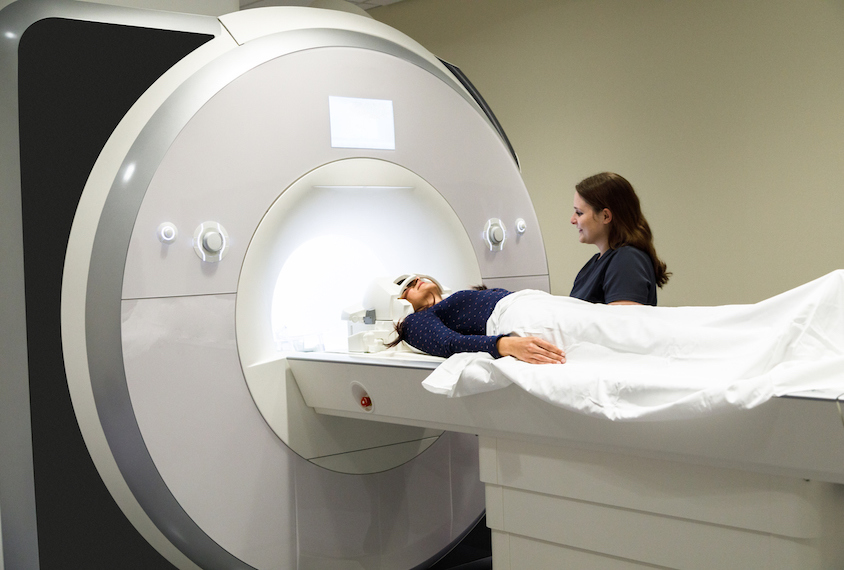
Head motion mars most fMRI results, even after correction
A new measure shows how greatly movement influences associations between traits and brain activity, revealing abundant false positives and false negatives.
A new metric called a motion impact score could help identify whether study participants’ movements during functional MRI (fMRI) scans bias the correlations researchers glean from those data, according to a preprint posted to bioRxiv in December. Using the statistical approach, its creators found that 39 out of 45 traits they evaluated, including measures of cognitive function as well as physical traits such as body mass index (BMI), significantly correlated with head motion, even though the data had been corrected to remove such artifacts in advance.
This so-called leftover motion could confound the results of many brain connectivity studies, the researchers say. Calculating a motion impact score, however, could help scientists plan studies that are better at sussing out real correlations while avoiding spurious ones.
False negatives — in which head motion serves to weaken an apparent correlation — could be a particular problem for autism studies that use effect sizes estimated from non-autistic people, who tend to move less in the scanner than autistic people do, says study investigator Benjamin Kay, assistant professor of neurology at Washington University School of Medicine. “Those studies might be underpowered, because that extra residual motion artifact is obscuring what you really want to see.”
The new result is “another illustration that simply applying [existing] tools, while they do help, is insufficient to fully address, to the degree that it can be fully addressed, head motion,” says Eric Porges, assistant professor in the clinical and health psychology department at the University of Florida in Gainesville, who was not involved in the study but has similarly found that head motion may affect fMRI results in older adults.
And although the approach still needs to undergo peer review, Porges emphasizes, it could be “implemented into our standard functional connectivity pipelines.”
N
“Unfortunately, head motion is correlated with all sorts of variables of interest — almost all of them, including all neurological and psychiatric conditions,” says Nico Dosenbach, co-lead investigator of the new study and associate professor of neurology at Washington University School of Medicine in St. Louis. Dosenbach co-founded Turing Medical (formerly NOUS Imaging, Inc.) to develop and sell software aimed at evaluating the quality of fMRI data in real time. “If something’s a little different about your brain, it’s also harder to hold still.”
Numerous methods exist to rid the data of motion-induced artifacts, including realigning the frames disturbed by movement, filtering out certain frequencies that tend to be associated with motion, and removing the frames most affected by movements. But as Kay discovered while mining data from the Adolescent Brain Cognitive Development (ABCD) study, even corrected data are riddled with head-motion artifacts.
As Kay created maps of brain connectivity for participants with attention-deficit/hyperactivity disorder (ADHD), he noticed that they “actually look a lot like the brain maps for if you just map out motion,” he says. “I became very paranoid about it — like, oh my God, I’m probably just looking at motion artifacts.”
The trouble was, there was no good way to determine just whether the correlation he found between ADHD and the participant’s brain connectivity was spurious. Existing methods evaluated overall motion effects, not whether motion specifically influences a correlation with a particular trait. So Kay decided to invent a statistical approach to do just that.
His solution takes each participant’s fMRI data and splits it in two — a low-motion half and a high-motion half. The idea, he explains, is that although motion varies from second to second, any variable of interest is relatively constant within an individual: “It’s the same person, so their brain should be doing about the same thing.” Any differences in the correlations identified in each half would then indicate that the leftover motion was influencing the overall result, with greater differences pointing to larger artifacts.
“Their way of testing was kind of clever,” says Andre van der Kouwe, a biomedical engineer at Massachusetts General Hospital in Boston, who did not work on the preprint but who has collaborated with one of its co-lead investigators, is involved in the ABCD study and holds stock options in Turing Medical. “I hadn’t heard of anyone doing that before.”
Sure enough, among the 7,270 children in the ABCD study who had all the data needed to calculate the motion impact score, Kay’s approach revealed that head motion affected a majority of the correlations. Many correlations, such as the relationship between brain activity and BMI, were stronger due to motion artifacts, whereas others, such as the brain activity associated with matrix reasoning scores, were weaker.
These so-called false negatives were “the biggest surprise,” Kay says, because in these cases, “the pattern of the motion tends to hide the real finding.”
T
To do that, Kay and Dosenbach recommend that research teams aim to remove frames that are most affected by motion, although that requires large datasets.
They also suggest taking a more flexible approach to designing studies, to account for when motion artifacts may be more problematic. For example, researchers could recruit more participants who tend to move more in MRI scanners, or allow those participants to spend more time in the scanner than those who move less. This is also where the real-time feedback on the quality of the data — the approach championed by Turing Medical — can help, they say. “So instead of saying, ‘Everyone gets scanned for 20 minutes,’ it’s, ‘Everyone gets scanned until we have 8 minutes of useable data,’” Dosenbach explains.
Van der Kouwe also advocates for real-time data monitoring — specifically, real-time data correction — but says that it’s still “really early days” for such technology. “Ideally we’d fix the problem before we’d even acquired the data, but [for] now we’re stuck with fixing it after the fact.”
Kay and Dosenbach note that using motion impact scores can help identify traits that are perhaps best studied by other means. Their results showed that physiological variables such as a person’s BMI are the most integrally linked with head motion, so much so that it was hard to disentangle the two.
On the other hand, “the cognitive variables that a lot of folks are interested in … they’re not hopelessly confounded,” he adds. “That’s probably the most positive news here, is that aggressively removing motion effects, which is definitely what we recommend, can also reveal findings that would otherwise have been hidden.”
Recommended reading
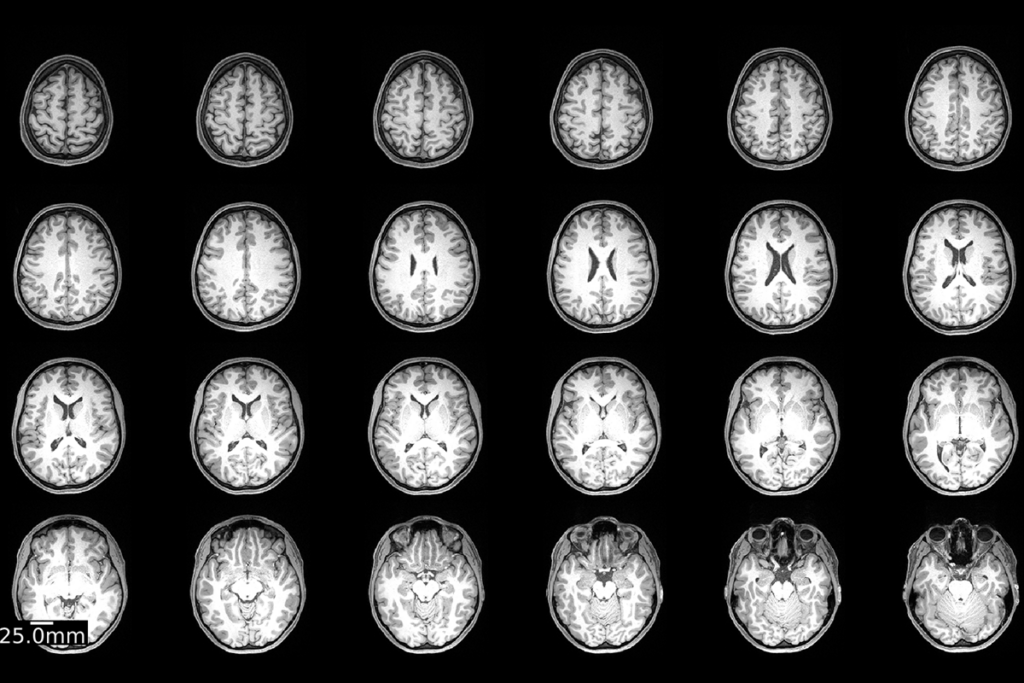
Dose, scan, repeat: Tracking the neurological effects of oral contraceptives
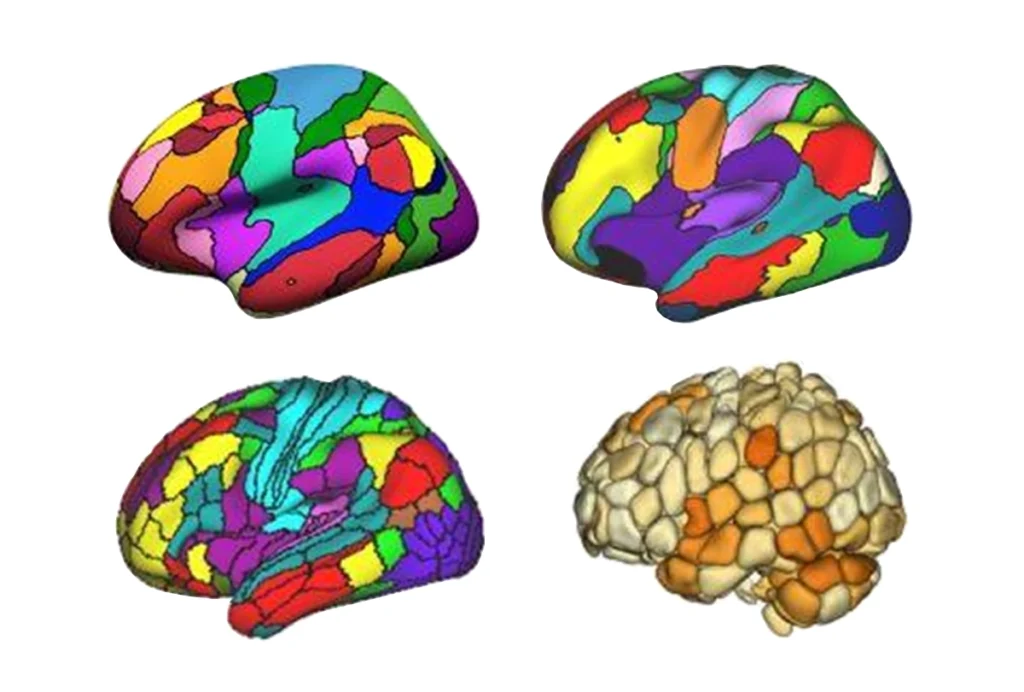
New ‘decoder’ tool translates functional neuroimaging terms across labs
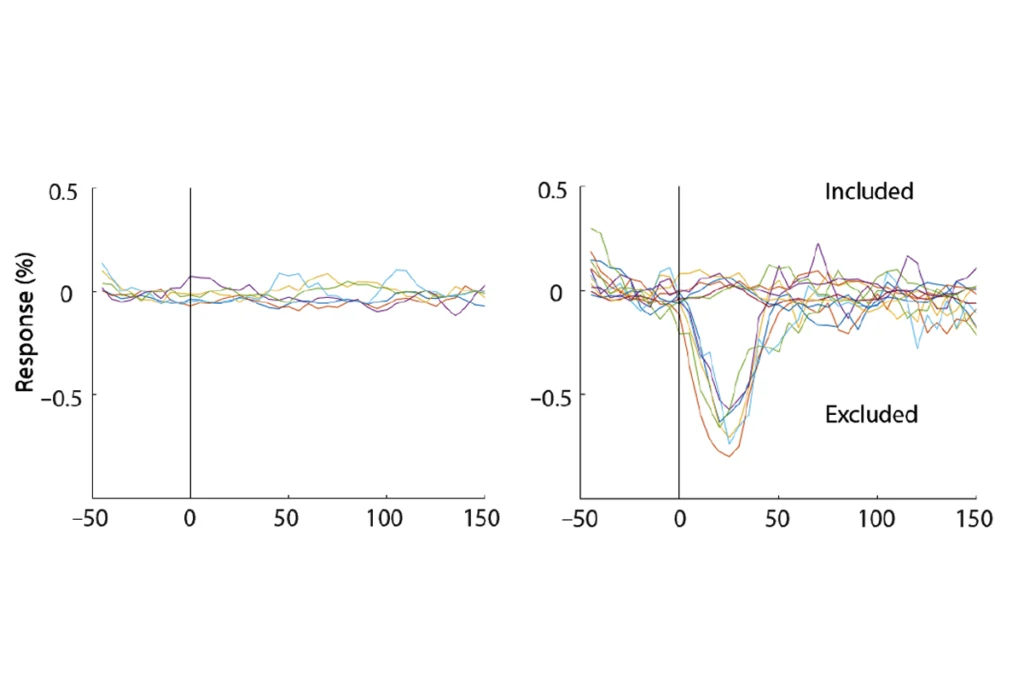
Two studies fail to replicate ‘holy grail’ DIANA fMRI method for detecting neural activity
Explore more from The Transmitter
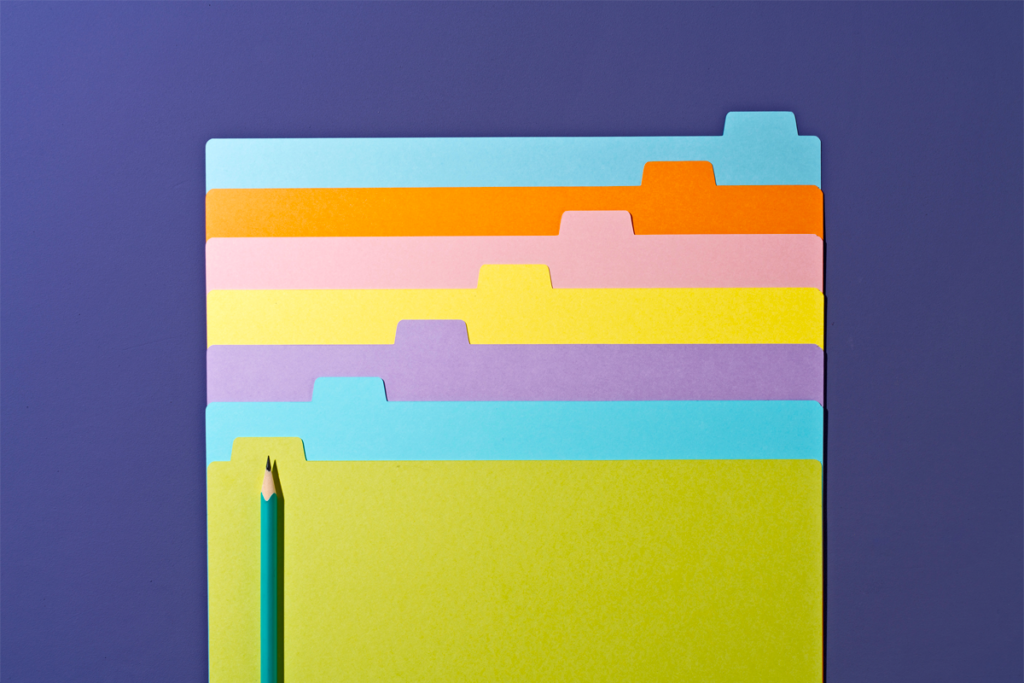
Protocol-sharing site aims to ease administrative burden of animal research
David Robbe challenges conventional notions of time and memory
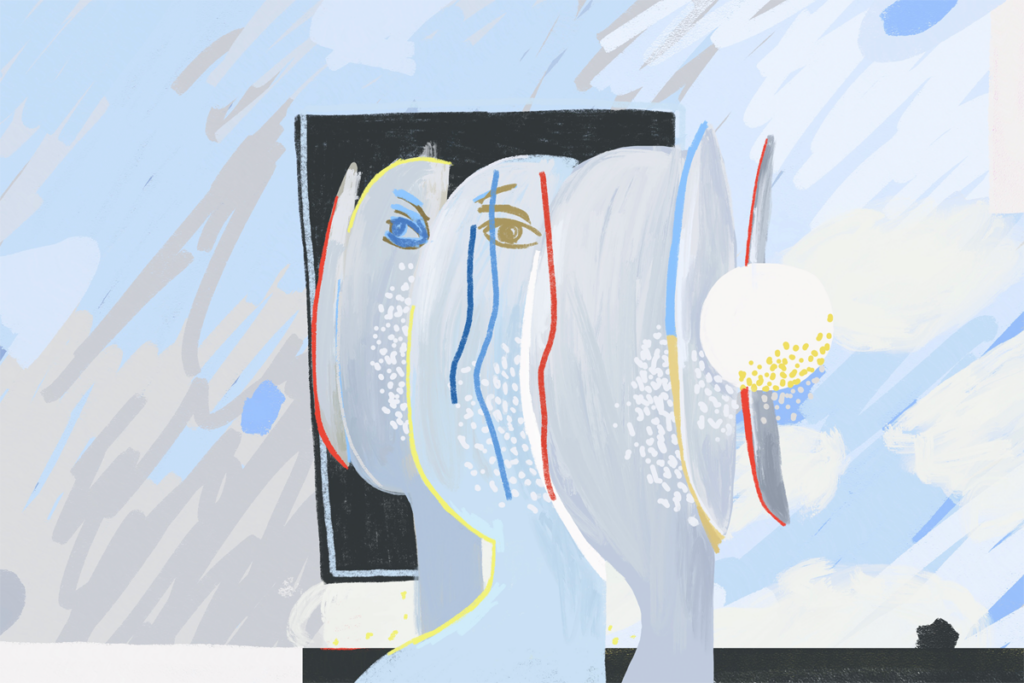