From bench to bot: How important is prompt engineering?
To draft the most effective prompt, assume the stance of teacher.
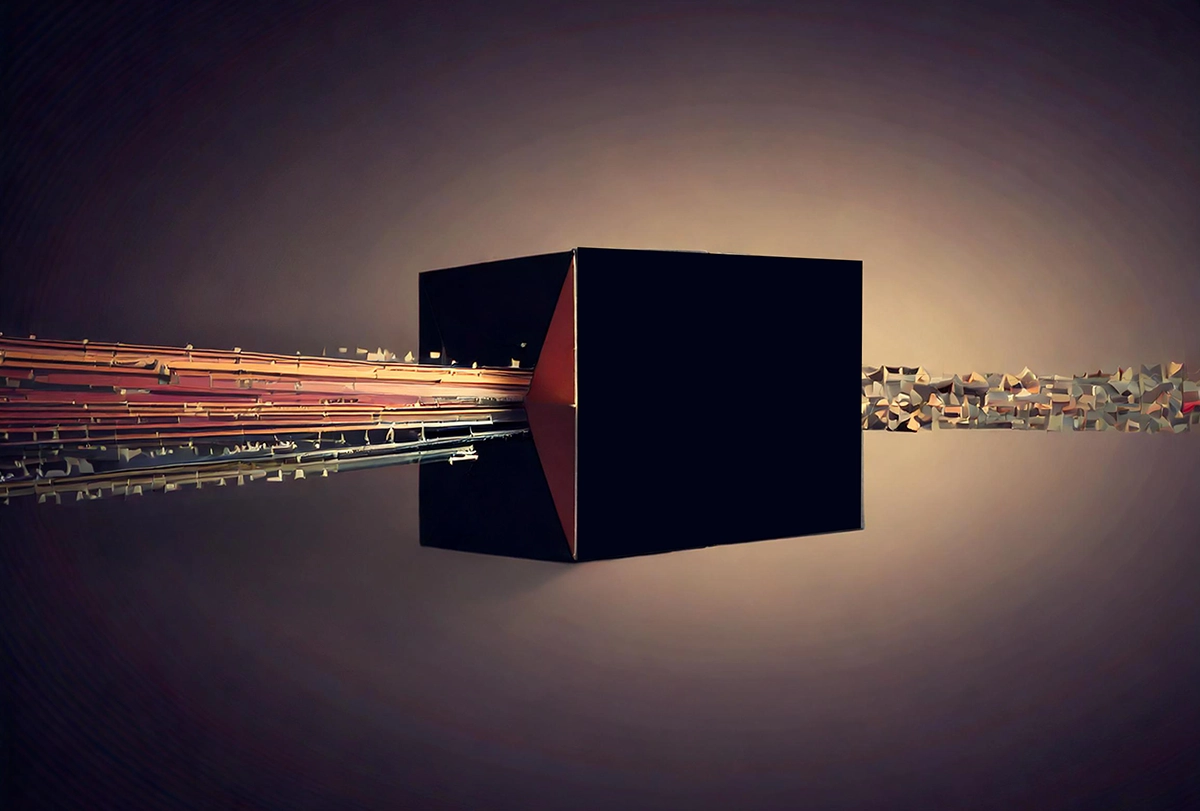
Shortly after ChatGPT hit the public consciousness, The Atlantic declared that prompting, or interacting with artificial intelligence to get the response you desire, will be “The Most Important Job Skill of This Century.” Companies offer salaries along the lines of $300K for “prompt engineers.” And a new species of internet influencer, the prompt guru, emerged to clutter social media feeds with impossibly complex instructions for AI chatbots guaranteed to “10x productivity.” Although it’s true that “prompt engineering” is a real skill, especially for highly specialized use cases, all this emphasis on prompting seems at odds with the central selling point of generative AI chatbots: that you can, well, just chat with them in plain language.
Yet coaxing AI to produce useful output seems to come more intuitively to some than to others. In my work helping scientists navigate AI, I notice that frustrated users search maddeningly for the secret syntax that will unlock AI’s elusive wizardry. This is understandable; generative AI chatbots are a new and poorly characterized technology, so it’s often unclear in a given instance whether a suboptimal output is due to inherent limitations of the technology or just a suboptimal prompt. But the exact words you use to prompt AI probably matter less than you think. Though there are a few evidence-based tips (more on those below), they aren’t that complicated—and for the typical scientist’s writing needs, no advanced technical skill is required. In fact, some of the most successful users don’t really interact with AI like it’s a piece of technology at all. They treat it instead like it’s a talented but naive and slightly untrustworthy person.
Let’s say you’re drafting a job description for a technician in your lab. This is a suitable task for AI assistance for two reasons: It’s somewhat time-consuming but relatively low stakes, and you are the authority on whether the output is accurate or not. If you approach the task like this—
“Write me a job description for a tech in a neurophysiology lab.”
Link: https://chatgpt.com/share/670931b2-6668-8002-b492-611bf94f0feb
—you’ll get a reasonable job description, but probably not the one you need. The prompt doesn’t provide any details about the role, leaving the AI to make assumptions. You’ve given little guidance on structure or key elements. In general, I’ve found that requesting a fully written document from a simple prompt is usually too much to ask of AI. This type of broad, unspecific prompt is more likely to produce content that requires extensive editing or rewriting, potentially creating more work than it saves.
A better approach would be to think about what you’d ask a human “naive collaborator” to do. You might give it more specifics about the role, a description of your lab’s work or a sample job description and ask it to adapt it to your lab. Any of those approaches would work better than a simple prompt. Take something like this:
“Your task is to write a job description for a Lab Technician in a neuroscience lab at Columbia University.
The format must be as follows:
[[PASTE IN AN EXAMPLE JOB DESCRIPTION HERE]]
Follow this format, but adapt it for a neuroscience lab that studies electrophysiology in animals. Responsibilities include managing mouse colonies, performing surgeries for in vivo and in vitro experiments, histology, confocal imaging, electrophysiology recording, and coordinating the day-to-day operation of the lab. Preferred qualifications are bachelor’s or master’s degree in neuroscience, biology or a related field and prior lab experience.”
Link: https://chatgpt.com/share/670938b1-a940-8002-b451-cc50cc30e155
This prompt demonstrates the “naive collaborator” stance. Rather than just asking the AI to complete the task, consider what a human would need to complete the task. Here, I provided context by explaining the duties, and I clearly outlined the desired structure of the output using an example job description from Columbia’s website. The final product needs some tweaking, but it’s a quick edit rather than a major overhaul. By using AI in this way, you’re leveraging its capacity to execute boilerplate writing without requiring it to divine details about your specific situation.
M
any “advanced” prompting techniques—such as asking the AI to think step by step or to reread, or giving the AI examples of preferred and non-preferred outputs—are strategies you’d use to teach or train a person. What this tells me is: If you get stuck or aren’t getting good outputs, don’t spend hours googling how to prompt and reading technical documentation. Ask yourself what you would do if a student just wasn’t getting it. You might ask them to reread and try again or to work step by step through a complex task. Or you might give them examples of what you’re looking for. These are, essentially, teaching or managerial techniques, not programming techniques. That’s the key shift in your stance toward using AI.Like teaching, prompting takes practice. This repetition, not technical mastery of prompting, is the biggest roadblock for many busy scientists: It requires time, not to mention rejiggering writing workflows that basically already work for you. With all the hype about AI, it’s tempting to hastily turn to a chatbot, throw a vague prompt at it, get a poor result and give up in frustration. But to truly see if AI can benefit you, you need to spend time integrating AI into your routine, even when it’s a bit annoying at first. Yes, it might slow you down initially. But as your intuition for AI’s capabilities improves and you start getting better output, you’ll notice the bots can make certain tasks easier. From time to time, you may even be pleasantly surprised by how good the results can be.
To maximize your success, it’s also crucial to interact with the latest AI models. Some performance issues stem simply from using outdated versions. And for now, it’s also best to access these models through the companies’ own websites. Third-party tools or “co-pilots” can modify your prompts or alter performance in ways that might not always be obvious or beneficial. In the end, as AI gets better, the details of prompting will probably matter even less than they do now—but gaining an intuitive understanding of the technology’s strengths and limitations in the context of your own work will remain valuable. And if you’re ever in a prompting pinch, you can always ask AI to generate the prompt for you.
AI-use statement: Anthropic’s Claude 3.5 (Sonnet) was used during the writing process for editorial feedback. ChatGPT-4o was used to generate the example responses to the prompts.
Data-privacy concerns arise when using standard web interfaces, as user inputs can be adopted to train future AI models, though certain technical workarounds offer more protection. Major journals require disclosure of AI use, and the U.S. National Institutes of Health has banned the use of AI for some purposes. Lastly, although generative AI generally does not pose a high risk of detectable plagiarism, that risk may increase for highly specialized content that is poorly represented in the training data (which might not be much of a concern for the typical user but could be a larger concern for the typical scientist). Some AI systems in development may overcome some of these problems, but none will be perfect. We’ll discuss these and other issues at length as they arise.
Recommended reading
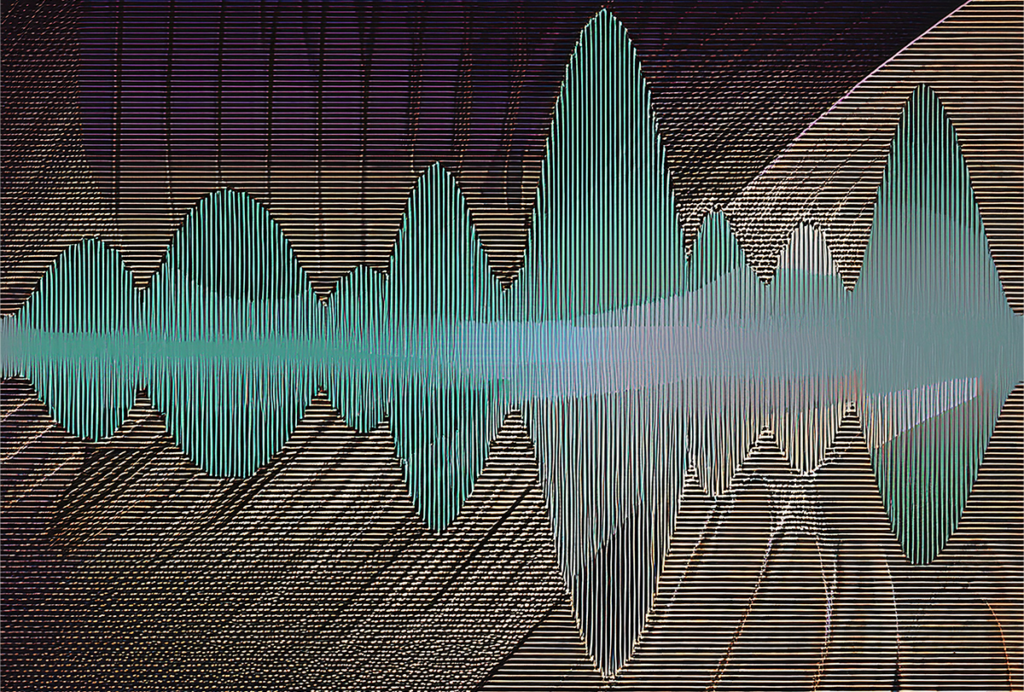
Keeping it personal: How to preserve your voice when using AI
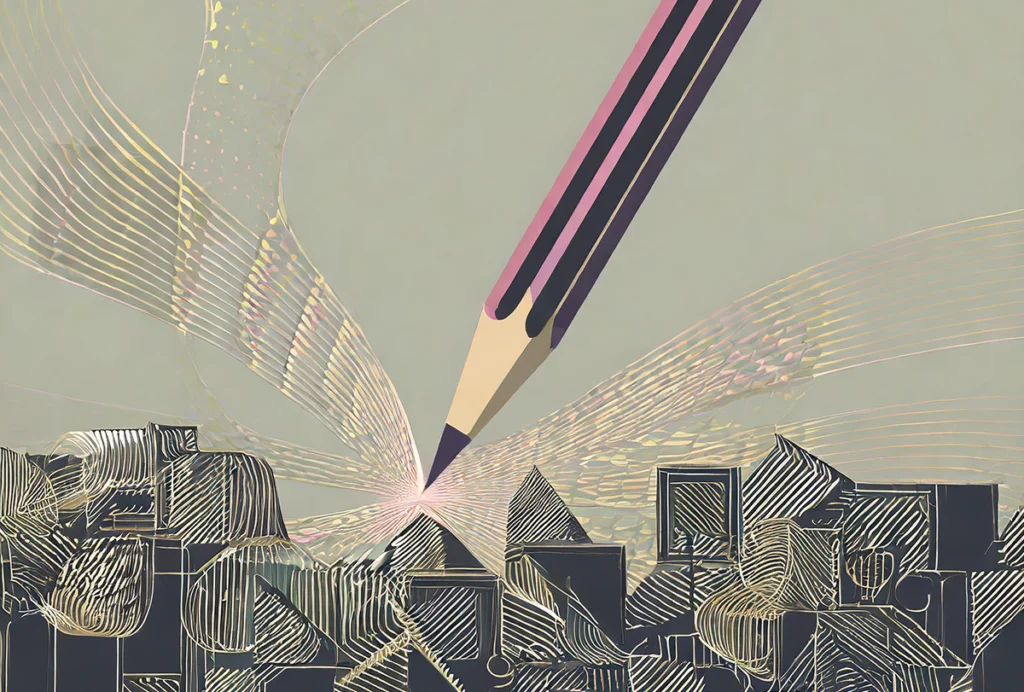
From bench to bot: Does AI really make you a more efficient writer?
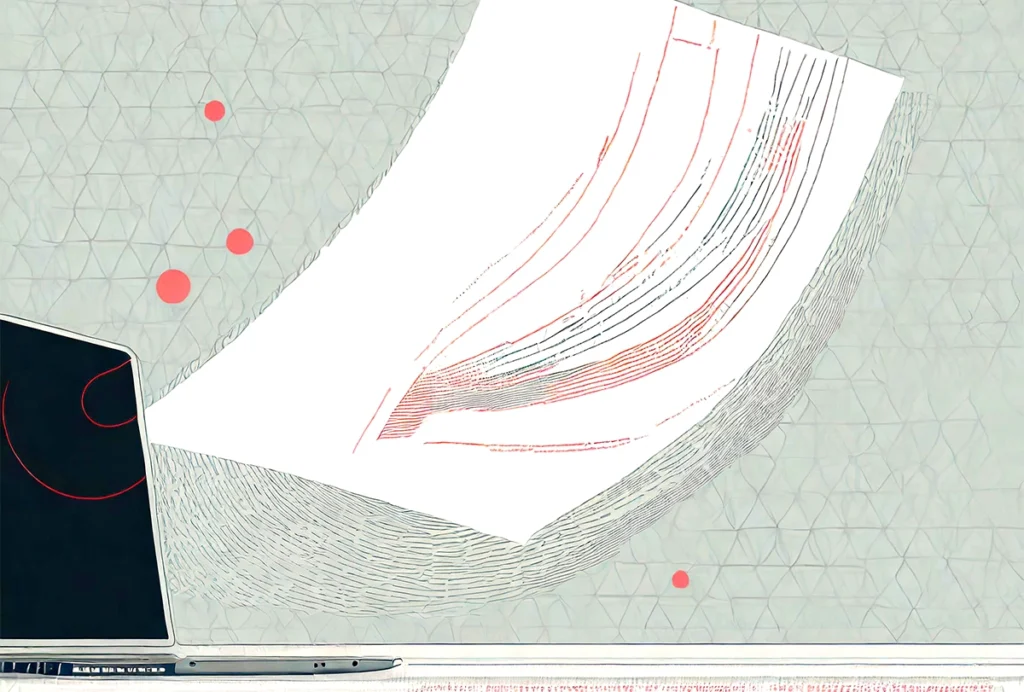
From bench to bot: Boost your writing with AI personas
Explore more from The Transmitter
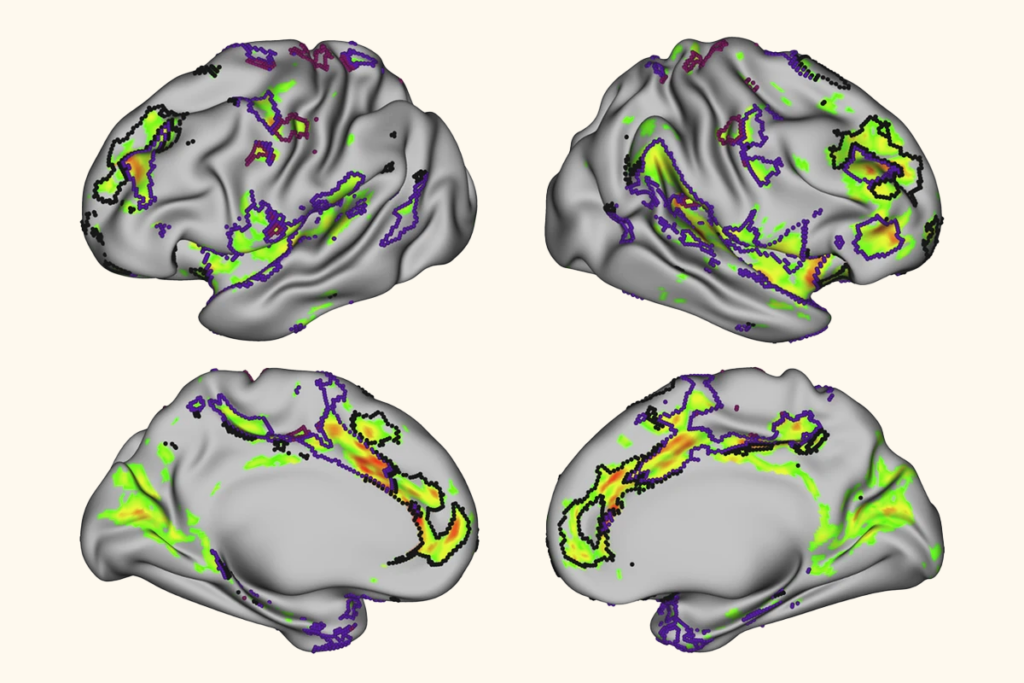
‘Ancient’ brainstem structure evolved beyond basic motor control
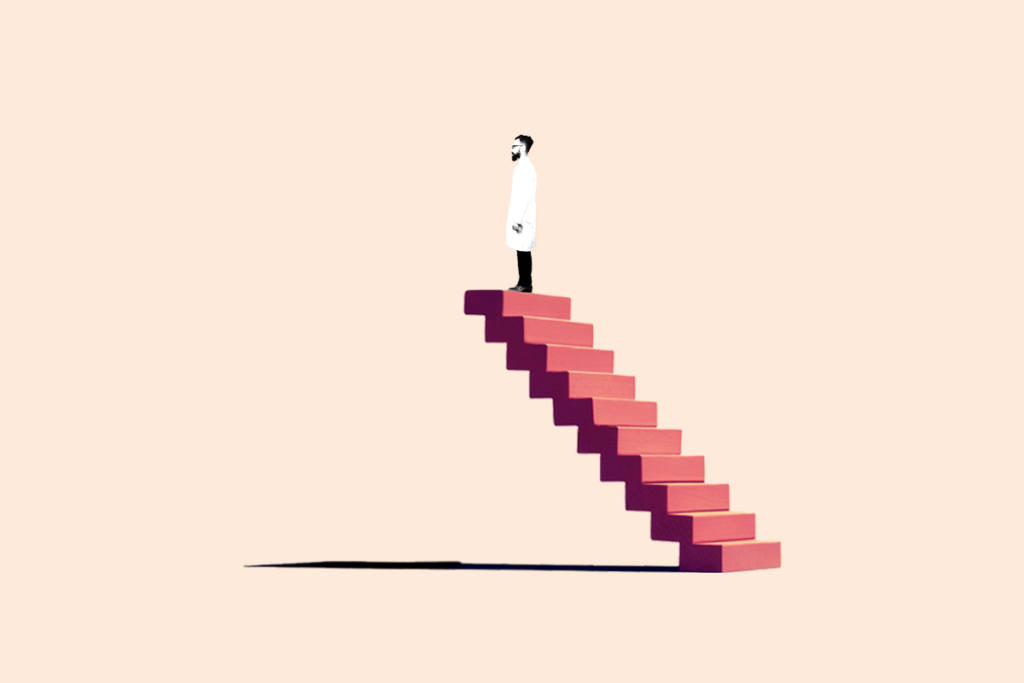