Nonsense correlations and how to avoid them
This statistical error is common in systems neuroscience. Fortunately, straightforward methods can help you prevent it.
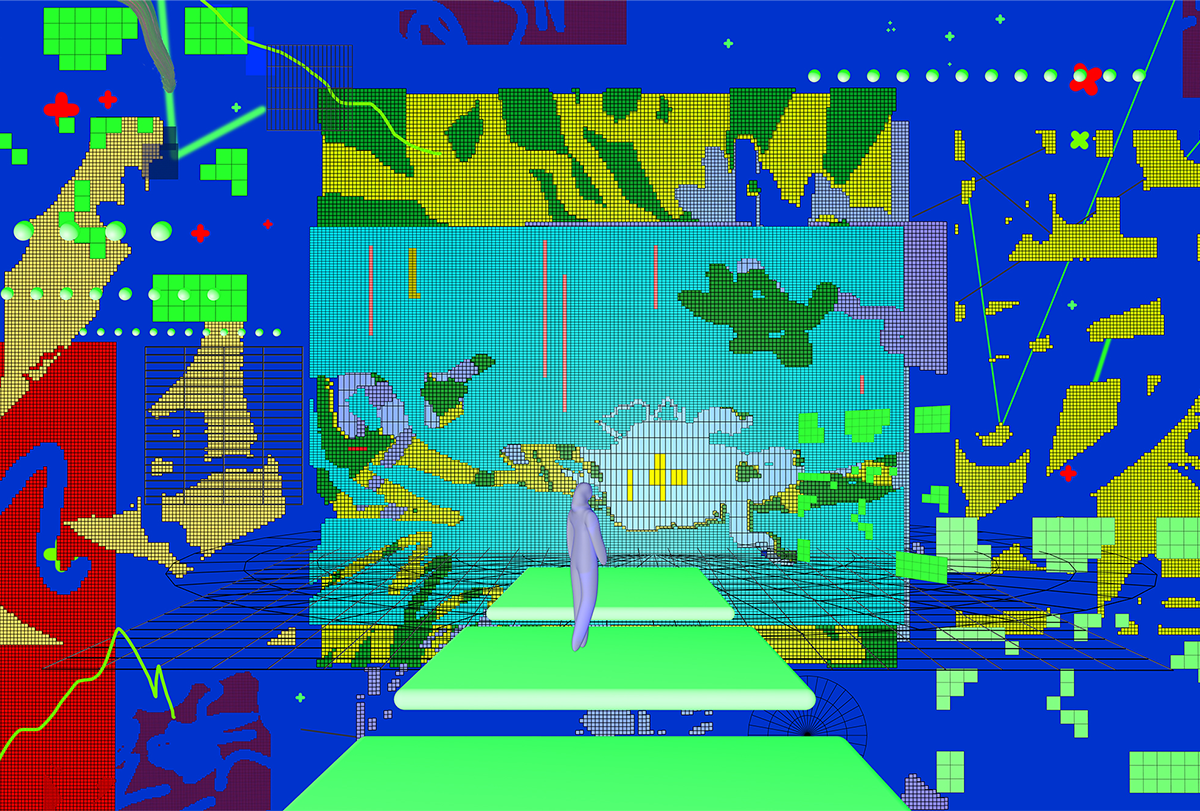
In 2021, neuroscientist Guido Meijer reported a seemingly bizarre finding—70 percent of neurons in the mouse brain correlated with fluctuations in the price of cryptocurrency. Meijer wasn’t trying to suggest that mice follow the crypto market. Rather, the study was a satirical demonstration of a common type of statistical error called nonsense correlations, which can occur between two unrelated variables that both evolve slowly over time.
Nonsense correlations are an altogether too common issue in systems neuroscience that can crop up whenever researchers incorrectly assume that samples in a study are statistically independent. Simultaneously recorded neurons are not statistically independent, nor are successive time points or trials in a recording. Human and animal behavior often changes slowly across an experimental session. Neuronal firing rates show slow drifts with time, leading to correlations between successive time points. Measurement errors, such as those due to brain movement, also show slow correlated drifts. Assuming samples are independent when these slow drifts are present can lead to the detection of nonsense correlations between unrelated variables—like neuronal activity and the price of cryptocurrency.
Consider a behavioral experiment in which the state of the world changes a few times during the experiment. In a decision-making task my group uses as part of the International Brain Lab, for example, sensory stimuli appear on the left or right of a head-fixed mouse according to a given probability. That probability changes every 100 trials or so, creating a block-like structure that is correlated across trials. Neuronal firing rates will also be correlated across trials because of technical phenomena, such as electrode drift, which are unrelated to the blocks. So if a neuron recorded for the first 100 trials of the experiment is then lost to drift, and if the first 100 trials happened to be a left block, traditional statistics would indicate a strong correlation between the block and neuronal firing, even though the neuron has no genuine relationship with the block.
Fortunately, many statistical approaches work even in the presence of non-independent trials. How can a scientist make sure to use valid statistics? The first and most important step is to actually care. If you are not worried about using invalid statistical methods, you are probably using them.
T
he best approach is to use randomization tests, which are almost foolproof and require only randomized experiments. In a randomized experiment, the experimenter controls one set of variables, X, according to a known probabilistic rule (the vector X stands for many variables together, such as the block identity of each trial) and measures a second set of variables, Y, such as the neuronal firing rates on each trial. The experimenter defines a test statistic, T(X,Y) and compares its actual value with a “null distribution” obtained by repeatedly and randomly redrawing X’ using the same probabilistic rules that originally generated X and recomputing the test statistic T(X’,Y). Under the null hypothesis that X has no effect on Y, the percentile of the test statistic within the null distribution gives a valid p-value. Randomization tests are easy to apply, difficult to get wrong and make no assumptions. They are thus one of the strongest available defenses against invalid statistics.Randomization tests can work even when successive trials and simultaneously recorded neurons are correlated. To use a randomization test in this situation, the experimenter must first generate a null distribution by re-randomizing the entire experimental session, producing a “pseudosession.” In the previous example, the experimenter would repeatedly generate pseudosession block sequences X’ using the same probabilistic rules as in the original experiment. Under the null hypothesis that the neuronal activity sequence is independent of the block sequence, firing is just as correlated with the block sequence in any of these pseudosessions as in the original. We can use any test statistic T(X,Y): One example would be the accuracy with which we can predict the block from neuronal population activity on each trial. The percentile of the actual value T(X,Y) within the null distribution of the T(X’,Y) again gives a valid p-value for the null hypothesis that X has no effect on Y, even though both the block and neuronal activity show slow drifts.
The pseudosession approach can be used only for randomly generated variables. Other methods exist for variables that were not randomly generated, such as an model animal’s spontaneous behavior and neuronal activity. For example, the “session permutation test” compares the correlation of brain activity with simultaneous behavior against a null ensemble in which entire behavior traces have been shuffled between recording sessions. Further tests are available for other cases, some of which make assumptions that the independence of each trial is weaker than full independence.
Statistical analysis is often seen as an afterthought, and caring about valid statistics as pedantic nitpicking. But a scientist who does not use valid statistics can prove anything. If a study uses invalid statistics to support a splashy conclusion, there is particular reason to be skeptical. Second, don’t use methods you don’t understand. Randomization tests are simple; some other methods, such as the bootstrap, have a seductively simple appearance but fail in some circumstances. If you don’t know when a method works and when it fails, stick to something you really understand. Finally, design experiments that make statistical analysis easy. Randomize everything you can: every stimulus, every inter-trial interval, every reward. This extra care may enable you to validly test hypotheses you hadn’t even thought of when you started the experiment.
Recommended reading
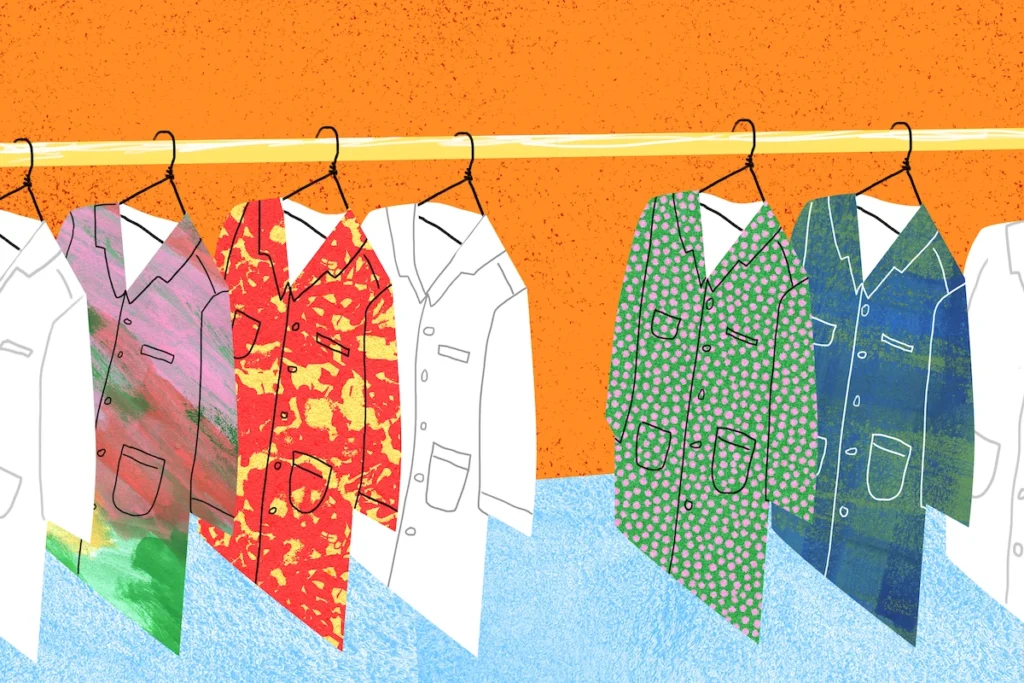
On fashion in neuroscience: In defense of freezing behavior
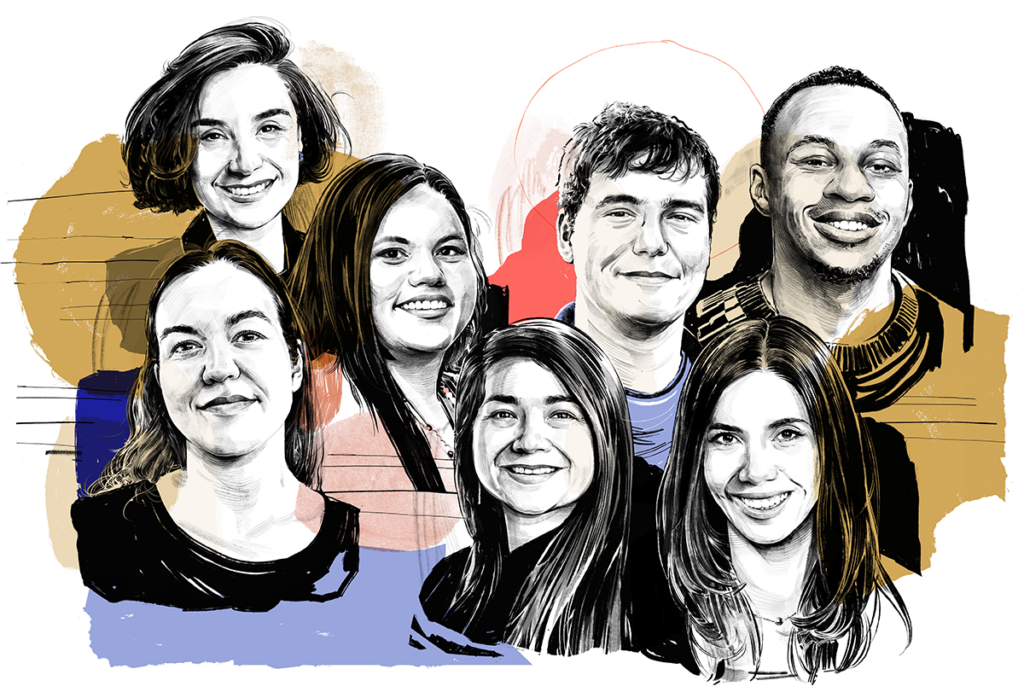
‘We still exist’: How four neuroscience advocacy groups are navigating federal DEI funding cuts
Explore more from The Transmitter
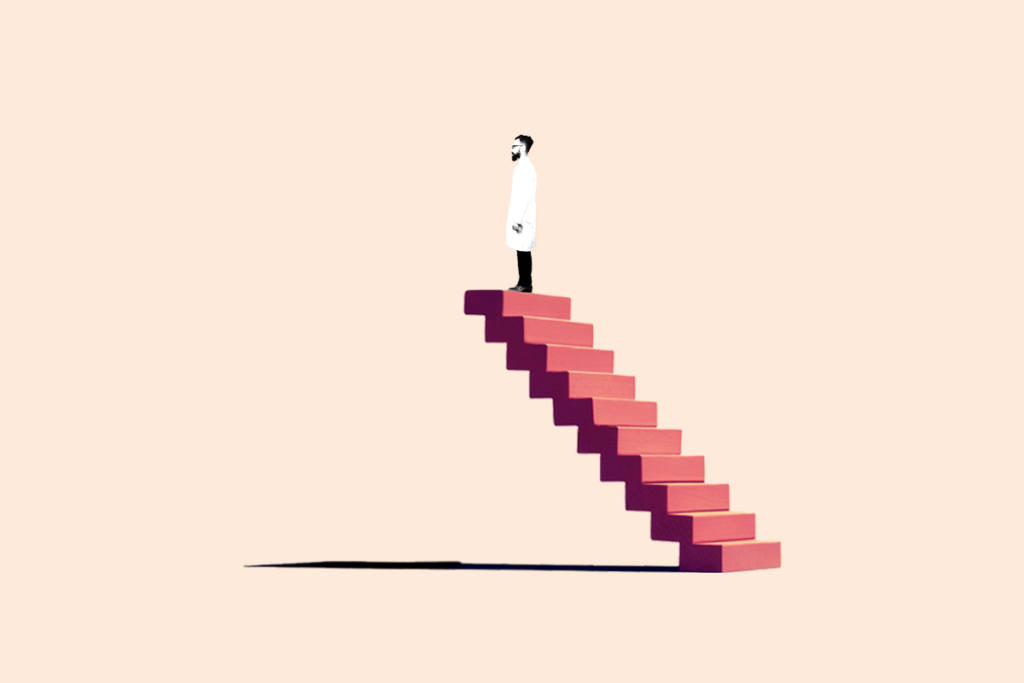