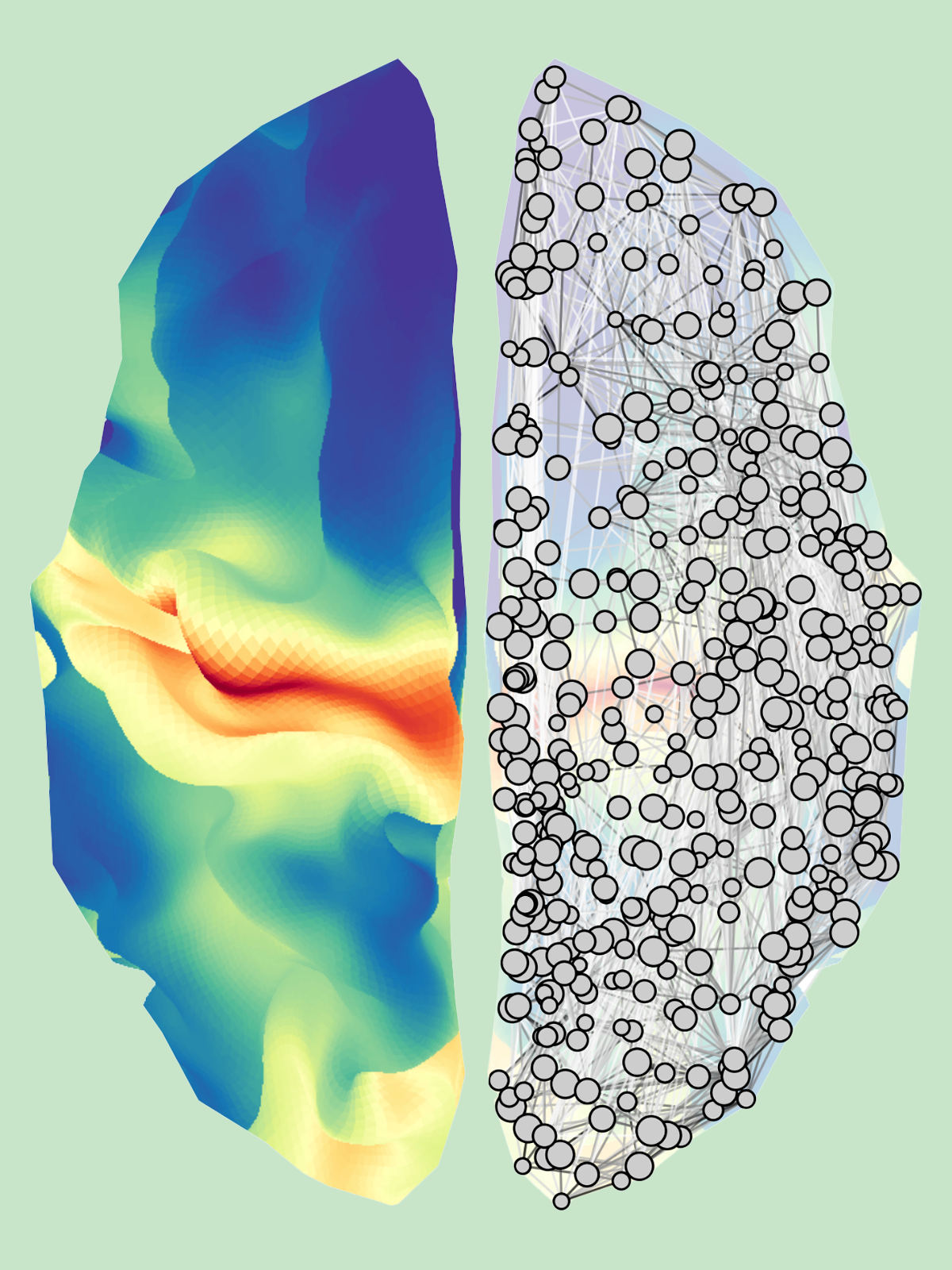
How can we fold cellular-level details into whole-brain neuroimaging networks?
I got answers from Bratislav Misic, who is inventing practical ways to connect the brain’s microscopic features with its macroscopic organization.
Bratislav Misic, who leads the Network Neuroscience Lab at the Montreal Neurological Institute-Hospital (MNI), has a particular knack for finding insightful ways to meld different imaging modalities into parsimonious descriptions of the network structure of the brain. Misic has applied that talent to fuse multi-modal neuroimaging datasets, map structure-function relationships and create beautiful, intuitive visualizations.
In one collaborative project, for example, Misic combined forces with Alain Dagher, a neurologist at the MNI, to better understand the mechanisms underlying neurodegenerative disease. Together, they devised a way to model how misfolded alpha-synuclein protein —implicated in Parkinson’s disease — moves through the brain’s white-matter network. Their study opened new ways of thinking about how these pathological proteins propagate. The findings also illustrate how we can combine cellular-level information with network-level descriptions of the brain.
In a review published in Nature Reviews Neuroscience in October, Misic, along with graduate students Vincent Bazinet and Justine Hansen, outlined the different approaches neuroscientists can take to map hard-won details from multi-modal neuroscience studies, such as the expression of particular classes of neuromodulatory receptors, into complex, network-level maps of the whole brain. I was excited to speak with Misic and learn more about how he so effortlessly crosses disciplinary boundaries.
This interview has been edited for length and clarity.
Mac Shine: Tell us a little bit about who you are, where you work and what you do in your day-to-day life.
Bratislav Misic: I’m a mathematician by training. Towards the end of my Ph.D. in Toronto, I attended a talk by Randy McIntosh. Up to that point, everything we had been taught in my undergrad courses was about how a particular brain area does this or that, or how a cognitive process can be broken down into smaller bits. And then Randy came along and gave this amazing talk about how, to understand brain function, you need to understand how areas coordinate with each other, how they talk to each other and participate as part of a bigger network. I thought: That’s amazing — that’s what I want to work on — and so I started a Ph.D. with Randy. A few years later, I was fortunate enough to get a position at the Montreal Neurological Institute.
MS: During your training, did you ever get to work with actual brains, or did you just learn from textbooks?
BM: Yeah, I was taught real functional neuroanatomy by someone in the medical school, and they had dissected brains and everything. It was actually an excellent course, with great functional neuroanatomy: It was set up so that we were asked about a patient who might present with a set of symptoms, and we had to figure out where in the brain the lesion might have been, or what the functional consequences would be. It was a really wonderful course that just cemented my interest in the brain.
MS: When I was in medical residency, we used to joke that clinical neurology is a bit like a game of “pin the tail on the donkey,” with lesion sites instead of tails. But there’s a limit to this kind of “locationalist” thinking, exposed in recent years by the “network” perspective. Can you tell us what network means to you?
BM: The idea, at its core, is that we’re studying a system in which there are many interacting elements. We need simplified ways of reducing this extraordinary dimensionality that allow us to summarize the system with a set of simpler principles. In this case, we’re using the tenets of network science, which is a branch of mathematics that summarizes the activity of the brain as a group of nodes that are connected by a set of edges.
MS: So you can make a network of “nodes” and “edges” out of any kind of data you like: This might be a set of pre-defined regions in the brain and the white-matter tracts connecting them, or something more abstract, such as the correlation between activity patterns.
BM: That’s right. How you construct a network is up to you. For me, the best way to think of nodes is as “neural elements,” in which the resolution of the elements can change as a function of your level of description. For folks who are looking at cellular networks, it could be populations of neurons or columns; for those conducting whole-brain imaging, the nodes might become collections of neurons that presumably represent, potentially, millions of cells. The edges are similarly flexible — they can be physical connections, such as axons, or the statistical correspondence between patterns of activity over time. The choice is really up to the experimenter.
MS: And just as there are many ways to construct a network, there are similarly many ways to analyze them.
BM: Yeah, there are a few popular ways. The first I call “counting” analyses — these let you find the most influential node in a network, such as identifying the most popular person in a neighborhood. For these approaches, you simply count the number of edges (or friends) that the node has: The more edges, the greater the influence. We call nodes with lots of edges “hubs.” Another complementary approach is called “path” analysis: These involve tracking how information (or influence) travels in a network. In this case, you ask how easy it is to get from one node in a network to another — the most influential nodes are those that often appear in the shortest paths. There are, of course, many other more sophisticated techniques, such as structural equation modeling and computational modeling approaches, but many of them use the same basic intuition.
MS: One of the things I love about your work is your effort to combine the information we obtain from different imaging modalities together, using tools from network science. In your recent review, you took this a step further than we’ve gone before by demonstrating ways that we can incorporate hard-won, cellular-level biological details into networks that are often missing these features. Can you tell me a bit about that?
BM: We wanted to take advantage of the amazing neuroimaging data in the community to make richer, more detailed maps of networks. By way of analogy, think about someone influential in your own social network: Are they popular? Are they rich? Do they travel a lot? Unsurprisingly, if you build more biologically realistic information into network representations of the brain, you can do a better job of recreating a variety of different neuroimaging signatures, or provide more effective descriptions of how the brain fails in models of disease.
MS: Kind of like the lovely work you’ve done with Alain Dagher and the spread of alpha-synuclein around the brain in people with Parkinson’s disease?
BM: I was unimaginably fortunate that, when I started at the MNI, my office was right next to Alain Dagher’s — he’s been a great collaborator and a mentor to me ever since I started. Here at the MNI, the whole center is focused on creating interdisciplinary conversations, and my discussions with Alain have been really transformative. One of the things that Alain mentioned when we first started working together is that many different neurodegenerative syndromes — Parkinson’s disease, Alzheimer’s disease, ALS and others — have a common pathological process, in that abnormally folded proteins accumulate in neurons, causing the cells to die. But the proteins then spread from cell to cell through synaptic connections.
MS: Like a meme spreading around a social network?
BM: Exactly. We simulated a misfolded protein in a particular area and then predicted the spread of the protein using networks derived from diffusion MRI. I think this is a really fascinating idea, and although the results are still somewhat preliminary, there are rumblings in the literature that maybe the idea of pathological spread around the brain’s network architecture is a good model, not only for the neurodegenerative diseases for which we very well know the proteins involved, but also for a variety of other neurological and psychiatric diseases.
MS: It strikes me that you have productive conversations with mathematicians, neuroimagers and neurologists — how do you facilitate these kinds of conversations?
BM: Richard Hamming had this great story from when he worked at Bell Labs. He recommended making it a point to sit at different tables every lunchtime so that you can try to meet everyone and talk to them about what they do. We have so much to learn from other fields, and just starting conversations with people from different backgrounds has a way of opening up opportunities for interdisciplinary collaboration.
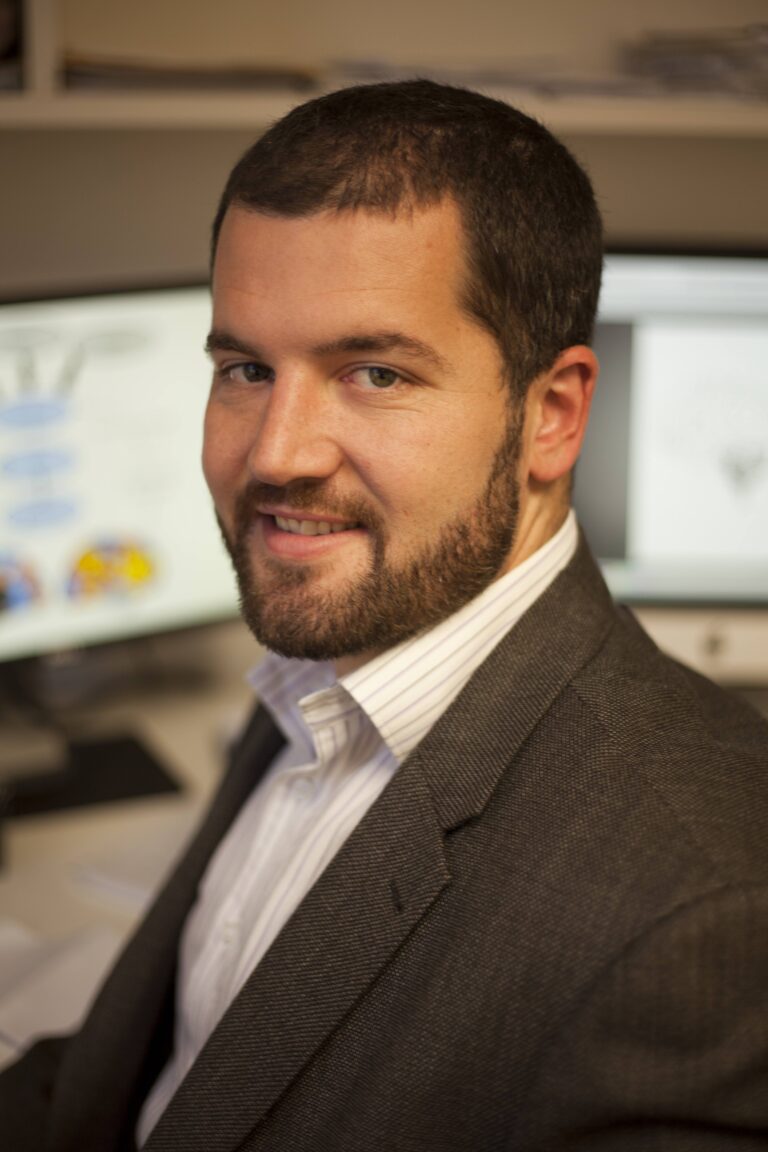
Mac Shine
University of Sydney
Recommended reading
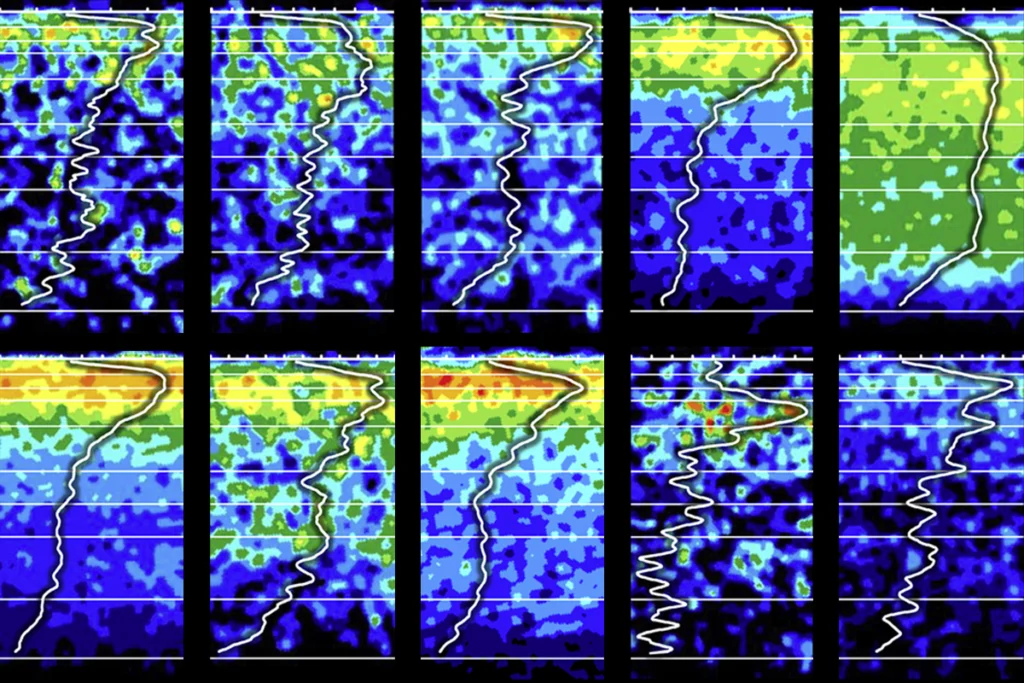
What happens when a histopathologist teams up with computational modelers?
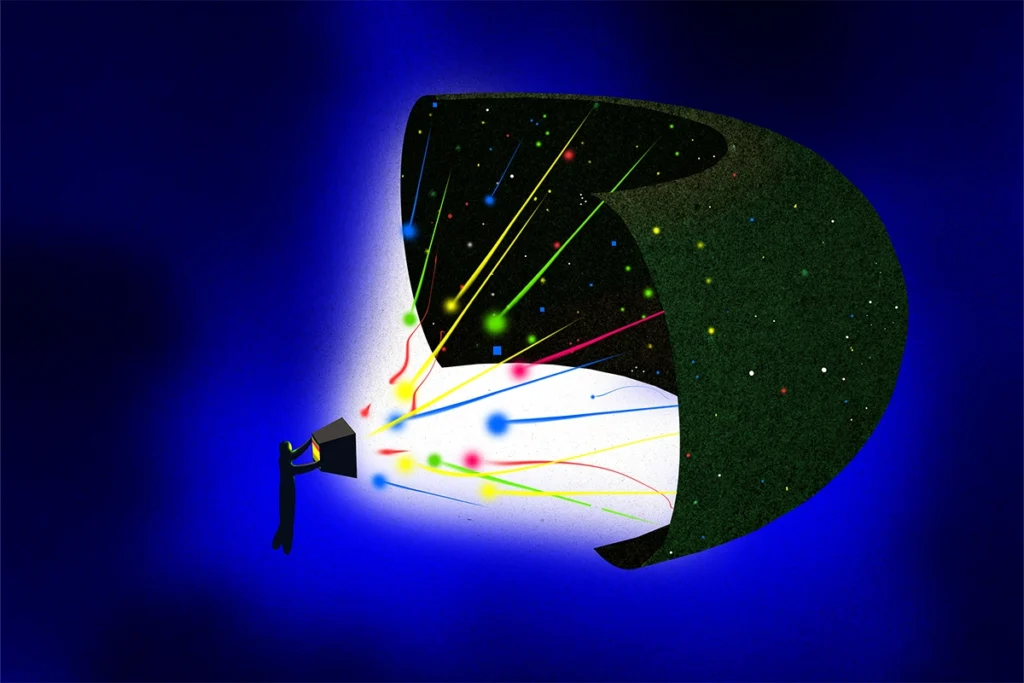
Imagining the ultimate systems neuroscience paper
Explore more from The Transmitter
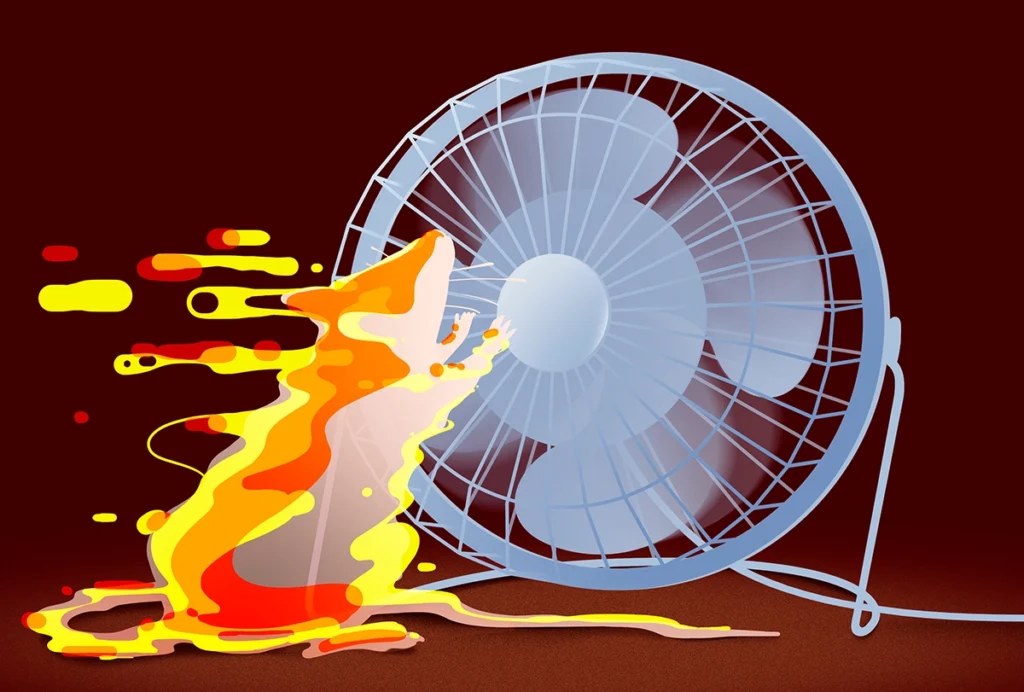