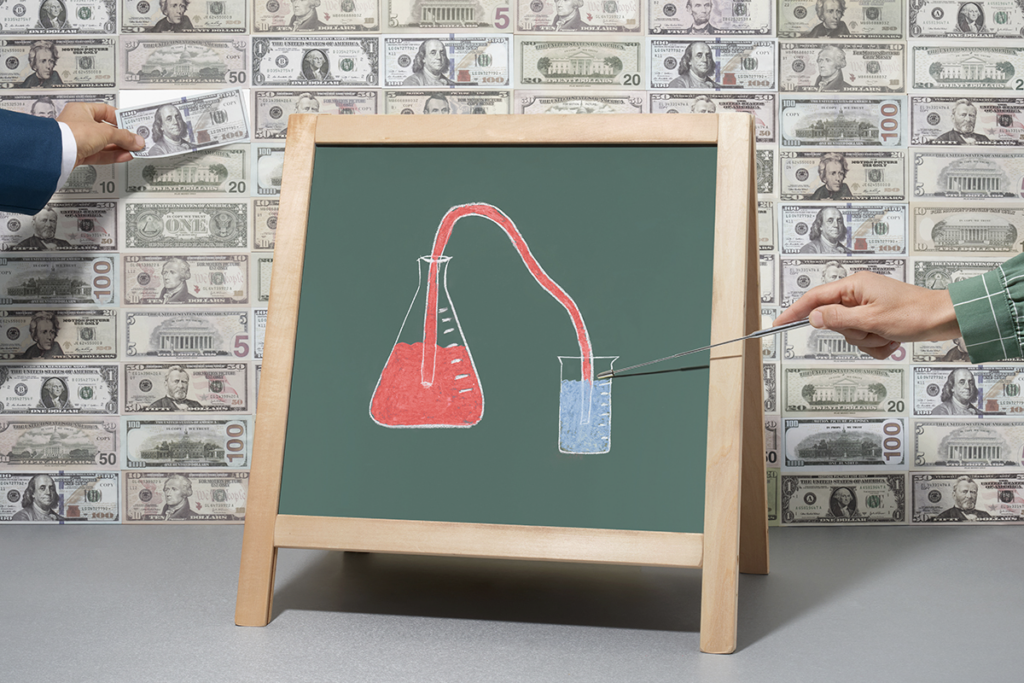
How to teach students about science funding
Explore more from The Transmitter
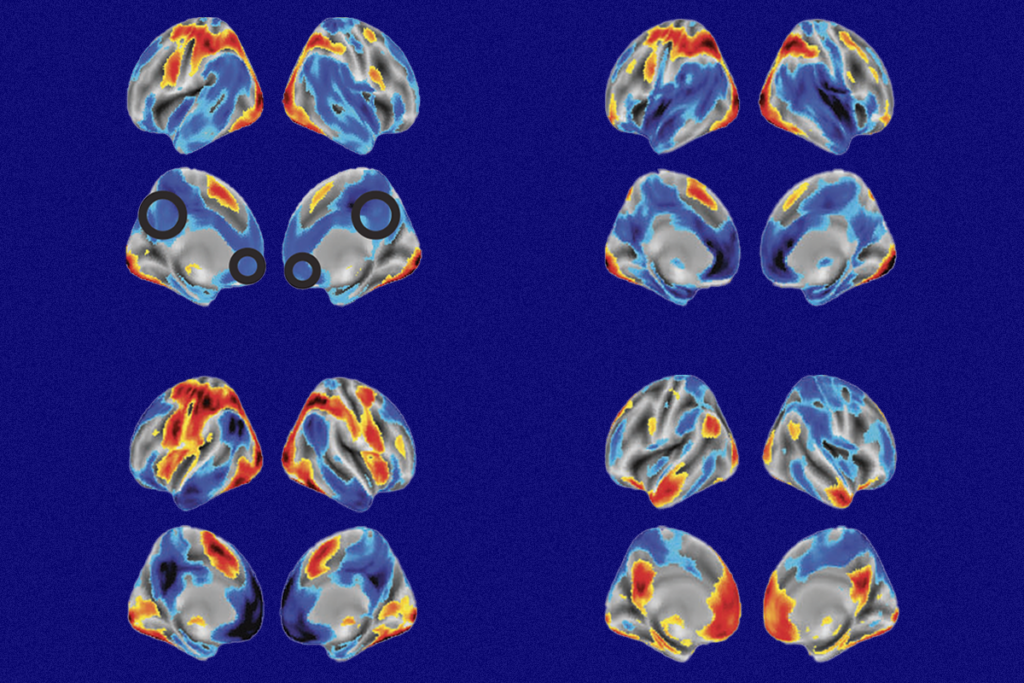
During decision-making, brain shows multiple distinct subtypes of activity
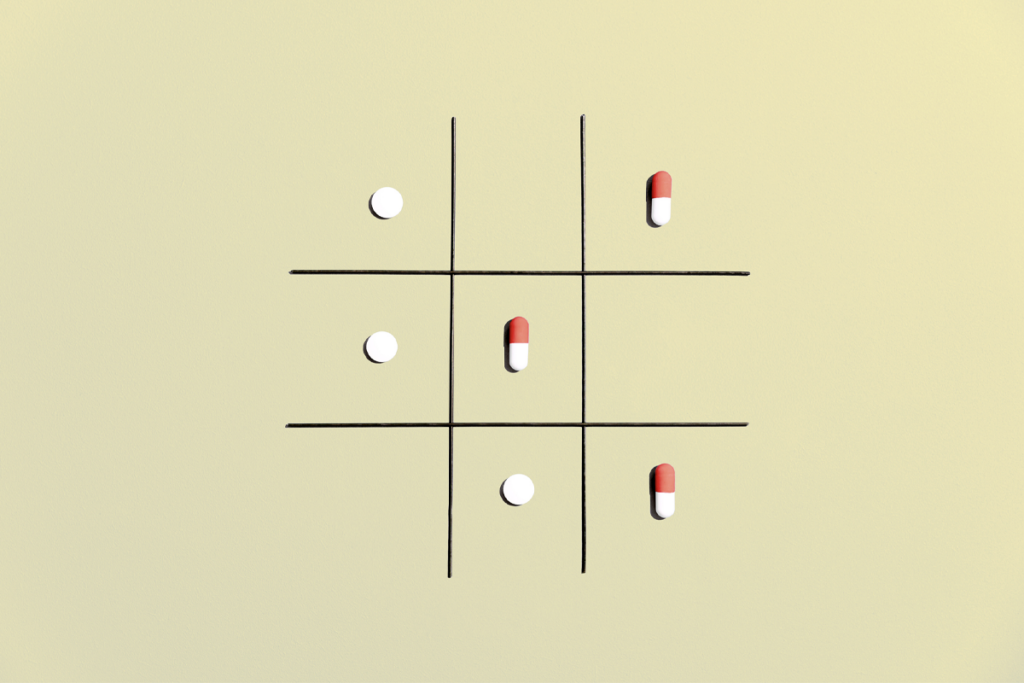
Basic pain research ‘is not working’: Q&A with Steven Prescott and Stéphanie Ratté
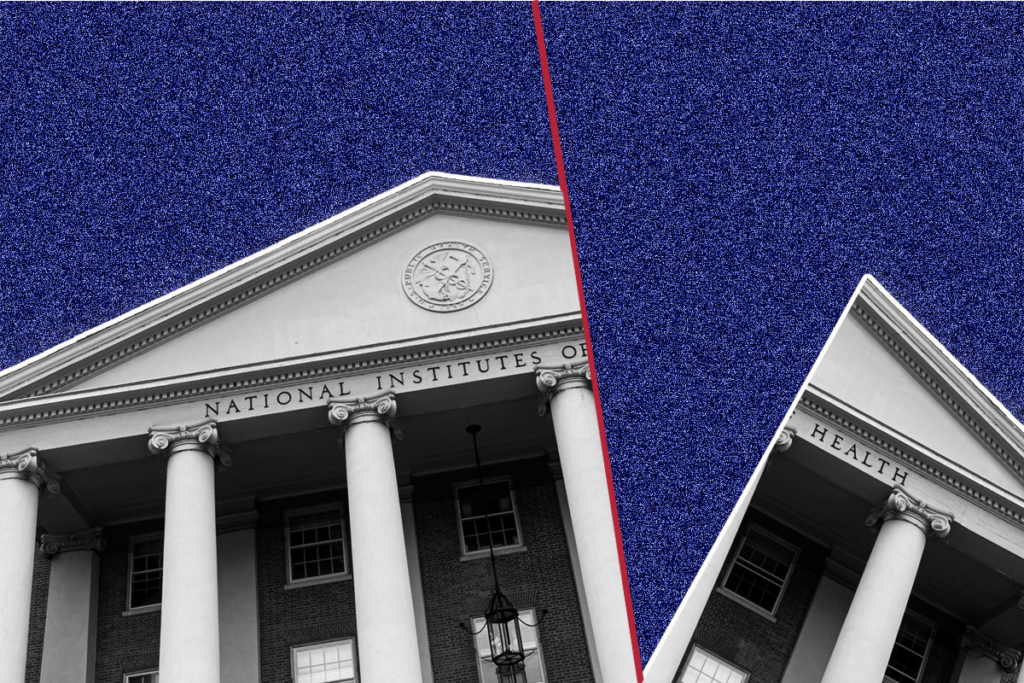