Reconstructing dopamine’s link to reward
The field is grappling with whether to modify the long-standing theory of reward prediction error—or abandon it entirely.
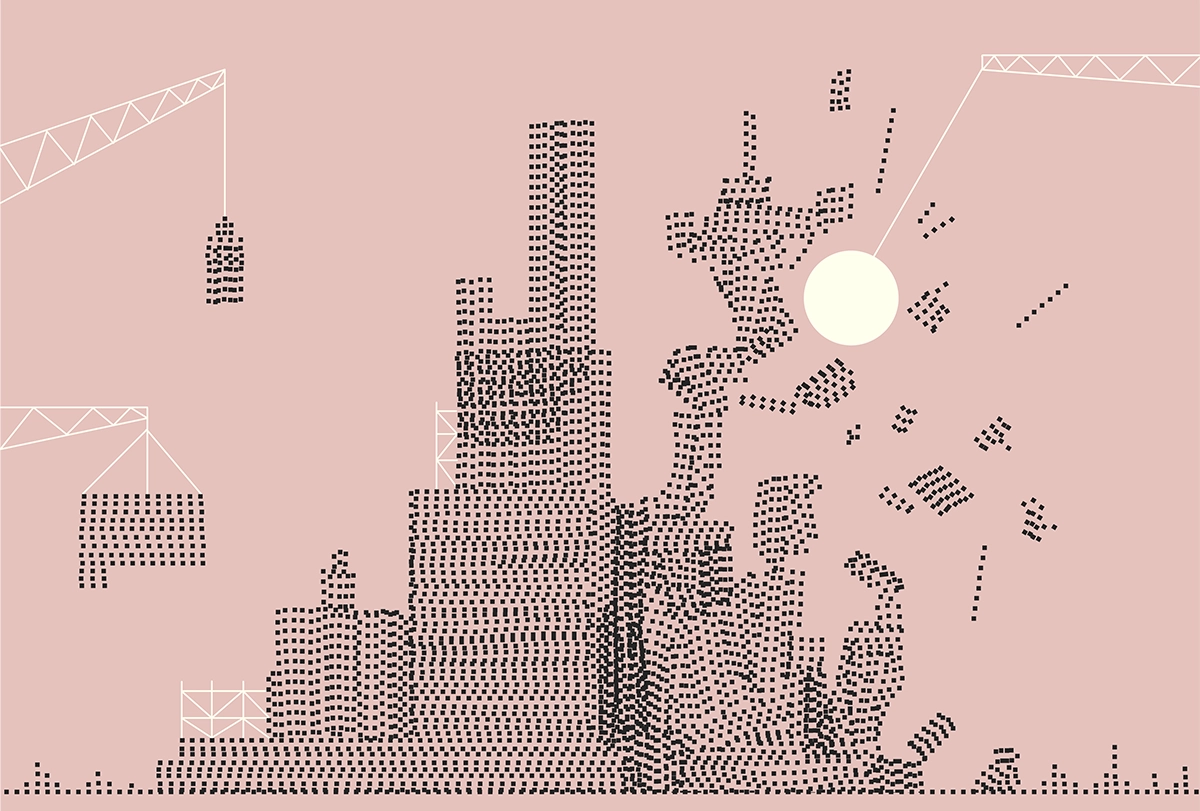
Nathaniel Daw has never touched a mouse. As professor of computational and theoretical neuroscience at Princeton University, he mainly works with other people’s data to construct models of the brain’s decision-making process. So when a collaborator came to him a few years ago with confusing data from mice that had performed a complex decision-making task in the lab, Daw says his best advice was just to fit the findings to the tried-and-true model of reward prediction error (RPE).
That model relies on the idea that dopaminergic activity in the midbrain reflects discrepancies between expected and received rewards. Daw’s collaborator, Ben Engelhard, had measured the activity of dopamine neurons in the ventral tegmental area (VTA) of mice as they were deciding how to navigate a virtual environment. And although the virtual environment was more complex than what a mouse usually experiences in the real world, an RPE-based model should have held, Daw assumed.
“It was obvious to me that there was this very simple story that was going to explain his data,” Daw says.
But it didn’t. The neurons exhibited a wide range of responses, with some activated by visual cues and others by movement or cognitive tasks. The classic RPE model, it turned out, could not explain such heterogeneity. Daw, Engelhard and their colleagues published the findings in 2019.
That was a wake-up call, Daw says, particularly after he watched videos of what the mice actually experienced in the maze. “It’s just so much more complicated, and high dimensional, and richer” than expected, he says. The idea that this richness could be reduced to such a simple model seems ludicrous now, he adds. “I was just so blinded.”
To reconcile the RPE theory with reality, Daw and his colleagues have developed a different model, which they published in July, that puts a new spin on the original theory: Dopamine neurons still encode errors in predictions, but instead of all the dopamine neurons responding to every kind of cue, each cell has its own narrow window on the world that accounts for the heterogeneity the researchers observed.
Theirs is not the first push to reframe the RPE theory in recent years. As more advanced tools have enabled researchers to collect more complex data than before, the field has gained fresh insights into how the dopamine system operates, how diverse the population of dopaminergic cells is, and how dopamine release seems to happen even in the absence of reward. And as a result, the theory of RPE, which was developed out of simpler data, is increasingly being put to the test.
“I find these studies add interesting aspects to the models one can derive from the empirical data, and I do want to encourage the work,” says Wolfram Schultz, professor of neuroscience at the University of Cambridge, who helped to develop the original RPE theory.
Some researchers, such as Daw and the authors of a recent perspective article on RPE refinements, say that the new discoveries just point to adjustments that need to be made to the original model. Others, including Erin Calipari, director of the Vanderbilt Center for Addiction Research at Vanderbilt University, say they are less convinced of the RPE model’s ability to explain behavior—and they have recently levied both direct and indirect challenges to RPE, suggesting that dopamine signals salience, or novelty, or retrospective learning, rather than errors in reward prediction.
“There is nothing about the [original] data that is wrong—it’s that you start to get more information, as tools get better, that changes your interpretation of exactly what would be happening,” Calipari says.
The stakes seem small in some ways: A better model can produce slight advantages here or there in predicting the activity of dopamine neurons. But the downstream effects—what it suggests about the brain, where it points for future research and how it shapes translational work—are large. On top of that, the debate over when to tweak or trash a theory reflects how different researchers approach the science. Those in favor of discarding RPE decry its ability to account for new data; those who want to revise RPE point out the alternative models’ inability to account for past findings.
That debate can bring clarity to the field, says Mark Humphries, chair in computational neuroscience at the University of Nottingham, who is not affiliated with the original theory or the critiques of it.
“We’re in that phase where it felt like the story was finished,” Humphries says. “And it’s not.”
T
he idea that dopamine signals errors in predictions about a reward has shaped neuroscience research for decades—ever since the publication of a seminal 1997 paper, “A neural substrate of prediction and reward,” which recorded the activity of dopamine neurons in the VTA of monkeys as the animals learned to associate a flash of light with an unexpected reward of a bit of apple or juice.When the light turned on for the first time, the dopaminergic cells kept ticking along at their baseline activity level, the study found. But after a monkey received a bit of juice, the cells’ firing increased—and presumably released more dopamine. After training, the cells burst into action as soon as the light went on, rather than in response to the reward itself; the monkey had learned to associate the light with a coming reward. And at that point, if the reward did not eventually follow the light, the dopaminergic neurons’ activity dipped. Independent teams later replicated those results in rodents.
Those findings built on a model of “temporal difference reinforcement learning” (or “temporal difference value learning”), which itself built on the 1972 Rescorla-Wagner model of classical conditioning—new ways of thinking about how people, animals and even computers learn from different cues. Using the temporal difference reinforcement learning model, computer scientists successfully taught computers to play backgammon in 1992. When, five years later, Schultz and his colleagues discovered that the model could also predict the response of dopamine neurons, it all seemed to fit. The model’s success inspired a field of learning and decision-making neuroscience, one that sought to use similar frameworks to understand how the internal representations of reward could drive animals to choose between different alternatives.
But dopamine neurons, it turned out, are more diverse in their response properties than previously thought: Not all dopamine neurons respond to errors in reward prediction, and some seem to respond to other features of a decision-making task entirely, as Daw came to see.
Advances in technologies and experiment design aided those discoveries. In the classic studies, animals highly trained on a task were rewarded if they performed correctly—conditions that produced a robust RPE signal. But experiments involving more complex environments and tasks began to tell a different story. Not only can dopamine neurons respond to visual and movement cues, such studies showed, but they can activate in response to threats, according to recordings from neurons in the tail of the striatum in a study from a different lab. These cells do not respond to reward, the team found; instead, they seem to implement reinforcement learning to help mice avoid threatening stimuli. Other researchers have found that dopamine neurons in this brain region respond to “action prediction errors,” which helps support movements that are not linked to threat or reward.
To reconcile that heterogeneity, some researchers propose that the dopamine system tackles each of these modalities independently. But no one dopamine neuron receives inputs from the entire brain, or information about all of the features of the world, Daw and his colleagues point out in their new paper. The adjustments they made to the RPE model lay out how individual dopamine neurons can be biased toward specific types of stimuli—such as threats or actions—yet still have the potential for a general RPE response: The input to each cell is limited, and so each cell is specialized in what it responds to. The activity of those cells as a population can then produce a full prediction error signal, the team suggests.
“It’s a more realistic way of thinking about the anatomy,” says Ilana Witten, professor of neuroscience at Princeton University, who led the 2019 study and co-authored the new paper with Daw. The team’s feature-specific model also makes sense in terms of how the brain might solve the complicated problem of learning in a multi-modal environment, Witten says. “It can just make the problem less complicated to predict reward based on any one modality” for certain dopamine projections, she says. “It’s a lower-dimensional problem.”
Researchers had already tweaked the RPE model to better fit a series of observations about the dynamics of dopamine activity. Once experiments moved from classical conditioning, as in the original monkey study, to rodents navigating virtual environments, researchers began seeing an unusual ramping up of dopamine signals as the animals approached a reward, as first reported in a 2013 paper. These “ramps” are more consistent with dopamine signaling a reward’s value than they are with it signaling errors in reward prediction, others proposed in the years after.
But later work directly contradicted that idea and found that the classic RPE model could, with appropriate adjustments, account for those ramps. “It’s not a disqualifying feature of the prediction model,” says Samuel Gershman, professor of psychology at Harvard University, who conducted that work.
After accounting for ramps and considering the ways in which prediction errors can generalize, the theory remains a useful way to account for dopamine’s role, Gershman and his colleagues argue in the recent perspective article.
Daw and his colleagues have a similar view when it comes to the traditional RPE model: “I wouldn’t say we’re apologists for it,” Daw says. “But we’re trying to preserve what’s good about it, and sort of extend it.”
T
his series of RPE rewrites has not assuaged a litany of concerns that other teams have raised, including the model’s flexibility.“There’s a danger that [RPE] could literally fit everything. Give me data, I could come up with some input to the model that could fit the data,” says Vijay Namboodiri, assistant professor of neurology at the University of California, San Francisco, who with his colleagues has put forward an alternative model called “adjusted net contingency for causal relations,” or ANCCR.
Also, Namboodiri says, the RPE theory accounts for the passage of time in an unrealistic way. The original model must constantly keep track of the amount of time that has passed following every potential cue that might later be associated with a reward, which may work fine for a computer algorithm—but not for a human or other animal, he says. “I think it’s relatively straightforward to say that’s impossible.”
Instead, in ANCCR, an animal has to look back on past events that might have signaled a reward it experienced. “If you imagine that dopamine is a signal that tells you that the current thing is meaningful—meaningful enough to look back and identify its cause—then dopamine is a molecule, or a system, that actually triggers this retrospective learning.”
In 11 different experiments, such as testing what happens in the event of an unexpected reward, ANCCR better predicted dopamine release than did the classic RPE model in each case. The classic model predicts that dopamine will decrease with repeated exposure to an unexpected sugar reward, for example, whereas ANCCR predicts that it will increase, which is what Namboodiri and his colleagues measured experimentally. They published the findings in 2022.
The original RPE model also fails to fully account for variability in animal learning, other work suggests. For instance, most experiments testing it use animals that are almost fully trained on a task—rather than those that are learning the task from scratch—to produce more robust results, says Joshua Dudman, senior group leader at the Howard Hughes Medical Institute’s Janelia Research Campus. But animals don’t always learn in the way that the model predicts, Dudman and his colleagues reported in 2018 after observing how mice perform as they become trained on a classical conditioning task.
Instead, a different form of reinforcement learning—called “policy learning”—better captures an individual animal’s performance, they proposed in a paper published last year. Under this model, dopamine no longer signals RPE; it instead signals the animal’s rate of learning.
That role for dopamine fits with past findings, says Luke Coddington, research scientist at Janelia, who worked on both papers with Dudman. The RPE model predicts that, after training, dopamine is no longer released in response to a reward, but that is not what experiments—including Coddington and Dudman’s—typically show, he says. “There’s still pretty significant reward responses even when the animal has a really good idea of what’s going on.” Instead, as their model predicts, dopamine is still released in response to rewards, even after an animal has learned to associate the reward with an earlier stimulus; the magnitude of dopamine just decreases at that point.
“As you get closer and closer to a good stable solution or model, you want to turn your learning rate way down and keep it small,” Dudman says. He likens it to driving a boat: “It’s easy to overcorrect. And then you actually make your model worse, because you keep overshooting the solution.”
Other recent studies present data that complicate the simple relationship between dopamine and reward. Dopamine release seems to occur, for example, in response to both positive and negative stimuli, such as foot shocks, rather than just in response to errors in reward, Calipari and her colleagues have found. Novel stimuli evoke the highest levels, suggesting that dopamine tracks novelty rather than errors in reward prediction, they reported in 2022.
Dopamine is also released in the striatum outside of a task, when mice are just sitting still, according to a 2023 study. The amount of dopamine released did increase when the mice received a reward, but the signal was overshadowed by background fluctuations in dopamine levels, says Nicolas Tritsch, assistant professor of psychiatry at McGill University, who led the work. Such a signal-to-noise ratio would make it difficult for postsynaptic neurons to learn from reward based on changes in dopamine alone, Tritsch says. Instead, he adds, dopamine may help reinforce all sorts of signals. “We need to rethink what the brain cares about reinforcing.”
Similarly, striatal dopamine fluctuates in response to a mouse’s spontaneous movements, according to another study from last year. Dopamine therefore is not a response to explicit rewards, or errors in predicting those rewards, but a rewarding signal that guides animals to build cognitive maps of their environment, says Sandeep Robert Datta, professor of neurobiology at Harvard University, who led that work and who co-authored the recent perspective with Gershman.
Understanding what an animal finds most rewarding in a given moment calls for studies in more naturalistic environments, says Jesse Goldberg, professor of neurobiology and behavior at Cornell University. When a songbird is learning a new song, for example, dopamine signals predictions of errors in the bird’s performance, rather than in reward as traditionally defined, Goldberg and his colleagues found in 2016. But that signal changes depending on what the bird is experiencing: When male songbirds are offered water while a potential mate is responding to their song, the male birds no longer have dopaminergic responses to the rewarding stimulus of water or to their own song—instead, the neurons respond to the female’s call, he and his colleagues reported in a paper last year.
B
roadening the idea of what animals find rewarding and what dopamine release indicates does not mean that the RPE theory is wrong—just that it might be incomplete, Datta says. “It seems like dopamine is signaling not just for [reward] prediction errors, but errors in general.”In that vein, most researchers are not ready to throw the RPE baby out with the bathwater, says Joe Paton, director of the Champalimaud Neuroscience Programme, who was not involved in developing the original theory.
The vast literature around RPE and dopamine, built up over time and reconciled with known brain connectivity and synaptic plasticity, should not be discounted, he says. “But at the same time, that doesn’t mean that it’s not in need of refinement.”
Some of the ideas can coexist, Paton says. The policy-learning algorithm proposed by Dudman and his colleagues is better at explaining how dopamine neurons facilitate learning than is the traditional model—at least in some parts of the brain, such as the cerebellum, Paton says. But that doesn’t mean that temporal difference learning is not happening in the VTA and striatum, where rewards are more important, he says.
In other cases, the parameters of the model, if properly adapted, can still account for the experimental data. For example, Paton and his colleagues ran the same tests from Namboodiri’s experiment and found that most of the data can be explained by RPE models, Paton says. “They may be demonstrating falsification of a model instance, but [they] fall far short of demonstrating falsification of the entire model class.” (That work has not yet been published.)
Namboodiri says that is a fair point, but that it may be a problem that no single instance of the model can capture all the known results of temporal difference RPE. “It can’t be that for one paper, animals use one algorithm, and for another paper, the animals use a different algorithm,” he says. “There needs to be some reckoning of the fact that these are actually different algorithms, even though they happen to use RPE as a computation in them.”
Some of those differences of opinion may stem from personal philosophy, many of the researchers in the field agree. Some people like to work within the historical framework, adding their own information like new bricks to a sturdy wall. Others are less inclined to trust the wall; they prefer to build their own theories and test them against existing structures. But even determining how to go about those tests can vary from scientist to scientist.
For example, researchers disagree about how precisely a model needs to match the data, Dudman says. He contends that, although Witten and Daw’s new model does successfully solve the task it is designed to solve, it only fits the data qualitatively, rather than quantitatively. “I think this is part of how science goes. There are different opinions about what constitutes an explanation and how you would rule out, or rule in, different explanations.”
Figuring out which approach is correct is not impossible, but it will be challenging, given the diversity of models that are currently being tested and the diversity of opinions on how to test them, Dudman adds. “How do we grapple with everybody’s data in all these different ways?” He says that he tries to be optimistic about the future of the field, but it can be difficult, because science often runs on a positive-reinforcement loop. “You give grants to people whose theory looks nice and plays nice with yours,” he says. “I have some concerns about that.”
Ultimately, the proof will be in the data, Gershman says. “I would completely drop [the RPE theory] on a dime if people showed me compelling enough evidence. I just disagree that the evidence is sufficiently compelling,” he says.
And the fact that the dopamine field can even have this debate is a good sign, Gershman adds. “We all agree that there’s some class of models that we’re trying to discriminate between, and we’re designing experiments to do that,” he says. “I think the real problems are for the areas of systems neuroscience where people aren’t doing that.”
Recommended reading
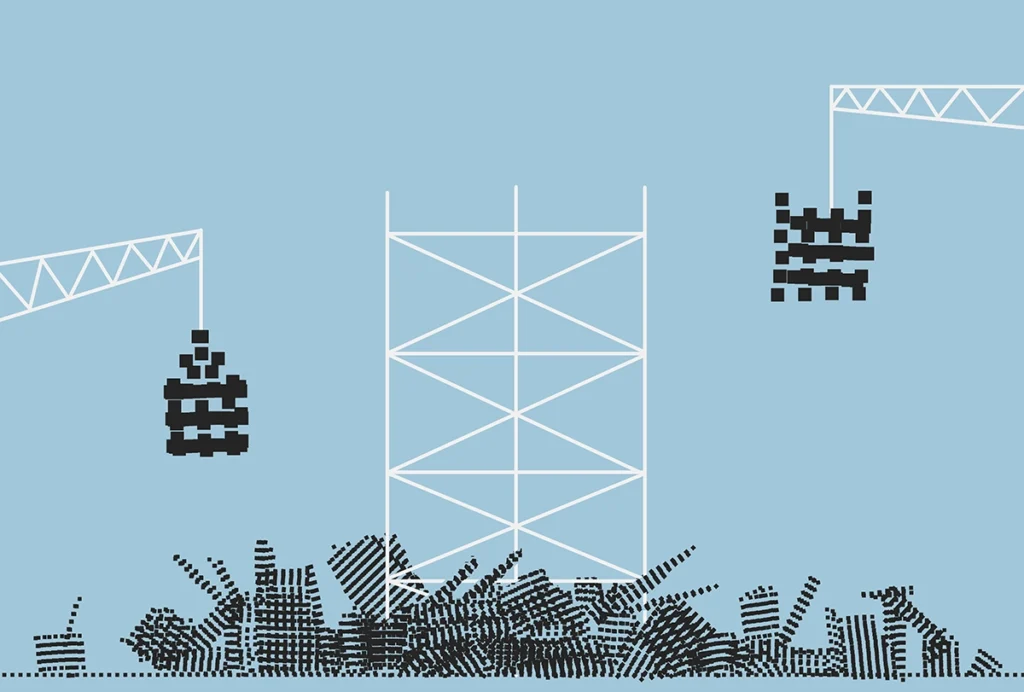
Dopamine and the need for alternative theories
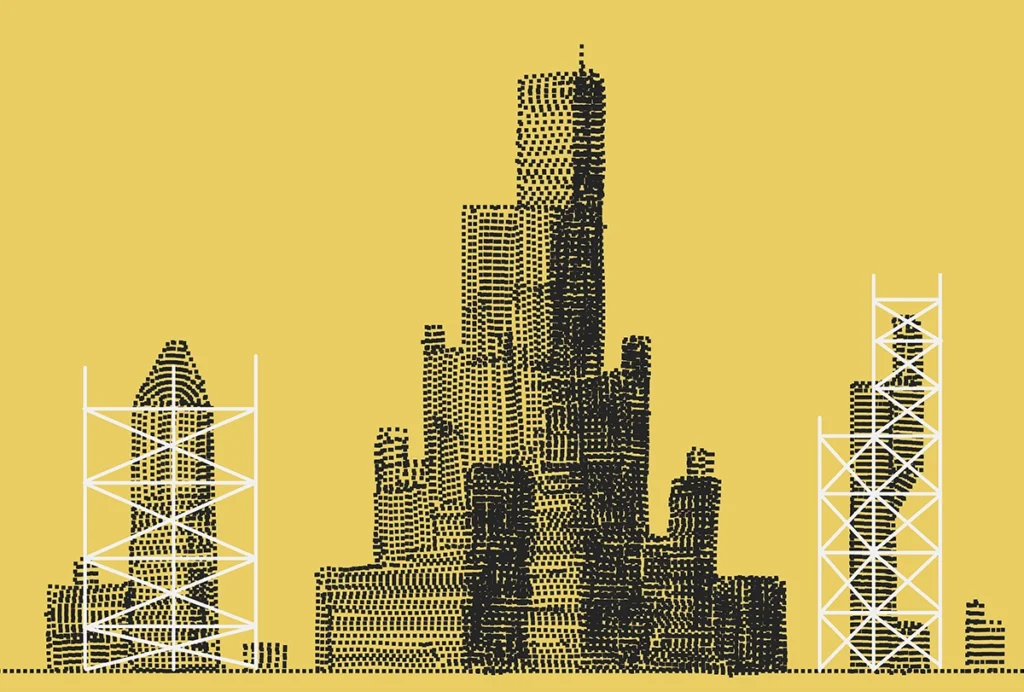
Does a new theory of dopamine replace the classic model?
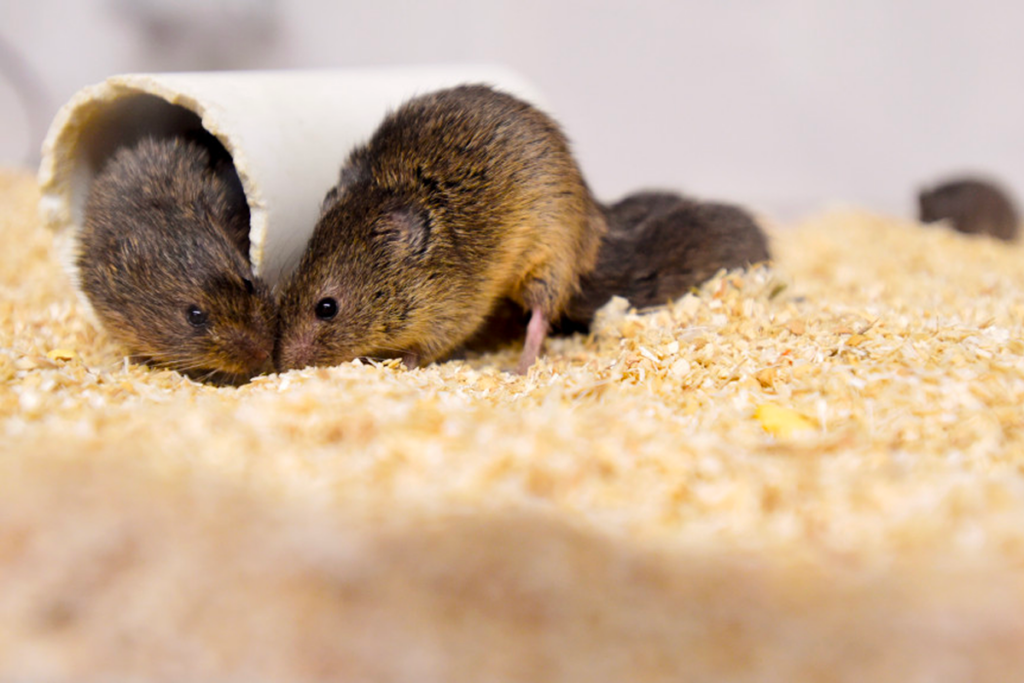
Brain gene expression syncs between bonded prairie voles
Explore more from The Transmitter
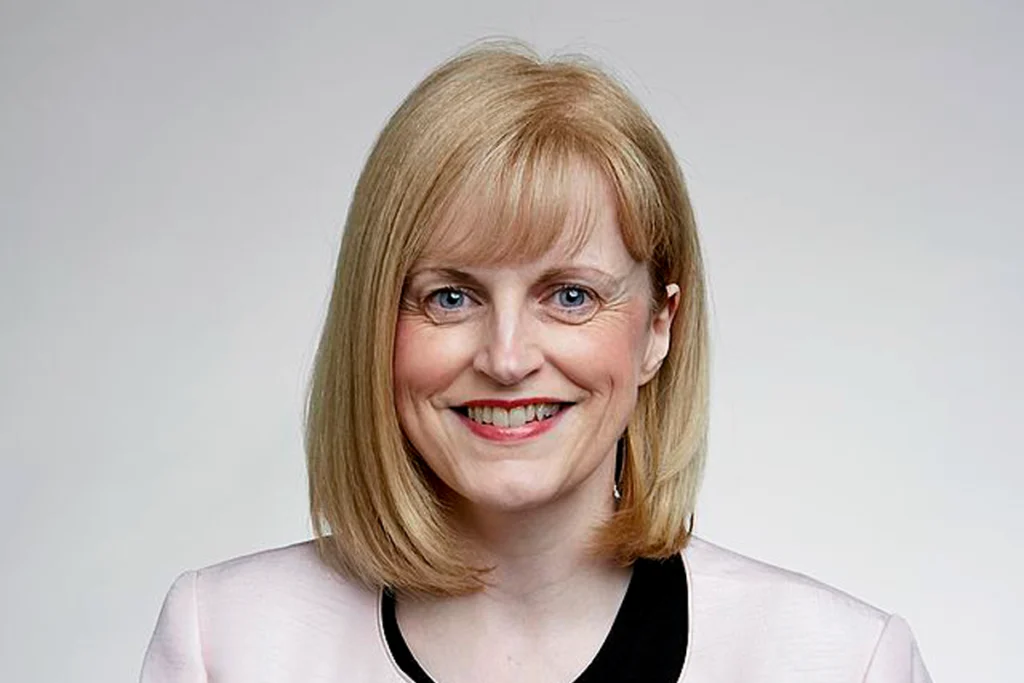
Remembering Eleanor Maguire, ‘trailblazer’ of human memory
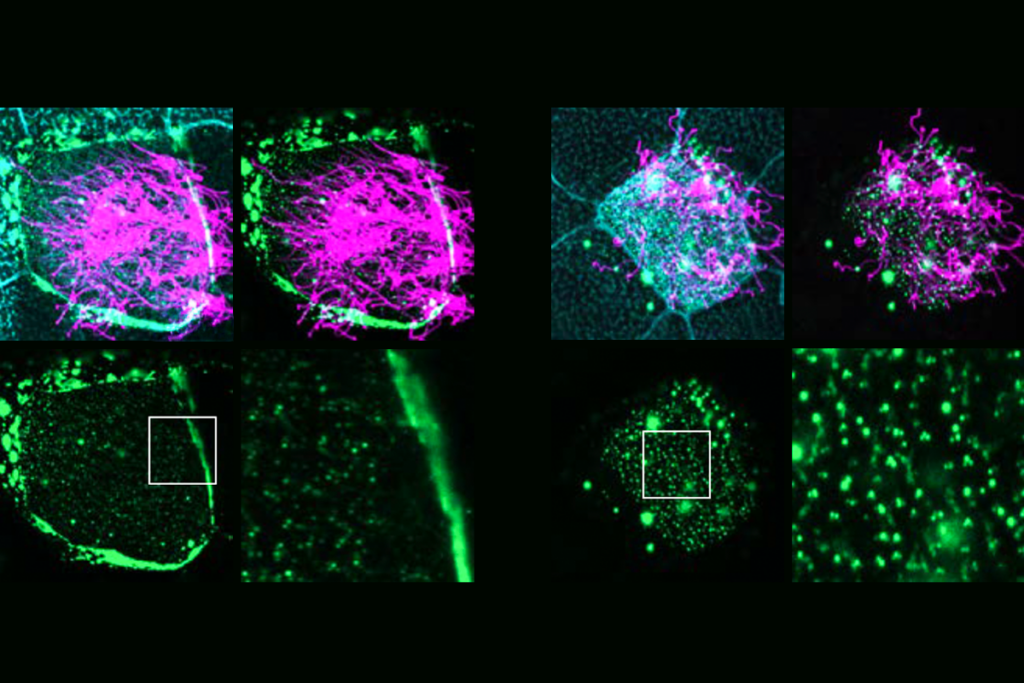
Many autism-linked proteins influence hair-like cilia on human brain cells
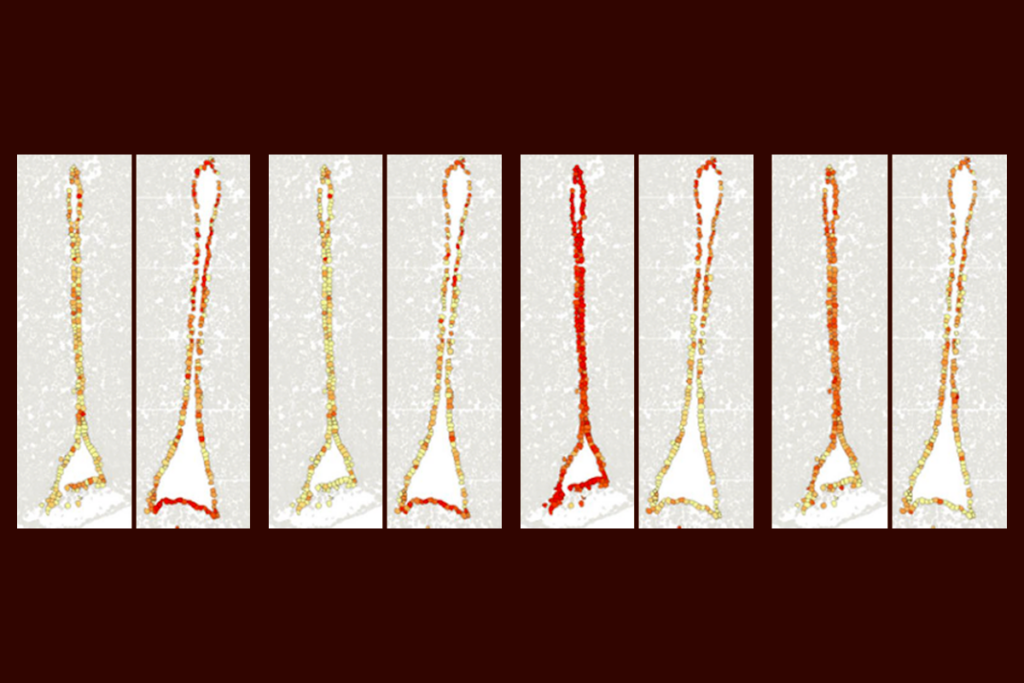