Where do cell states end and cell types begin?
High-throughput transcriptomics offers powerful new methods for defining different types of brain cells. But we need to think more explicitly about how we use these data to distinguish a cell’s permanent identity from its transient states.
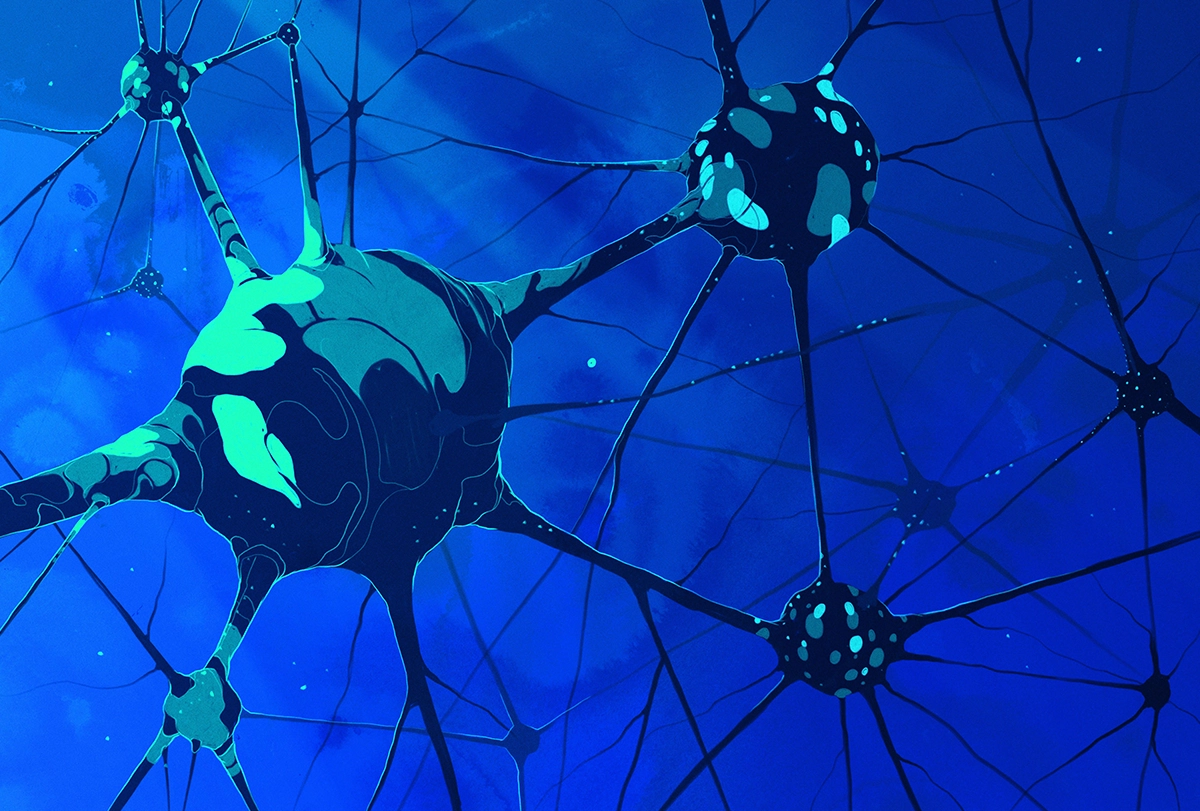
”‘Frogs are frogs and fish is fish, and that’s that!’ said the tadpole.
Lionni L. (1970). Fish is Fish. Pantheon Books.
In the children’s story “Fish is Fish,” a minnow and a tadpole celebrate their identity as fellow fish until the tadpole grows legs and hops out of the pond as a frog. The story reminds us that a collection of features observed at any one point in time is only a snapshot along the trajectory of any living thing, be it a cell or a more complex organism. Even if we introduced a subclassification of fish that includes both minnow and tadpole, without additional data these categories alone would not foretell that these creatures ultimately head in radically different directions from each other.
Neuroscientists risk falling into the same trap when it comes to cataloging the diversity of cell types that make up the brain. Single-cell and single-nucleus RNA sequencing (scRNA-seq and snRNA-seq) have revolutionized our ability to resolve the brain’s heterogeneity—unsupervised algorithms can quickly classify cell types based only on the expression patterns of thousands of genes.
Despite its success, though, the reliance on transcriptomics to define cell types comes with intellectual hazards. Like the fish story, defining cell types from transcriptomic snapshots assumes that a cell’s gene expression is relatively fixed in time. Yet decades of evidence show that neurons undergo widespread and robust changes in their transcriptional programs in response to stimuli, including experience-driven neural activity. And these experience-induced transcriptional states can be quite persistent in contexts in which they mediate behavioral adaptability.
Given that gene-expression programs play a central role in defining both neuronal classification and cellular plasticity, how should we consider the question of where cell state ends and cell type begins?
Time-dependent transcriptional states are well understood in developmental biology. All the cells in an organism ultimately derive from the same source—a single fertilized egg—and all contain the same genomic DNA. Over the course of cell divisions and environmental exposures, progressive changes to the epigenome promote or restrict the expression of different genes, driving transcriptomic identities of distinct cell types.
Once established, epigenomic states can be remarkably persistent, such as the chromatin landscape that keeps one X chromosome permanently inactivated in each female cell. High-throughput, single-cell transcriptomic technologies rely on the stability of genome regulation to classify cells, and indeed, transcriptomic classifications of neurons overlap robustly with chromatin accessibility and DNA methylation patterns in single cells, supporting the premise of this classification strategy.
But the narrative of epigenomic inflexibility is inconsistent with current neuroplasticity research, which over the past 20 years has documented that numerous features of the epigenome can be modified by experience, even in terminally differentiated, post-mitotic neurons. If such fundamental mechanisms of genome regulation can change in a fate-committed cell, then researchers are left with an important question: Do differences in gene-expression programs always represent fixed cell types? Or could they also reflect transient cell states?
H
ow we think about this question is shaped by both culture and technology. The microglia field offers an example of how naming conventions can influence how we interpret biological data. Historically, some groups referred to microglia in a way that implied static functional identities, akin to cell type, and others used language more reminiscent of cell state. A recent consensus paper on microglial nomenclature argues that the more static naming approach obscured an important aspect of microglial biology—that microglia transcriptomes are highly sensitive to the local environment. (Ironically, when describing neurons, the authors made the same mistake they had warned against, referring to neuronal transcriptomes as “fixed and terminally differentiated,” ignoring their potential for plasticity.)The techniques researchers use to analyze transcriptomics data, notably cell-clustering algorithms, also shape how we think about cell identity. These algorithms were explicitly designed to find discrete gene-expression programs that differ between tissues and represent cell types. They tend to overlook more subtle gene-expression programs in scRNA-seq data, those that vary over time or within areas and may reflect cell state. But clustering algorithms can sometimes detect cell state from scRNA-seq data as well—unpublished research suggests that they can identify neurons in a seizure state. Though this case represents a well-defined type of cell state, in which a single class of neurons is undergoing a precisely timed program of gene expression, it shows that the features we use to distinguish cell type from state may be less distinct than we think.
New computational approaches further support this idea. For example, Dylan Kotliar and his colleagues developed a mathematical model using matrix factorization that assumes cells can simultaneously express more than one gene transcription program, permitting cells to be assigned to more than one cluster. The researchers applied the model to snRNA-seq data from the visual cortex of mice that had been dark-adapted or exposed to light and showed they could identify activity-regulated transcriptional programs embedded both within and across cell-type identity clusters.
Studies that take a cell’s precise location into account also support a more complex picture, identifying gene-expression programs that vary continuously across brain structures rather than in a discrete fashion. It remains to be resolved whether these gradient gene-expression programs should be conceptualized as subtypes of a cell type versus a single cell type that is varying its gene-expression state in response to its local environment. This question will become especially important as the field begins analyzing a major tranche of the data from the National Institutes of Health’s BRAIN Initiative Cell Census Network (BICCN), published last October. These data will need to be placed into the context of brain circuits using spatial transcriptomics.
New experimental and computational methods will undoubtedly be essential in refining our understanding of cell types. But like the minnow and tadpole in the fish story, it will also be helpful to think outside the pond.
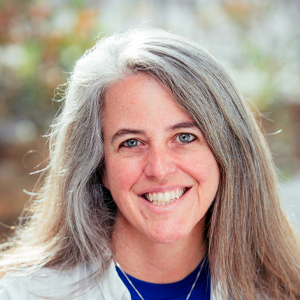
Anne E. West
Duke University School of Medicine
Recommended reading
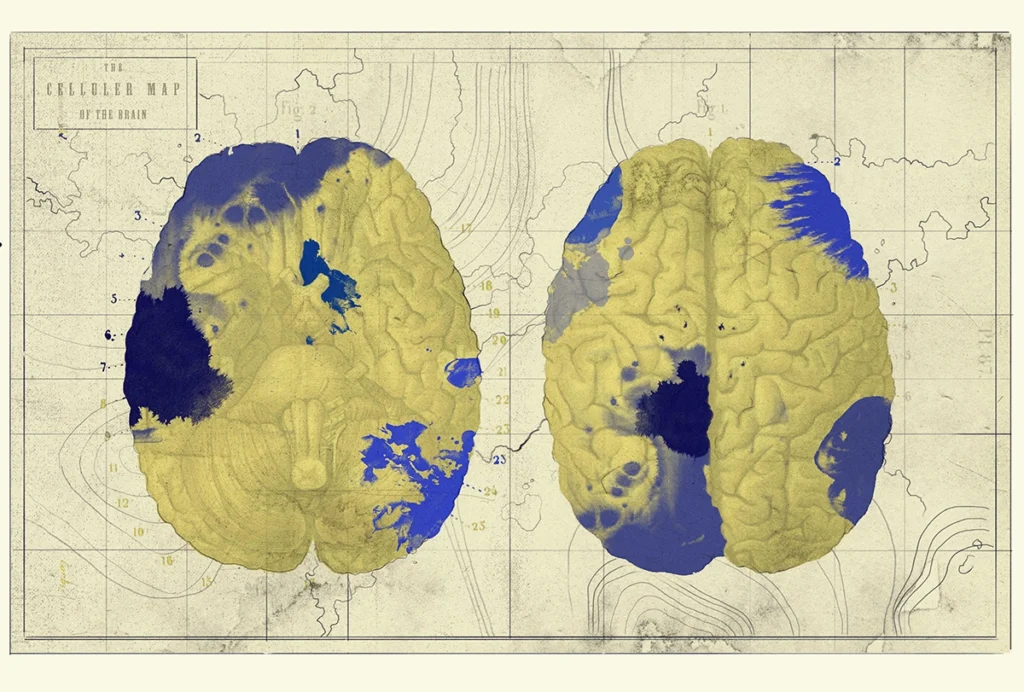
Knowledge graphs can help make sense of the flood of cell-type data
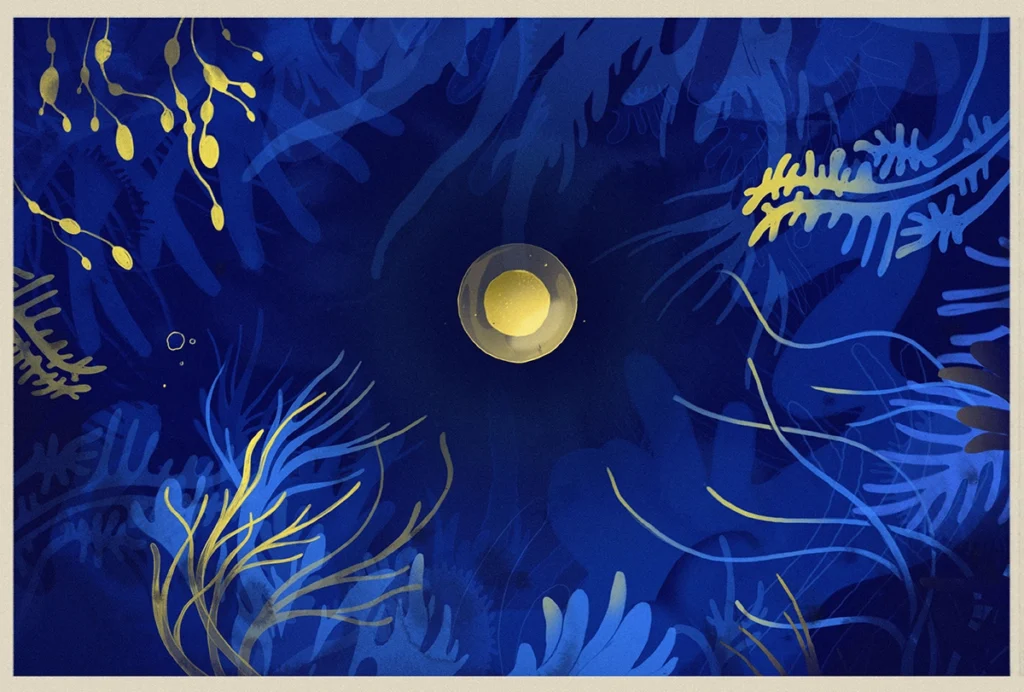
Building a brain: How does it generate its exquisite diversity of cells?
Explore more from The Transmitter
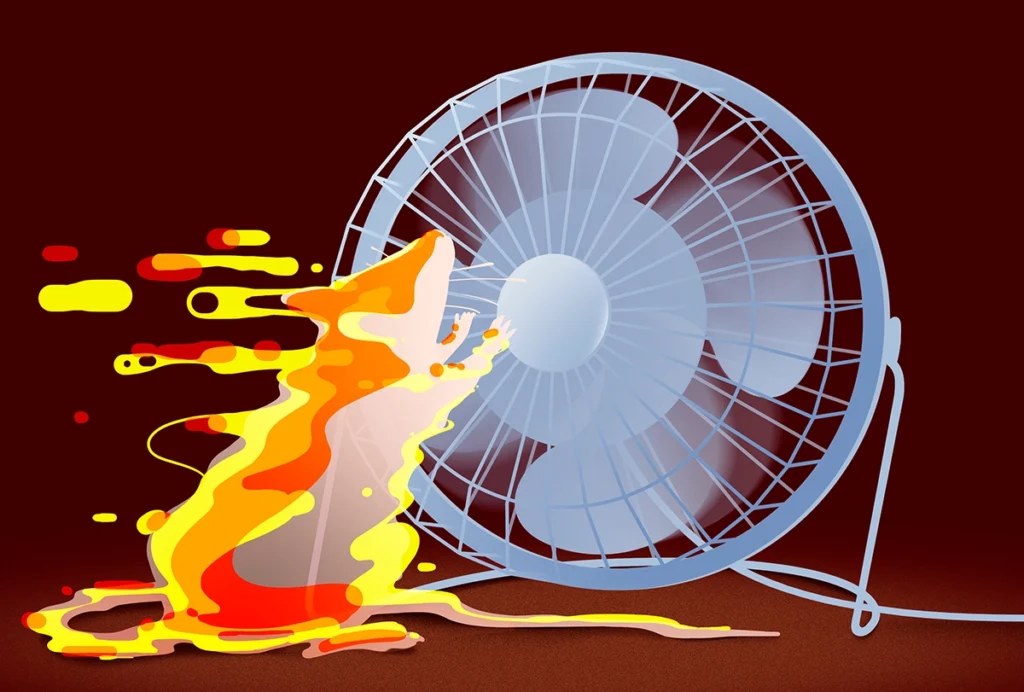